Yolo Selfies Website
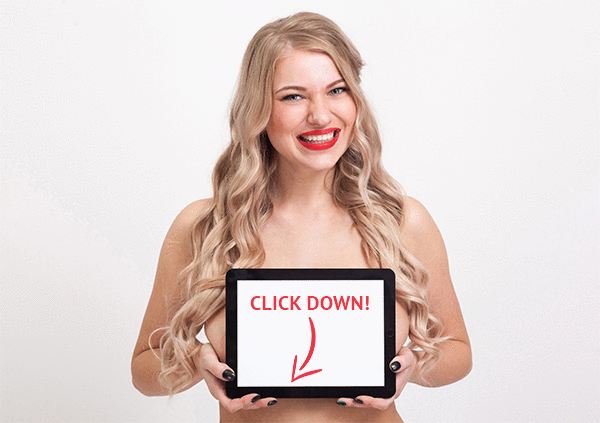
β‘ ALL INFORMATION CLICK HERE ππ»ππ»ππ»
Yolo Selfies Website
Want to join? Log in or sign up in seconds. | English
Get an ad-free experience with special benefits, and directly support Reddit.
use the following search parameters to narrow your results:
e.g. subreddit:aww site:imgur.com dog
Where a community about your favorite things is waiting for you.
and subscribe to one of thousands of communities.
submitted 6 years ago by lealover to r/legendarylea
Use of this site constitutes acceptance of our User Agreement and Privacy Policy . Β© 2022 reddit inc. All rights reserved.
REDDIT and the ALIEN Logo are registered trademarks of reddit inc.
ΟΒ Rendered by PID 38 on reddit-service-r2-loggedout-569764b694-5kgxb at 2022-07-25 10:50:35.703882+00:00 running fdb1904 country code: RU.
Shortcuts to other sites to search off DuckDuckGo Learn More
yoloselfie (@ yoloselfie ) / Twitter yoloselfie @ yoloselfie http://www. yoloselfie .com Joined September 2013 14 Following 580 Followers Tweets Tweets & replies Media Likes yoloselfie @ yoloselfie Β· Jan 25, 2014 this is one hell of a damn fine nude selfie . color & lighting matches YOLO logo perfectly yoloselfie .com/i/2314 # yoloselfie #nakedselfie 3 1 10
April 7, 2017, 3:20 PM YOLO ! This girl took secret selfies with a horse in her parents' bathroom Sometimes kids can get into some pretty strange stuff at home, and parents have no idea what's going...
Reddit gives you the best of the internet in one place. Get a constantly updating feed of breaking news, fun stories, pics, memes, and videos just for you. Passionate about something niche? Reddit has thousands of vibrant communities with people that share your interests. Alternatively, find out what's trending across all of Reddit on r/popular.
YOLO is a collective of workaholic filmmakers who will do all it takes to make films. Because they are running out of time. Statistically, there are 7,372,859,848 people all around the world, each of them has a story waiting to be told. Assuming they are all equally compelling. Assuming our filmmakers have 70 years to live, then they have ...
YOLO : Real-Time Object Detection You only look once ( YOLO ) is a state-of-the-art, real-time object detection system. On a Pascal Titan X it processes images at 30 FPS and has a mAP of 57.9% on COCO test-dev. Video unavailable Watch on YouTube Watch on Comparison to Other Detectors YOLOv3 is extremely fast and accurate.
A collection of the hottest selfies that will make you happy. Hotness; Video; Lifestyle; More; Tyler. Hotness Selfies . 2 min read 113343. The hottest selfies on the Web (36 Photos) Report Issue. Please complete the required fields. Report Issue, thanks for your time in advance! Email. Reason (required)
PSA: Come to a complete stop before taking a Car Selfie (36 Photos) by: Brady. Apr 12, 2022 04/12/22. 44 Liked! 0 Disliked 0 hotness Magic mirror on the wall, S*xy Chivers are the fairest of all (100 Photos) by: Brady. Apr 8, 2022 04/08/22. 24 Liked! 0 Disliked 0 hotness
Madonna's Topless Selfie Reignites Claims She Drinks Adrenochrome from Kids' Blood 22 Inappropriate Selfies By Shameless People 22 People Taking Awesome Selfies 21 People Giving up Their Dignity in the Relationship 32 Passive Aggressive Parking Notes 34 Cool Things People Seldom See ...
View 5 883 NSFW pictures and enjoy GoneWildScrubs with the endless random gallery on Scrolller.com. Go on to discover millions of awesome videos and pictures in thousands of other categories.
May 14, 2021 Borland and her L.A. dealer, Nino Mier, who will open an exhibition featuring 14 of the artist's new photos at his latest space in West Hollywood on Saturday, May 15, consider this recent body ...
Help your friends and family join the Duck Side!
Stay protected and informed with our privacy newsletters.
Searches related to Yolo Selfies Website
Switch to DuckDuckGo and take back your privacy!
Try our homepage that never shows these messages:
Learn how you can free yourself from Google for good.
You're in control. Customize the look-and-feel of DuckDuckGo.
Learn how we're dedicated to keeping you safe online.
You only look once (YOLO) is a state-of-the-art, real-time object detection system. On a Pascal Titan X it processes images at 30 FPS and has a mAP of 57.9% on COCO test-dev.
YOLOv3 is extremely fast and accurate. In mAP measured at .5 IOU YOLOv3 is on par with Focal Loss but about 4x faster. Moreover, you can easily tradeoff between speed and accuracy simply by changing the size of the model, no retraining required!
Prior detection systems repurpose classifiers or localizers to perform detection. They apply the model to an image at multiple locations and scales. High scoring regions of the image are considered detections.
We use a totally different approach. We apply a single neural network to the full image. This network divides the image into regions and predicts bounding boxes and probabilities for each region. These bounding boxes are weighted by the predicted probabilities.
Our model has several advantages over classifier-based systems. It looks at the whole image at test time so its predictions are informed by global context in the image. It also makes predictions with a single network evaluation unlike systems like R-CNN which require thousands for a single image. This makes it extremely fast, more than 1000x faster than R-CNN and 100x faster than Fast R-CNN . See our paper for more details on the full system.
YOLOv3 uses a few tricks to improve training and increase performance, including: multi-scale predictions, a better backbone classifier, and more. The full details are in our paper !
This post will guide you through detecting objects with the YOLO system using a pre-trained model. If you don't already have Darknet installed, you should do that first . Or instead of reading all that just run:
You already have the config file for YOLO in the cfg/ subdirectory. You will have to download the pre-trained weight file here (237 MB) . Or just run this:
You will see some output like this:
Darknet prints out the objects it detected, its confidence, and how long it took to find them. We didn't compile Darknet with OpenCV so it can't display the detections directly. Instead, it saves them in predictions.png . You can open it to see the detected objects. Since we are using Darknet on the CPU it takes around 6-12 seconds per image. If we use the GPU version it would be much faster.
I've included some example images to try in case you need inspiration. Try data/eagle.jpg , data/dog.jpg , data/person.jpg , or data/horses.jpg !
The detect command is shorthand for a more general version of the command. It is equivalent to the command:
You don't need to know this if all you want to do is run detection on one image but it's useful to know if you want to do other things like run on a webcam (which you will see later on ).
Instead of supplying an image on the command line, you can leave it blank to try multiple images in a row. Instead you will see a prompt when the config and weights are done loading:
Enter an image path like data/horses.jpg to have it predict boxes for that image.
Once it is done it will prompt you for more paths to try different images. Use Ctrl-C to exit the program once you are done.
By default, YOLO only displays objects detected with a confidence of .25 or higher. You can change this by passing the -thresh flag to the yolo command. For example, to display all detection you can set the threshold to 0:
So that's obviously not super useful but you can set it to different values to control what gets thresholded by the model.
We have a very small model as well for constrained environments, yolov3-tiny . To use this model, first download the weights:
Then run the detector with the tiny config file and weights:
Running YOLO on test data isn't very interesting if you can't see the result. Instead of running it on a bunch of images let's run it on the input from a webcam!
To run this demo you will need to compile Darknet with CUDA and OpenCV . Then run the command:
YOLO will display the current FPS and predicted classes as well as the image with bounding boxes drawn on top of it.
You will need a webcam connected to the computer that OpenCV can connect to or it won't work. If you have multiple webcams connected and want to select which one to use you can pass the flag -c to pick (OpenCV uses webcam 0 by default).
You can also run it on a video file if OpenCV can read the video:
That's how we made the YouTube video above.
You can train YOLO from scratch if you want to play with different training regimes, hyper-parameters, or datasets. Here's how to get it working on the Pascal VOC dataset.
To train YOLO you will need all of the VOC data from 2007 to 2012. You can find links to the data here . To get all the data, make a directory to store it all and from that directory run:
There will now be a VOCdevkit/ subdirectory with all the VOC training data in it.
Now we need to generate the label files that Darknet uses. Darknet wants a .txt file for each image with a line for each ground truth object in the image that looks like:
Where x , y , width , and height are relative to the image's width and height. To generate these file we will run the voc_label.py script in Darknet's scripts/ directory. Let's just download it again because we are lazy.
After a few minutes, this script will generate all of the requisite files. Mostly it generates a lot of label files in VOCdevkit/VOC2007/labels/ and VOCdevkit/VOC2012/labels/ . In your directory you should see:
The text files like 2007_train.txt list the image files for that year and image set. Darknet needs one text file with all of the images you want to train on. In this example, let's train with everything except the 2007 test set so that we can test our model. Run:
Now we have all the 2007 trainval and the 2012 trainval set in one big list. That's all we have to do for data setup!
Now go to your Darknet directory. We have to change the cfg/voc.data config file to point to your data:
You should replace with the directory where you put the VOC data.
For training we use convolutional weights that are pre-trained on Imagenet. We use weights from the darknet53 model. You can just download the weights for the convolutional layers here (76 MB) .
You can train YOLO from scratch if you want to play with different training regimes, hyper-parameters, or datasets. Here's how to get it working on the COCO dataset .
To train YOLO you will need all of the COCO data and labels. The script scripts/get_coco_dataset.sh will do this for you. Figure out where you want to put the COCO data and download it, for example:
Now you should have all the data and the labels generated for Darknet.
Now go to your Darknet directory. We have to change the cfg/coco.data config file to point to your data:
You should replace with the directory where you put the COCO data.
You should also modify your model cfg for training instead of testing. cfg/yolo.cfg should look like this:
If you want to use multiple gpus run:
If you want to stop and restart training from a checkpoint:
If you are using YOLO version 2 you can still find the site here: https://pjreddie.com/darknet/yolov2/
If you use YOLOv3 in your work please cite our paper!
The hottest selfies on the Web (36 Photos)
Please complete the required fields.
Report Issue, thanks for your time in advance!
Reason (required) This post contains broken image(s)
This post contains a broken video
This post contains broken links
Post has incorrect information
Post has spam or spam comments
Other
Β© 2006 - 2021 MEMT-NETWORK. All rights reserved.
This website uses cookies to improve your experience. We'll assume you're ok with this, but you can opt-out if you wish. Cookie settings ACCEPT
This website uses cookies to improve your experience while you navigate through the website. Out of these cookies, the cookies that are categorized as necessary are stored on your browser as they are essential for the working of basic functionalities ...
Necessary cookies are absolutely essential for the website to function properly. This category only includes cookies that ensures basic functionalities and security features of the website. These cookies do not store any personal information.
Any cookies that may not be particularly necessary for the website to function and is used specifically to collect user personal data via analytics, ads, other embedded contents are termed as non-necessary cookies. It is mandatory to procure user consent prior to running these cookies on your website.
You must be logged in to post a comment.
Hq Milf Pics
Twistys Porn Star
Pornleech