Solo V2
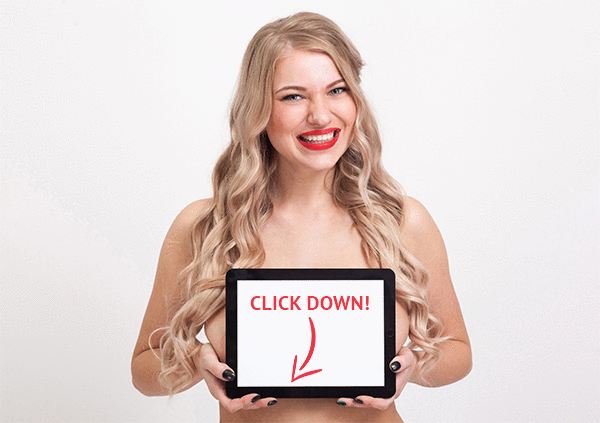
🛑 ALL INFORMATION CLICK HERE 👈🏻👈🏻👈🏻
Solo V2
The Solo V2 brings improved NFC, water resistant, updatable firmware, and a reversible USB connector.
Google Pixel 7 and Pixel 7 Pro: Everything announced and the best deals
Here's everything announced at the Google Pixel Event
Pixel 7 and Pixel 7 Pro hands-on: The start of Google's walled garden
we equip you to harness the power of disruptive innovation, at work and at home.
© 2022 ZDNET, A Red Ventures company. All rights reserved.
Privacy Policy |
Cookie Settings |
Advertise |
Terms of Use
Two-factor authentication security keys are now all the rage. If you care about security, then you have at least a couple of them on keyrings with you at any one time. The Solo V2, currently on offer via Kickstarter , brings some new features to these must-have items.
On the face of it, they look like any other two-factor authentication security keys on the market but look closer, and there are some interesting features.
First up, they are robust. The guts are encapsulated in epoxy resin, making them durable and hard to tamper with.
Then there's the reversible connector, a really useful feature for the USB-A version since you can orient it in a way that the LED shows up.
There are also three capacitive touch pads, again making the Solo V2 easy to use no matter what the orientation.
There's also enhanced NFC; you get more reliable wireless authentication.
Then there's updatable firmware that can keep the keys fully updated. As far as I'm aware, this feature is unique to SoloKeys. The firmware updates will be signed by SoloKeys, and the user will need to carry this out (so no stealthy background updates). According to Solokeys, this is more secure and much cheaper than physically replacing all your security keys.
The Solo V2 keys also come with colorful silicon sleeves.
The Solo V2 support FIDO2 and will work seamlessly with services such as Google, Facebook, Twitter, Dropbox, Github, and many more.
Keys are expected to start shipping June 2021, and prices start at $34 for a single key.
Home Blog
Solo V2 – Your Safety Net Against Phishing
When you login first time using a Social Login button, we collect your account public profile information shared by Social Login provider, based on your privacy settings. We also get your email address to automatically create an account for you in our website. Once your account is created, you'll be logged-in to this account.
new follow-up comments
new replies to my comments
The comment form collects your name, email and content to allow us keep track of the comments placed on the website. Please read and accept our website Terms and Privacy Policy to post a comment.
When you login first time using a Social Login button, we collect your account public profile information shared by Social Login provider, based on your privacy settings. We also get your email address to automatically create an account for you in our website. Once your account is created, you'll be logged-in to this account.
Archives Archives
Select Month
October 2022
September 2022
August 2022
July 2022
June 2022
May 2022
April 2022
March 2022
February 2022
January 2022
December 2021
November 2021
October 2021
September 2021
August 2021
July 2021
June 2021
May 2021
April 2021
March 2021
February 2021
January 2021
December 2020
November 2020
October 2020
September 2020
August 2020
July 2020
June 2020
May 2020
April 2020
March 2020
February 2020
January 2020
December 2019
November 2019
October 2019
September 2019
August 2019
July 2019
June 2019
May 2019
April 2019
March 2019
February 2019
January 2019
December 2018
November 2018
October 2018
September 2018
August 2018
July 2018
June 2018
May 2018
April 2018
March 2018
February 2018
January 2018
December 2017
November 2017
October 2017
September 2017
August 2017
July 2017
June 2017
May 2017
April 2017
March 2017
February 2017
January 2017
December 2016
November 2016
October 2016
September 2016
August 2016
July 2016
June 2016
May 2016
April 2016
March 2016
February 2016
January 2016
December 2015
November 2015
October 2015
September 2015
August 2015
July 2015
Check your inbox now to confirm your subscription.
A campaign was launched on Kickstarter for an open security key to protect your Gmail, Twitter, GitHub & more called Solo V2 . Solo V2 is from the team that brought the first open-source FIDO2 security key. Modern security solutions are often expensive, making them unavailable to many. However, you can easily protect yourself from a compromised account thanks to the Solo V2 security key. Solo V2 helps reduces the risk of security breaches, as over 80% of all breaches are caused by passwords compromised through phishing email attacks. Talking about Solo V2, the company says :
“Our goal with Solo V2 is to harden physical security of the device itself while providing all the protections that come with 2FA or passwordless login.”
The Solo V2 is a complete redesign of the original FIDO2 security key. They designed the hardware to be more physically robust, the NFC performance is increased, and uses a brand new microcontroller by NXP with enhanced security features, and the firmware has been rewritten in Rust to be more modular/reusable by the community and to reduce the attack surface. Solo V2 is easy to use. No need to install any software. You can use your V2 immediately. You just have to follow the following steps:
Solo V2 is offering the first-ever reversible USB-A connector. This connector enables you to insert the key into a USB Port, and also helps you to decide what side you want to face up. This fixes the problem of having a key be oriented with the LED light not facing the operator.
The Solo V2 features 3 touch buttons that eliminate the stress of depressing buttons, like what’s applicable in the first version. This allows for easier activation. Solo V2 features an innovative antenna design, which enhances its performance, improving the range and field strength. Solo V2’s design strengthens the weak points that are usually present at the base of the USB connector and the PCB. Solo V2 supports firmware upgrades. This enables users and enterprise customers to commit to the company’s authenticator knowing that they will have access and benefit from the improvements the company makes to the firmware down the road. Thanks to Solo supporting the new FIDO2 standard, you get the strongest, most secure login with protection against phishing, account takeover, and other online attacks.
About software updates, the company says:
“We believe that software must be up-to-date to be secure. Like laptops and mobile phones, security keys are adding more and more functionality, and therefore won’t be exempt from bugs and vulnerabilities. Since our very first model, all of our keys support signed firmware updates. Each device can only update to the latest firmware that has been securely signed by SoloKeys.”
The company really paid attention to the durability of the v2 series. They made it one of the most robust keys on the market. Its Components are assembled on a module, inserted in a cavity, and sealed with a layer of epoxy. This makes the keys waterproof and tamper-resistant. Its hard, robust exterior shell house inputs like USB, buttons, NFC. It has an internal PCB that contains all the sensitive circuits and a secure microcontroller. Its internal PCB is housed inside the external PCB & sealed shut using transparent epoxy.
Preventing breaches can save corporations millions of dollars. Protection from phishing can save you from major problems and personal security concerns.
For more information about the Solo V2, visit the campaign page on Kickstarter .
I am passionate about technology especially consumer electronics and gadgets and I love to talk and write about them. At my spare time I play video games, watch movies and I love biking.
After subscribing you can choose how often you will receive our updates: https://wordpress.com/following/manage
© Electronics-lab.com – 2022, WORK IS LICENCED UNDER CC BY SA 4.0
# cate branch
for i , cate_layer in enumerate ( self . cate_convs ):
if i == self . cate_down_pos :
seg_num_grid = self . seg_num_grids [ idx ]
cate_feat = F . interpolate ( cate_feat , size = seg_num_grid , mode = 'bilinear' )
# 将FPN最高层的特征从H*W*feat_channel 对齐到 S*S*feat_channel
cate_feat = cate_layer ( cate_feat ) # 7个卷积
cate_pred = self . solo_cate ( cate_feat ) # 从 S*S*feat_channel 到 S*S*num_classes
# ins branch
# concat coord
x_range = torch.linspace(-1, 1, ins_feat.shape[-1], device=ins_feat.device)
y_range = torch.linspace(-1, 1, ins_feat.shape[-2], device=ins_feat.device)
y, x = torch.meshgrid(y_range, x_range)
y = y.expand([ins_feat.shape[0], 1, -1, -1])
x = x.expand([ins_feat.shape[0], 1, -1, -1])
coord_feat = torch.cat([x, y], 1)
ins_feat = torch.cat([ins_feat, coord_feat], 1) # CoordConv
for i, ins_layer in enumerate(self.ins_convs): # 7个3*3 Conv 提取特征
ins_feat = ins_layer(ins_feat) # H*W*feat_channel
ins_feat = F.interpolate(ins_feat, scale_factor=2, mode='bilinear') # 上采样到2H*2W*feat_channel
ins_pred = self.solo_ins_list[idx](ins_feat)
# 从2H*2W*feat_channel到2H*2W*(S^2) S^2的复杂度,这里也是Decoupled 和 Solo v2的优化之处
对应关系如下:1×1×E的卷积核,则D=E,3×3×E的卷积核,则D=9E,??没看懂
1x1的卷积核有一个权重需要学习,3x3的卷积核就对应了9个权重需要学习。
unified mask feature representation 和 separate maskfeature representation的定义写反了,很棒的文章
这个就像YOLO V1啊,一个格子预测一个物体,不过这里用了FPN做到了多尺度,但是依然和YOLO V1一样的问题,格子数量无法覆盖全部小物体。所以这里后续肯定还是要继续改进升级。
大家看,小目标的精度远低于大目标的,我觉得既然使用了残差结构,假如小目标在前级网络中就已经被识别出来,那么在残差块的作用下也会基本无损传到后级网络,但实际是小目标的精度很低。而大目标的精度很高,网络超参数对大目标的识别远高于小目标,说明是网络本身的问题导致大小目标精度不一致,所以得改进基础网络还提高小目标的召回率和精度,同时由于网络是loss函数的平均优化,小目标的精度提高大概率也会提高大目标的精度。
最后两张图说明的是精度要比makrcnn更好,那么当maskrcnn加上pointrend呢
FPN输出的不同层级特征图,不是都要接类别分支和掩膜分支吗? 所以两个分支的输入,应该是FPN不同层级的特征图H1*W1*256、H2*W2*256、H3*W3*256、H4*W5*256、H5*W5*256吧
我也认为这个题主没能说清楚这件事。你说的这个不同层级的特征图确实用在了动态卷积核分支上,应该是每一个层级都预测了相应的卷积核。但是,对于掩膜特征分支(Mask Feature Branch),它的输入特征是被整合了的特征(原论文里附件里图5就是这个意思,将不同层级的特征图整合为1/4大小,这样会带来效果的提升),随后再经过一些上采样等操作,输出的大小就会变成H×W×E。
未注册手机验证后自动登录,注册即代表同意 《知乎协议》 《隐私保护指引》
论文名称:《SOLO v2: Dynamic, Faster and Stronger》
SOLO v2遵循了SOLO的优雅、简单的设计,并且针对mask的检测效果和运行效率做了两个改进:(1)mask learning:能够更好地学习到mask(2)mask NMS:提出了matrix nms,大大减少了前向推理的时间。 最终,SOLOv2相比SOLOv1,AP提升1.9%的同时速度快了33%,light-weight 版本的SOLVv2能够在COCO上达到31.3FPS,37.1%AP。除此之外,SOLO v2还在目标检测和全景分割任务中表现上佳,证实了SOLO的思路有用在更多视觉任务的潜力。
SOLO的核心思想是:将分割问题转化为位置分类问题,从而做到不需要anchor,不需要normalization,不需要bounding box detections的实例分割。具体做法是:将图片划分成S×S的网格,如果物体的中心(质心)落在了某个网格中,那么该网格就有了两个任务:(1) Category Branch 负责预测该物体语义类别(2) Mask Branch 负责预测该物体的instance mask。这就对应了网络的两个分支。同时,SOLO在骨干网络后面使用了 FPN ,用来应对尺寸。FPN的每一层后都接上述两个并行的分支,进行类别和位置的预测,每个分支的网格数目也相应不同,小的实例对应更多的的网格。
Category Branch负责预测物体的语义类别,每个网格预测类别S×S×C,这部分跟YOLO是类似的。
重点看一下Mask Branch,每个正样本(有类别输出的网格)都会输出对应类别的instance mask,这里的通道channel和网格的对应关系是:第k个通道负责预测出第(i,j)个网格的instance mask,k = i*S+j。因此 输出维度 是 H×W×(S^2) 。这样的话就有了一一对应的语义类别和class-agnostic的instance mask。
SOLO的两个分支光看论文可能会比较迷糊,还好作者昨天开源了源代码,通过代码可以更加清晰地了解head部分的操作流程。总结如下:
(2)对于Category分支,首先将FPN最高层的特征从H×W×256对齐至S×S×256(256为特征通道数),然后经过一系列卷积(7个3×3卷积)提取特征,最后再经过一个3×3卷积将输出对齐到S×S×C(C为预测类别-1);
(3)对于Mask分支,首先对FPN最高层的特征做一个CoordConv,然后同样经过一系列卷积(7个3×3卷积)提取特征,此时维度为H×W×256,再做一步上采样至2H×2W×256,最后就是对齐到2H×2W×S^2的输出(Decoupled SOLO此处则为2H×2W×S)
仔细来看一下mask branch的最后一层的情况,如图中的(a)所示:这其实是一个1×1的卷积,以特征F(维度为H×W×E)做输入,输出M(维度为H×W×(S^2)),S^2的输出通道。这里卷积核设为G,G的维度就是1×1×E×(S^2)。这一层的操作其实是M=F∗G,作用是生成S×S个分类器,每个分类器都用来判断像素点是否属于这个位置的类别。
在SOLO v1中也提到了,包含S×S输出通道的M是冗余的,因为在大多数情况下物体在图片中的分布都是稀疏的,起到作用的通道(分类器数目)远远少于S×S。因此SOLO v1提出了 Decoupled SOLO ,如图中的(b)所示,通过将这些分类器分解成X和Y两个方向,将输出通道从S×S降到了 S+S 。
SOLO v2中提出了另一种思路,更加直接,如图中的(c)所示。由于M=F∗G中的M是冗余,F是固定的,那么就可以 直接去学习卷积核G 。这样做的话有三个好处:
此外,由于SOLO是一个完全box-free的算法,没有依赖box去生成mask,因此不需要做box的NMS,但取而代之的是需要做mask的NMS,但mask NMS是一个比较费时间的地方,复杂度比box NMS高不少,这也是SOLO需要优化的点。
SOLO v2中的mask branch 被分解为mask kernel branch和mask feature branch,分别对应卷积核的学习和特征的学习。两个分支的输出最终组合成整个mask branch的输出。
Mask kernel branch用来学习卷积核,即分类器的权重,有点类似STN和Dynamic Filter的思路。这里输入为H×W×E的特征F,其中E是输入特征的通道数;输出为卷积核S×S×D,其中S是划分的网格数目,D是卷积核的通道数。对应关系如下:1×1×E的卷积核,则D=E,3×3×E的卷积核,则D=9E,以此类推。注意到这里不需要激活函数。
Mask feature branch用来学习特征的表达,它的输入是backbone+FPN提取的不同层级的特征,输出是H×W×E的mask feature,用F表示。SOLO框架中一个重要的设计就是采用了FPN,应对不同的尺寸。如何将不同层级的特征融合得到mask feature是一个重要的部分。通常来说,可以有两种做法:
经过试验对比,作者选择了unified maskfeature representation。具体的实现则参考了Panotic FPN中的feature pyramid fusion,将FPN的P2到P5层分别依次通过【若干个3×3卷积 + group norm + ReLU + 2个双线性插值操作】,统一到原图的1/4尺寸,再做element-wise summation,最后接【1 × 1 convolution + group norm + ReLU】。
需要注意的是这里的 CoordConv 是在FPN的最高层(原图的1/32尺寸)使用,位置在3×3卷积和双线性插值之前。CoordConv的作用与SOLO v1中相同,提供更准确的位置敏感信息和实例特征信息。
有了前两个分支的输出,生成mask也就水到渠成。具体的做法是:对于在(i,j)的每个网格,先获得卷积核G,然后将其与特征F卷积得到实例Mask,这会生成【 最多】 S×S个mask。最后,使用Matrix NMS方法得到最终的实例分割结果。
在 前向预测 的过程中,图像先通过backbone和FPN,得到grid(i,j)位置的类别分值P(i,j)之后,先用较低的置信度阈值0.1筛选一遍结果后,再进行卷积提取mask 特征。mask特征与预测得到的卷积核进行卷积,再经过一个sigmoid操作,然后用0.5的阈值生成binary mask,最后做一步Matrix NMS,得到结果。
NMS是视觉任务的老熟人了,其缺点是繁琐、冗余的计算,此外,mask NMS 会比bbox NMS 造成更多的计算时间。对NMS的优化已经有了不少工作,通常分为两类,一是Soft NMS和Adaptive NMS,主要优化了精度;另一类是YOLACT中的Fast NMS,主要优化了速度。
SOLO v2中提出了Matrix NMS,思路源于Soft NMS,效果类似Fast NMS。使用并行的矩阵运算单次地实现NMS,Matrix NMS可以做到在不到1ms的时间里处理500张mask,并且比目前最快的Faster NMS要高0.4% AP。
Soft NMS的思路通过递归地根据IoU降低其他bbox的检测分数,分数较高的检测结果被降低分数后将被以很小的阈值消除。经过优化,这种顺序的过程与传统的Greedy NMS一样是顺序的,无法并行实现。
对于一个预测得到的mask,它的decay factor主要受两部分影响:
一是得分大于j点的所有i点对i的惩罚,这个很好实现,直接通过算一个包含IoU的衰减函数(线性衰减或高斯衰减) f\left(\mathrm{i} \mathrm{ou}_{i, j}\right) 就能得到;
二是整个mask被抑制的概率,这个概率就比较难直接算,但它通常与IoU有着正相关的关系。因此,Matrix NMS提出直接将最大重复的预测结果(对应最大的IoU值)的结果来近似这个抑制概率 f(i o u, i)=\min _{\forall s_{k}>s_{i}} f\left(\mathrm{i} \mathrm{ou}_{k, i}\right)
最后得到的decay factor就是 \operatorname{decay}_{j}=\min _{\forall s_{i}>s_{j}} \frac{f\left(\mathrm{i} \circ \mathrm{u}_{i, j}\right)}{f(\mathrm{i} 0 \mathrm{u}, i)} ,预测的分值就通过这个decay factor单步更新 s_{j}=s_{j} \cdot d e c a y_{j}
Matrix NMS实现可以一次性完成,不需要循环重复,具体的实现就不多说了,附上伪代码和对比实验结果。
实验结果就没啥好说的了,总的来说:更快更强,适应不同任务/不同数据集;分割效果来说,也要更加精细。
小白想问一下为什么用mask head之后可以减少参数呢?具体怎么从sxs classifier里面挑选valid ones啊
Self-Attention Attribution: Interpreting Information Interactions Inside Transformer(AAAI21)在之前大家对于Transformer的理解都是,Transformer的成功得益于强大Multi-head自注意机…
摘要 Graph neural networks (GNNs,图神经网络)广泛应用于获取图的表示学习,并在node classifification(节点分类)、link prediction(链接预测)任务上取得了当前最好的效果。当前的GNN…
Naughty But Nice Porn
Sister Taboo Hd Porno
Man Fuck Little Cow