Sharon Lee utilise la bonne technique
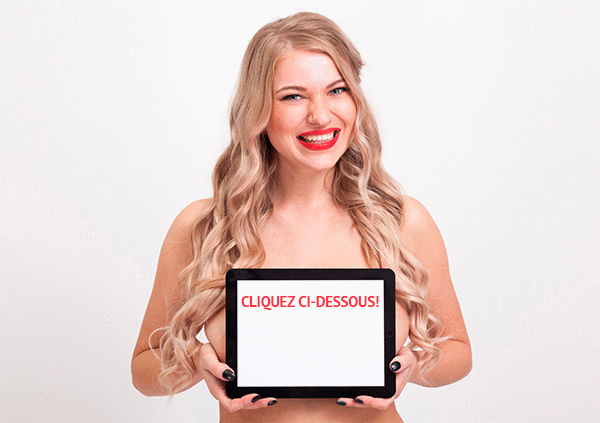
đ TOUTES LES INFORMATIONS CLIQUEZ ICI đđ»đđ»đđ»
Sharon Lee utilise la bonne technique
A password will be e-mailed to you.
Home Mode En photos, ces stars qui ont osé la mode des « seins libres »
La quatriĂšme gĂ©nĂ©ration de la sĂ©rie Galaxy Z vient changer notre rapport aux smartphones en repoussant les limites de la personnalisation, de lâexpĂ©rience Flex...
Ooredoo, sponsor officiel du Festival International de Carthage continue Ă propager la joie et dessiner le sourire sur les visages des enfants et leur...
C'est le moment de prendre soin de sa peau, de ses cheveux et de ses ongles aprĂšs les longues heures d'exposition au soleil
PassionnĂ© du football ? Vous voulez rencontrer des joueurs mondiaux et des cĂ©lĂ©britĂ©s ? Vous voulez assister aux matchs de lâĂ©quipe nationale lors de la coupe...
Vous ĂȘtes enfin en vacances et vous ĂȘtes amateurs de sensations fortes et dâadrĂ©naline. Vous avez toujours rĂȘvĂ© de rĂ©aliser sauts et acrobaties en...
Une cĂ©rĂ©monie sâest tenue hier jeudi 14 Juillet au siĂšge de Talan Tunisie en lâhonneur dâOns Jabeur aprĂšs sa qualification Ă la finale de...
La société Japanese Motors Company (JMC), concessionnaire agréé en Tunisie pour les véhicules particuliers du constructeur automobile japonais HONDA, annonce le retour de son...
La marque italienne premium de prĂȘt Ă porter pour hommes, BOGGI Milano a choisi le quartier le plus prisĂ© de Tunis pour y ouvrir...
ARTICLES LES PLUS POPULAIRES LA SEMAINE DERNIĂRE
La quatriĂšme gĂ©nĂ©ration de la sĂ©rie Galaxy Z vient changer notre rapport aux smartphones en repoussant les limites de la personnalisation, de lâexpĂ©rience Flex...
Ooredoo, sponsor officiel du Festival International de Carthage continue Ă propager la joie et dessiner le sourire sur les visages des enfants et leur...
C'est le moment de prendre soin de sa peau, de ses cheveux et de ses ongles aprĂšs les longues heures d'exposition au soleil
Un témoignage à lire jusqu'au bout #coronavirus
Follow us on Instagram @femmes_de_tunisie_officiel
© MENTIONS LĂGALES CGU© 2019 FEMMES DE TUNISIE BY NEXPRESSE
Les pro-« no bras » ne vous le diront jamais assez : le soutien-gorge nâest pas sans danger. Selon certaines recherches, les soutien-gorges seraient mĂȘme en partie responsables du cancer du sein. Sans soutif, la poitrine sâembellit et gagne en fermetĂ©. Les risques de chute des seins sont aussi minimisĂ©s. Bref, autant dâarguments, mĂȘme si non prouvĂ©s Ă 100%, qui prĂŽnent lâabandon de cette lingerie. Si de nombreuses femmes ont du mal Ă sâafficher les tĂątons Ă lâair, certaines stars ont dĂ©jĂ adoptĂ© la vie « publique » sans soutif.
Dashboard
Publications
Account settings
Log out
Journal List
CMAJ
v.193(44); 2021 Nov 8
PMC8584368
CMAJ. 2021 Nov 8; 193(44): E1708âE1715.
RĂ©seau hospitalier Unity Health de Toronto (Verma, Murray, Straus, Pou-Prom, Mamdani); Institut du savoir Li Ka Shing de lâHĂŽpital St. Michael (Verma, Straus, Pou-Prom, Mamdani); DĂ©partement de mĂ©decine (Verma, Shojania, Straus, Mamdani) et Institut des politiques, de la gestion et de lâĂ©valuation de la santĂ© (Verma, Mamdani) et DĂ©partement de statistique (Murray), UniversitĂ© de Toronto, Toronto, Ont.; UniversitĂ© de lâAlberta (Greiner); Institut dâintelligence machine de lâAlberta (Greiner), Edmonton, Alb.; Institut des algorithmes dâapprentissage de MontrĂ©al (Cohen), MontrĂ©al, Qc.; Centre pour lâamĂ©lioration de la qualitĂ© et la sĂ©curitĂ© des patients (Shojania), UniversitĂ© de Toronto; Centre des sciences de la santĂ© Sunnybrook (Shojania); Institut Vecteur (Ghassemi, Mamdani) et DĂ©partement des sciences informatiques (Ghassemi); FacultĂ© de pharmacie Leslie Dan (Mamdani), UniversitĂ© de Toronto, Toronto, Ont.; DĂ©partement de radiologie, UniversitĂ© Stanford (Cohen), Stanford, Calif.
Droit d'auteur © 2021 CMA Joule Inc. or its licensors
Il sâagit dâun article en libre accĂšs distribuĂ© conformĂ©ment aux modalitĂ©s de la licence Creative Commons Attributions (CC BY-NC-ND 4.0), qui permet lâutilisation, la diffusion et la reproduction dans tout mĂ©dium Ă la condition que la publication originale soit adĂ©quatement citĂ©e, que lâutilisation se fasse Ă des fins non commerciales (c.-Ă -d., recherche ou Ă©ducation) et quâaucune modification ni adaptation nây soit apportĂ©e. Voir: https://creativecommons.org/licenses/by-nc-nd/4.0/
Lâapprentissage machine a le potentiel de transformer le domaine de la santĂ©, mais ses applications en mĂ©decine clinique sont pour le moment limitĂ©es.
Les partenariats multidisciplinaires entre experts techniques et utilisateurs (professionnels de la santĂ©, gestionnaires, patients et membres de la famille) sont essentiels au dĂ©veloppement et Ă la mise en Ćuvre des solutions fondĂ©es sur lâapprentissage machine en santĂ©.
Un cadre en 3 phases peut ĂȘtre utilisĂ© pour dĂ©crire le dĂ©veloppement et lâadoption des solutions fondĂ©es sur lâapprentissage machine: une phase dâexploration pour comprendre le problĂšme Ă rĂ©gler et lâenvironnement de dĂ©ploiement, une phase de conception de la solution pour le dĂ©veloppement de modĂšles fondĂ©s sur lâapprentissage machine et dâoutils conviviaux, et une phase de mise en Ćuvre et dâĂ©valuation pour le dĂ©ploiement de la solution et lâĂ©valuation de ses retombĂ©es.
1. Liu Y, Chen PHC, Krause J, et al.. How to read articles that use machine learning: usersâ guides to the medical literature . JAMA
2019; 322 :1806â16. [ PubMed ] [ Google Scholar ]
2. Sinsky C, Colligan L, Li L, et al.. Allocation of physician time in ambulatory practice: a time and motion study in 4 specialties . Ann Intern Med
2016; 165 :753â60. [ PubMed ] [ Google Scholar ]
3. Topol EJ. High-performance medicine: the convergence of human and artificial intelligence . Nat Med
2019; 25 :44â56. [ PubMed ] [ Google Scholar ]
4. Ben-Israel D, Jacobs WB, Casha S, et al.. The impact of machine learning on patient care: a systematic review . Artif Intell Med
2020; 103 :101785. [ PubMed ] [ Google Scholar ]
5. Cohen JP, Cho T, Viviano JD, et al.. Problems in the deployment of machine-learned models in health care . CMAJ
2021.
Aug.
30 [cyberpublication avant impression]. doi: 10.1503/cmaj.202066. [ Article PMC gratuit ] [ PubMed ] [ CrossRef ]
6. Antoniou T, Mamdani MM. Evaluation of machine learning solutions in medicine . CMAJ
2021.
Aug.
30 [cyberpublication avant impression]. doi: 10.1503/cmaj.210036. [ Article PMC gratuit ] [ PubMed ] [ CrossRef ]
7. Chapman P, Clinton J, Kerber R, et al.. CRISP-DM 1.0: a step-by-step data mining guide . Armonk (NY): SPPS; 2000. Accessible ici: https://www.the-modeling-agency.com/crisp-dm.pdf (consulté le 18 mai 2021). [ Google Scholar ]
8. How to improve . Boston: Institute for Healthcare Improvement. Accessible ici: http://www.ihi.org/resources/Pages/HowtoImprove/default.aspx (consulté le 18 mai 2021). [ Google Scholar ]
9. Graham ID, Logan J, Harrison MB, et al.. Lost in knowledge translation: time for a map?
J Contin Educ Health Prof
2006; 26 :13â24. [ PubMed ] [ Google Scholar ]
10. van Galen LS, Struik PW, Driesen BEJM, et al.. Delayed recognition of deterioration of patients in general wards is mostly caused by human related monitoring failures: A root cause analysis of unplanned ICU admissions . PLoS One
2016; 11 :e0161393. doi: 10.1371/journal.pone.0161393. [ Article PMC gratuit ] [ PubMed ] [ CrossRef ] [ Google Scholar ]
11. Burch VC, Tarr G, Morroni C. Modified early warning score predicts the need for hospital admission and in-hospital mortality . Emerg Med J
2008; 25 :674â8. [ PubMed ] [ Google Scholar ]
12. McGinley A, Pearse RM. A national early warning score for acutely ill patients . BMJ
2012; 345 :e5310. [ PubMed ] [ Google Scholar ]
13. Linnen DT, Escobar GJ, Hu X, et al.. Statistical modeling and aggregate-weighted scoring systems in prediction of mortality and ICU transfer: a systematic review . J Hosp Med
2019; 14 :161â9. [ Article PMC gratuit ] [ PubMed ] [ Google Scholar ]
14. Verma AA, Guo Y, Kwan JL, et al.. Patient characteristics, resource use and outcomes associated with general internal medicine hospital care: the General Medicine Inpatient Initiative (GEMINI) retrospective cohort study . CMAJ Open
2017; 5 :E842â9. [ Article PMC gratuit ] [ PubMed ] [ Google Scholar ]
15. Quality Improvement Essentials Toolkit . Boston: Institute for Healthcare Improvement; 2021. Accessible ici: www.ihi.org/resources/Pages/Tools/Quality-Improvement-Essentials-Toolkit.aspx (consulté le 18 mai 2021). [ Google Scholar ]
16. Etchells E, Ho M, Shojania KG. Value of small sample sizes in rapid-cycle quality improvement projects . BMJ Qual Saf
2016; 25 :202â6. [ PubMed ] [ Google Scholar ]
17. Escobar GJ, Liu VX, Schuler A, et al.. Automated identification of adults at risk for in-hospital clinical deterioration . N Engl J Med
2020; 383 :1951â60. [ Article PMC gratuit ] [ PubMed ] [ Google Scholar ]
18. Weiskopf NG, Weng C. Methods and dimensions of electronic health record data quality assessment: enabling reuse for clinical research . J Am Med Inform Assoc
2013; 20 :144â51. [ Article PMC gratuit ] [ PubMed ] [ Google Scholar ]
19. Verma AA, Pasricha SV, Jung HY, et al.. Assessing the quality of clinical and administrative data extracted from hospitals: the General Medicine Inpatient Initiative (GEMINI) experience . J Am Med Inform Assoc
2021; 28 :578â87. [ Article PMC gratuit ] [ PubMed ] [ Google Scholar ]
20. Beam AL, Kohane IS. Big data and machine learning in health care . JAMA
2018; 319 :1317â8. [ PubMed ] [ Google Scholar ]
21. Shah NH, Milstein A, Bagley Steven CP. Making machine learning models clinically useful . JAMA
2019; 322 :1351â2. [ PubMed ] [ Google Scholar ]
22. Phansalkar S, Edworthy J, Hellier E, et al.. A review of human factors principles for the design and implementation of medication safety alerts in clinical information systems . J Am Med Inform Assoc
2010; 17 :493â501. [ Article PMC gratuit ] [ PubMed ] [ Google Scholar ]
23. Sendelbach S, Funk M. Alarm fatigue: a patient safety concern . AACN Adv Crit Care
2013; 24 :378â86. [ PubMed ] [ Google Scholar ]
24. Zheng K, Ratwani RM, Adler-Milstein J. Studying workflow and workarounds in electronic health recordâsupported work to improve health system performance . Ann Intern Med
2020; 172 ( Suppl 11 ):S116â22. [ Article PMC gratuit ] [ PubMed ] [ Google Scholar ]
25. Teich JM, Merchia PR, Schmiz JL, et al.. Effects of computerized physician order entry on prescribing practices . Arch Intern Med
2000; 160 :2741â7. [ PubMed ] [ Google Scholar ]
26. Koppel R, Metlay JP, Cohen A, et al.. Role of computerized physician order entry systems in facilitating medication errors . JAMA
2005; 293 :1197â203. [ PubMed ] [ Google Scholar ]
27. Craig P, Dieppe P, Macintyre S, et al.. Developing and evaluating complex interventions: the new Medical Research Council guidance . BMJ
2008; 337 : a1655. [ Article PMC gratuit ] [ PubMed ] [ Google Scholar ]
28. Michie S, van Stralen MM, West R. The behaviour change wheel: a new method for characterising and designing behaviour change interventions . Implement Sci
2011; 6 :42. [ Article PMC gratuit ] [ PubMed ] [ Google Scholar ]
29. Lee JD, See KA. Trust in automation: designing for appropriate reliance . Hum Factors
2004; 46 :50â80. [ PubMed ] [ Google Scholar ]
30. Asan O, Bayrak AE, Choudhury A. artificial intelligence and human trust in healthcare: focus on clinicians . J Med Internet Res
2020; 22 :e15154. [ Article PMC gratuit ] [ PubMed ] [ Google Scholar ]
31. Nestor B, McCoy LG, Verma AA, et al.. Preparing a clinical support model for silent mode in general internal medicine . Proceedings of the 5th Machine Learning for Healthcare Conference, PMLR
2020; 126 :950â72. Accessible ici: https://static1.squarespace.com/static/59d5ac1780bd5ef9c396eda6/t/5f22ccecc4a74012a31d4b4a/1596116209243/155_CameraReadySubmission_155_nestor2020preparing.pdf (consultĂ© le 14 sept. 2021). [ Google Scholar ]
32. Lundberg SM, Nair B, Vavilala MS, et al.. Explainable machine-learning predictions for the prevention of hypoxaemia during surgery . Nat Biomed Eng
2018; 2 :749â60. [ Article PMC gratuit ] [ PubMed ] [ Google Scholar ]
33. Lipton ZC. The mythos of model interpretability: In machine learning, the concept of interpretability is both important and slippery . Queue
2018; 16 :1â28. doi: 10.1145/3236386.3241340. [ CrossRef ] [ Google Scholar ]
34. Payrovnaziri SN, Chen Z, Rengifo-Moreno P, et al.. Explainable artificial intelligence models using real-world electronic health record data: a systematic scoping review . J Am Med Inform Assoc
2020; 27 :1173â85. [ Article PMC gratuit ] [ PubMed ] [ Google Scholar ]
35. Carvalho DV, Pereira EM, Cardoso JS. Machine learning interpretability: a survey on methods and metrics . Electronics
2019; 8 :832. [ Google Scholar ]
36. Bombard Y, Baker GR, Orlando E, et al.. Engaging patients to improve quality of care: a systematic review . Implement Sci
2018; 13 :98. doi: 10.1186/s13012-018-0784-z. [ Article PMC gratuit ] [ PubMed ] [ CrossRef ] [ Google Scholar ]
37. Sharma AE, Knox M, Mleczko VL, et al.. The impact of patient advisors on healthcare outcomes: a systematic review . BMC Health Serv Res
2017; 17 :693. doi: 10.1186/s12913-017-2630-4. [ Article PMC gratuit ] [ PubMed ] [ CrossRef ] [ Google Scholar ]
38. Kiran T, Tepper J, Gavin F. Working with patients to improve care . CMAJ
2020; 192 : E125â7. [ Article PMC gratuit ] [ PubMed ] [ Google Scholar ]
39. Hamilton C, Hoens AM, Backman CL, et al.. Workbook to guide the development of a patient engagement in research (PEIR) plan . Richmond (BC): Arthritis Research Canada and Vancouver: University of British Columbia; 2018. Accessible ici: http://www.arthritisresearch.ca/wp-content/uploads/2018/06/PEIR-Plan-Guide.pdf (consulté le 9 juin 2021). [ Google Scholar ]
40. Taylor MJ, McNicholas C, Nicolay C, et al.. Systematic review of the application of the plan-do-study-act method to improve quality in healthcare . BMJ Qual Saf
2014; 23 :290â8. [ Article PMC gratuit ] [ PubMed ] [ Google Scholar ]
41. Leis JA, Shojania KG. A primer on PDSA: executing plan-do-study-act cycles in practice, not just in name . BMJ Qual Saf
2017; 26 :572â7. [ PubMed ] [ Google Scholar ]
42. Blier N. Stories of AI failure and how to avoid similar AI fails [blog] . Amherst (MA): Lexalytics; 2020.
Jan
30. Accessible ici: https://www.lexalytics.com/lexablog/stories-ai-failure-avoid-ai-fails-2020 (consulté le 18 mai 2021). [ Google Scholar ]
Articles from CMAJ : Canadian Medical Association Journal are provided here courtesy of Canadian Medical Association
1. Liu Y, Chen PHC, Krause J, et al.. How to read articles that use machine learning: usersâ guides to the medical literature . JAMA
2019; 322 :1806â16. [ PubMed ] [ Google Scholar ] [ Ref list ]
2. Sinsky C, Colligan L, Li L, et al.. Allocation of physician time in ambulatory practice: a time and motion study in 4 specialties . Ann Intern Med
2016; 165 :753â60. [ PubMed ] [ Google Scholar ] [ Ref list ]
3. Topol EJ. High-performance medicine: the convergence of human and artificial intelligence . Nat Med
2019; 25 :44â56. [ PubMed ] [ Google Scholar ] [ Ref list ]
4. Ben-Israel D, Jacobs WB, Casha S, et al.. The impact of machine learning on patient care: a systematic review . Artif Intell Med
2020; 103 :101785. [ PubMed ] [ Google Scholar ] [ Ref list ]
5. Cohen JP, Cho T, Viviano JD, et al.. Problems in the deployment of machine-learned models in health care . CMAJ
2021.
Aug.
30 [cyberpublication avant impression]. doi: 10.1503/cmaj.202066. [ Article PMC gratuit ] [ PubMed ] [ CrossRef ] [ Ref list ]
6. Antoniou T, Mamdani MM. Evaluation of machine learning solutions in medicine . CMAJ
2021.
Aug.
30 [cyberpublication avant impression]. doi: 10.1503/cmaj.210036. [ Article PMC gratuit ] [ PubMed ] [ CrossRef ] [ Ref list ]
7. Chapman P, Clinton J, Kerber R, et al.. CRISP-DM 1.0: a step-by-step data mining guide . Armonk (NY): SPPS; 2000. Accessible ici: https://www.the-modeling-agency.com/crisp-dm.pdf (consulté le 18 mai 2021). [ Google Scholar ] [ Ref list ]
8. How to improve . Boston: Institute for Healthcare Improvement. Accessible ici: http://www.ihi.org/resources/Pages/HowtoImprove/default.aspx (consulté le 18 mai 2021). [ Google Scholar ] [ Ref list ]
9. Graham ID, Logan J, Harrison MB, et al.. Lost in knowledge translation: time for a map?
J Contin Educ Health Prof
2006; 26 :13â24. [ PubMed ] [ Google Scholar ] [ Ref list ]
10. van Galen LS, Struik PW, Driesen BEJM, et al.. Delayed recognition of deterioration of patients in general wards is mostly caused by human related monitoring failures: A root cause analysis of unplanned ICU admissions . PLoS One
2016; 11 :e0161393. doi: 10.1371/journal.pone.0161393. [ Article PMC gratuit ] [ PubMed ] [ CrossRef ] [ Google Scholar ] [ Ref list ]
11. Burch VC, Tarr G, Morroni C. Modified early warning score predicts the need for hospital admission and in-hospital mortality . Emerg Med J
2008; 25 :674â8. [ PubMed ] [ Google Scholar ] [ Ref list ]
12. McGinley A, Pearse RM. A national early warning score for acutely ill patients . BMJ
2012; 345 :e5310. [ PubMed ] [ Google Scholar ] [ Ref list ]
13. Linnen DT, Escobar GJ, Hu X, et al.. Statistical modeling and aggregate-weighted scoring systems in prediction of mortality and ICU transfer: a systematic review . J Hosp Med
2019; 14 :161â9. [ Article PMC gratuit ] [ PubMed ] [ Google Scholar ] [ Ref list ]
14. Verma AA, Guo Y, Kwan JL, et al.. Patient characteristics, resource use and outcomes associated with general internal medicine hospital care: the General Medicine Inpatient Initiative (GEMINI) retrospective cohort study . CMAJ Open
2017; 5 :E842â9. [ Article PMC gratuit ] [ PubMed ] [ Google Scholar ] [ Ref list ]
15. Quality Improvement Essentials Toolkit . Boston: Institute for Healthcare Improvement; 2021. Accessible ici: www.ihi.org/resources/Pages/Tools/Quality-Improvement-Essentials-Toolkit.aspx (consulté le 18 mai 2021). [ Google Scholar ] [ Ref list ]
16. Etchells E, Ho M, Shojania KG. Value of small sample sizes in rapid-cycle quality improvement projects . BMJ Qual Saf
2016; 25 :202â6. [ PubMed ] [ Google Scholar ] [ Ref list ]
17. Escobar GJ, Liu VX, Schuler A, et al.. Automated identification of adults at risk for in-hospital clinical deterioration . N Engl J Med
2020; 383 :1951â60. [ Article PMC gratuit ] [ PubMed ] [ Google Scholar ] [ Ref list ]
19. Verma AA, Pasricha SV, Jung HY, et al.. Assessing the quality of clinical and administrative data extracted from hospitals: the General Medicine Inpatient Initiative (GEMINI) experience . J Am Med Inform Assoc
2021; 28 :578â87. [ Article
L'Ă©talon dĂ©licat et passionnĂ© Ă©clate les fesses de sa belle-sĆur
Perfore son jeune cul pour la premiĂšre fois
Grosse explosion de sperme pour une grosse femme sexy aux seins naturels