Галерея 3501547
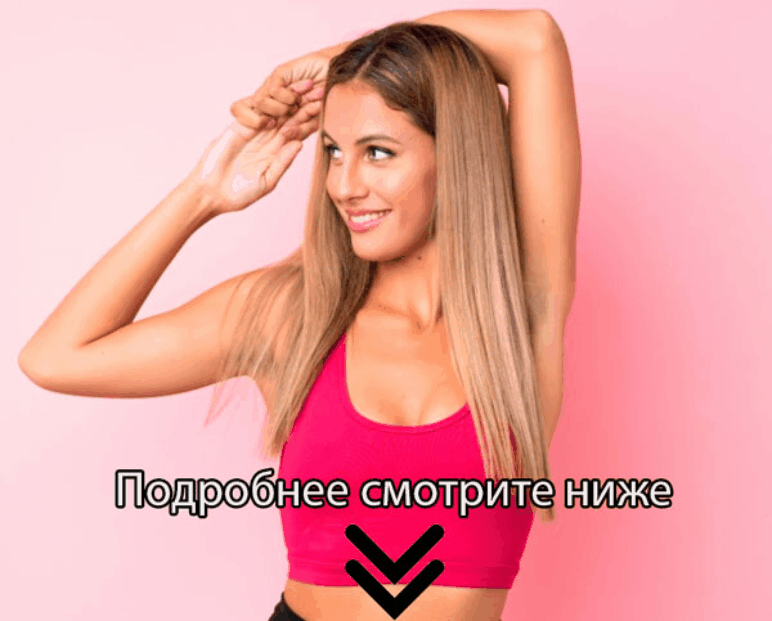
⚡ ПОДРОБНЕЕ ЖМИТЕ ЗДЕСЬ 👈🏻👈🏻👈🏻
Галерея 3501547
To navigate to Skip menu use control + q shortcut.
FLEX BOOKING. Kayakers Delight on the Salt Pond, Bethany Beach. 26SP
About Rooms & beds Amenities Reviews Map Host Policies Availability
Sussex County, Delaware, United States of America
2. Bethany Beach Nature Center 0.3 mi
4. Chief Little Owl Totem Pole 1 mi
Previous page 1 - 6 of 12 Next page
Wilgus Associates, Inc is a Premier Host · They consistently provide great experiences for their guests
Bookings at this property are non-refundable. Free cancellation deadlines are in the property's timezone. Learn more about cancellation policies.
If you have upcoming trips, you can manage or cancel your booking in your traveler account. View upcoming trip
You will be responsible for any damage to the rental property caused by you or your party during your stay.
Update your browser for a better experience.
Sign in
Register
Research on the Prediction of Employee Turnover Behavior and Its Interpretability
Published: 31 December 2021 Publication History
EITCE 2021: Proceedings of the 2021 5th International Conference on Electronic Information Technology and Computer Engineering
Qualifiers research-article Research Refereed limited
EITCE 2021
Paper Acceptance Rate 294 of 531 submissions, 55% Overall Acceptance Rate 508 of 972 submissions, 52%
Alao, D. and A.B. Adeyemo, ANALYZING EMPLOYEE ATTRITION USING DECISION TREE ALGORITHMS. Computing Information Systems Development Informatics & Allied Research Journal, 2014. 4(1). Google Scholar Sikaroudi, E., et al., A data mining approach to employee turnover prediction (case study: Arak automotive parts manufacturing). Journal of industrial and systems engineering, 2015. 8(4): p. 106--121. Google Scholar Sisodia, D.S., S. Vishwakarma, and A. Pujahari. Evaluation of machine learning models for employee churn prediction. in 2017 International Conference on Inventive Computing and Informatics (ICICI). 2017. IEEE. Google Scholar Cross Ref Zhang, H., et al. Analysis and prediction of employee turnover characteristics based on machine learning. in 2018 18th International Symposium on Communications and Information Technologies (ISCIT). 2018. IEEE. Google Scholar Cross Ref Jain, N., A. Tomar, and P.K. Jana, A novel scheme for employee churn problem using multi-attribute decision making approach and machine learning. Journal of Intelligent Information Systems, 2021. 56(2): p. 279--302. Google Scholar Chen, T., et al., Xgboost: extreme gradient boosting. R package version 0.4-2, 2015. 1(4): p. 1--4. Google Scholar Chen, T. and C. Guestrin. Xgboost: A scalable tree boosting system. in Proceedings of the 22nd acm sigkdd international conference on knowledge discovery and data mining. 2016. Google Scholar Digital Library Fernández, A., et al., SMOTE for learning from imbalanced data: progress and challenges, marking the 15-year anniversary. Journal of artificial intelligence research, 2018. 61: p. 863--905. Google Scholar Cross Ref Kong Xiangwei, Tang Xinze, and Wang Ziming, A review of research on the interpretability of artificial intelligence decision-making. System engineering theory and practice. 41(2): p. 524--536. Google Scholar Ribeiro, M.T., S. Singh, and C. Guestrin. "Why should i trust you?" Explaining the predictions of any classifier. in Proceedings of the 22nd ACM SIGKDD international conference on knowledge discovery and data mining. 2016. Google Scholar Digital Library Shapley, L.S., 17. A value for n-person games. 2016: Princeton University Press. Google Scholar Lundberg, S.M., et al., From local explanations to global understanding with explainable AI for trees. Nature machine intelligence, 2020. 2(1): p. 56--67. Google Scholar Lundberg, S.M. and S.-I. Lee. A unified approach to interpreting model predictions. in Proceedings of the 31st international conference on neural information processing systems. 2017. Google Scholar Digital Library Lundberg, S.M., G.G. Erion, and S.-I. Lee, Consistent individualized feature attribution for tree ensembles. arXiv preprint arXiv:1802.03888, 2018. Google Scholar Sundararajan, M. and A. Najmi. The many Shapley values for model explanation. in International Conference on Machine Learning. 2020. PMLR. Google Scholar Janzing, D., L. Minorics, and P. Blöbaum. Feature relevance quantification in explainable AI: A causal problem. in International Conference on Artificial Intelligence and Statistics. 2020. PMLR. Google Scholar Khalid, S., T. Khalil, and S. Nasreen. A survey of feature selection and feature extraction techniques in machine learning. in 2014 science and information conference. 2014. IEEE. Google Scholar Kira, K. and L.A. Rendell. The feature selection problem: Traditional methods and a new algorithm. in Aaai. 1992. Google Scholar
Browse All Return Change zoom level
Close modal New Citation Alert added!
Connect
Contact
Facebook
Twitter
Linkedin
Feedback
Bug Report
The ACM Digital Library is published by the Association for Computing Machinery. Copyright © 2023 ACM, Inc.
If you 'd like us to contact you regarding your feedback, please provide your contact details here.
Employee turnover is a key issue that companies must consider, and predicting employee turnover behavior can help companies reduce losses. However, the current Mechanism-Driven prediction method has some problems such as simplified assumptions and low accuracy. Scholars have begun to use it the Data-Driven method establishes an employee turnover behavior prediction model, but there is a lack of interpretability research on the model, which cannot effectively explain the prediction results, it will hinder the actual implementation of the method. This research establishes the RSGSBoost-SHAP prediction and interpretation framework on the basis of combing relevant literature. The framework can directly predict employee turnover behavior based on input characteristics, and explore the mechanism of the characteristics of the turnover behavior, providing management inspiration for enterprises. In the prediction results, AUC is 0.9224, and Recall is 0.8487, reaching the expected accuracy. The interpretation results elaborated on the mechanism of employee characteristics affecting their turnover behavior.
Check if you have access through your login credentials or your institution to get full access on this article.
Association for Computing Machinery
Request permissions about this article.
This publication has not been cited yet
View this article in digital edition.
https://dl.acm.org/doi/10.1145/3501409.3501547
This alert has been successfully added and will be sent to:
You will be notified whenever a record that you have chosen has been cited.
To manage your alert preferences, click on the button below.
We use cookies to ensure that we give you the best experience on our website.
Save time and increase productivity
Buy from all your part sellers in one place
Save time and increase productivity
Buy from all your part sellers in one place
Buy from all your part sellers in one place
View order history from all your accounts
Save Orders from your favorite sellers
User management on the customizable dashboard
Available through updated Shop Management Systems
Copyright © 1995-2023 WHI Solutions Inc. All Rights Reserved. OEM Copyright , User Agreement , Privacy and Cookies .
Два худых парня решают расчехлить девушку и дают ей опробовать два члена в себе
Галерея 2855461
Красивая баба ебется ради трудоустройства