Secretary Problem
🔞 ALL INFORMATION CLICK HERE 👈🏻👈🏻👈🏻
Secretary Problem
All languages English Russian Chinese German French Spanish Swedish Italian Latin Finnish Kazakh Greek Uzbek Welsh Arabic Belarusian Swahili Hebrew Norwegian Portuguese Hungarian Turkish Indonesian Polish Komi Estonian Latvian Dutch Danish Albanian Croatian Nahuatl Armenian Ukrainian Japanese Sanskrit Thai Irish Tatar Slovak Slovene Tuvan Urdu Faroese Yiddish Macedonian Catalan Bashkir Czech Korean Georgian Romanian, Moldavian Yakut Kyrgyz Tibetan Standard Icelandic Bulgarian Serbian Vietnamese Azerbaijani Basque Hindi Māori Quechua Akan Aymara Haitian Mongolian Pāli Maya Lithuanian Shor Crimean Tatar language Esperanto Ingush Northern Sami Hornjoserbska Chechen Sumerian Scottish Gaelic Ossetian Circassian Adyghe Persian Ainu Khmer Old Russian Old Church Slavonic Mycenaean Quenya Yupik Afrikaans Papiamento Panjabi Tagalog Moksha Cree Waray Kurdish Alsatian Abkhaz Aragonese Aromanian Asturian Erzya Komi Mari Chuvash Ladino Udmurt Veps Altaic Dolgan Karachay Kumyk Nogai Ottoman Turkish Tofalar Turkmen Uighur Idioma urrumano Manchu Buryat Orok Evenki Guaraní Tajik Inupiaq Malay Kabyle Twi Lingala Bagobo Yoruba Silesian Luxembourgish Cherokee Cheyenne Klingon Lojban
All languages Russian English Danish Tatar German Latin Kazakh Ukrainian Hungarian Turkish Tajik Persian Spanish Hebrew Norwegian Chinese French Italian Portuguese Arabic Polish Swahili Dutch Croatian Catalan Galician Georgian Belarusian Albanian Kurdish Greek Slovene Indonesian Bulgarian Vietnamese Māori Tagalog Urdu Icelandic Hindi Irish Faroese Latvian Lithuanian Finnish Mongolian Swedish Thai Pāli Japanese Macedonian Korean Estonian Romanian, Moldavian Chechen Karachay Slovak Czech Serbian Armenian Azerbaijani Uzbek Quechua Haitian Maya Aymara Shor Esperanto Crimean Tatar language Ossetian Adyghe Yakut Ainu Khmer Old Russian Old Church Slavonic Tamil Quenya Avaric Afrikaans Papiamento Moksha Yoruba Alsatian Yiddish Abkhaz Erzya Ingush Ingrian Mari Chuvash Udmurt Votic Veps Altaic Kumyk Turkmen Uighur Idioma urrumano Evenki Bashkir Malay Maltese Lingala Panjabi Cherokee Chamorro Klingon Basque Pashto
Мы разработали для вас новый сервис!
Пользуясь Скидки.Академик.ру , вы поддерживаете Академик.ру!
The secretary problem is an optimal stopping problem that has been studied extensively in the fields of applied probability , statistics , and decision theory . It is also known as the marriage problem , the sultan ' s dowry problem , the fussy suitor problem , and the best choice problem . The problem can be stated as follows:
# There is a single secretarial position to fill . # There are n applicants for the position , and the value of n is known . # The applicants can be ranked from best to worst with no ties . # The applicants are interviewed sequentially in a random order , with each order being equally likely . # After each interview , the applicant is accepted or rejected . # The decision to accept or reject an applicant can be based only on the relative ranks of the applicants interviewed so far . # Rejected applicants cannot be recalled . # The object is to select the best applicant . The payoff is 1 for the best applicant and zero otherwise .
Let us say that an applicant is a " candidate " only if it is better than all the applicants viewed previously . Clearly , since the objective in the problem is to select the single best applicant , only candidates will be considered for acceptance . One reason why the secretary problem has received so much attention is that the optimal policy for the problem ( the stopping rule ) has a surprising feature . Specifically , for large n the optimal policy is to skip the first n / e applicants ( where e is the base of the natural logarithm ) and then to accept the next candidate --- an applicant that is better than all those previously interviewed . As n gets larger , the probability of selecting the best applicant from the pool goes to 1 / e , which is around 37 %. Whether one is searching through 100 or 100 , 000 , 000 applicants , the optimal policy will select the single best one about 37 % of the time .
The optimal policy for the problem is a stopping rule . Under it , the interviewer should skip the first r - 1 applicants , and then take the next applicant who is a candidate ( i . e ., who has the best relative ranking of those interviewed up to that point ). For an arbitrary cutoff r , the probability that the best applicant is selected is
: P ( r )= sum _{ j = r }^{ n } left ( frac { 1 }{ n }
ight ) left ( frac { r - 1 }{ j - 1 }
ight )= left ( frac { r - 1 }{ n }
ight ) sum _{ j = r }^{ n } left ( frac { 1 }{ j - 1 }
ight ).
Letting n tend to infinity , writing x as the limit of r / n , using t for j / n and dt for 1 / n , the sum can be approximated by the integral
: P ( r )= x int _{ x }^{ 1 } frac { 1 }{ t }, dt = - x log ( x ).
Taking the derivative of P ( r ) with respect to x , setting it to 0 , and solving for x , we find that the optimal x is equal to 1 / e . Thus , the optimal cutoff tends to n / e as n increases , and the best applicant is selected with probability 1 / e .
For small values of n , the optimal r can also be obtained by standard dynamic programming methods . The optimal thresholds r and probability of selecting the best alternative P for several values of n are shown in the following table .
Note that the probability of selecting the best alternative converges quite rapidly toward 1 / eapprox 0 . 368 .
The secretary problem and several modifications of this problem can be solved in a straightforward manner by the Odds algorithm .
Stein , Seale , and Rapoport ( 2003 ) derived the expected success probabilities for several psychologically plausible heuristics that might be employed in the secretary problem . The heuristics they examined were:
* The Cutoff Rule ( CR ) : Do not accept any of the first y applicants ; thereafter , select the first encountered candidate ( i . e ., an applicant with relative rank 1 ). This rule has as a special case the optimal policy for the CSP for which y = r . * Candidate Count Rule ( CCR ) : Select the y encountered candidate . Note , that this rule does not necessarily skip any applicants ; it only considers how many candidates have been observed , not how deep the decision maker is in the applicant sequence . * Successive Non - Candidate Rule ( SNCR ) : Select the first encountered candidate after observing y non - candidates ( i . e ., applicants with relative rank > 1 ).
Note that each heuristic has a single parameter y . The figure ( shown on right ) displays the expected success probabilities for each heuristic as a function of y for problems with n = 80 .
Finding the single best applicant might seem like a rather strict objective . One can imagine that the interviewer would rather hire a higher - valued applicant than a lower - valued one , and not only be concerned with getting the best . That is , she will derive some value from selecting an applicant that is not necessarily the best , and the value she derives is increasing in the value of the one she selects .
To model this problem , suppose that the n applicants have " true " values that are random variables X drawn i . i . d . from a uniform distribution on [ 0 , 1 ] . Similar to the classical problem described above , the interviewer only observes whether each applicant is the best so far ( a candidate ), must accept or reject each on the spot , and " must " accept the last one if he is reached . ( To be clear , the interviewer does not learn the actual relative rank of " each " applicant . She learns only whether the applicant has relative rank 1 .) However , in this version her " payoff " is given by the true value of the selected applicant . For example , if she selects an applicant whose true value is 0 . 8 , then she will earn 0 . 8 . The interviewer ' s objective is to maximize the expected value of the selected applicant .
Since the applicant ' s values are i . i . d . draws from a uniform distribution on [ 0 , 1 ] , the expected value of the t th applicant given that x _{ t }= maxleft { x _{ 1 }, x _{ 2 }, ldots , x _{ t }
ight } isgiven by
: E _{ t }= Eleft ( X _{ t }| I _{ t }= 1
ight )= frac { t }{ t + 1 }.
As in the classical problem , the optimal policy is given by a threshold , which for this problem we will denote by c , at which the interviewer should begin accepting candidates . [ http: // dx . doi . org / 10 . 1016 / j . jmp . 2005 . 11 . 003 Bearden ( 2006 ) ] showed that c is either lfloor sqrt n
floor or lceil sqrt n
ceil . This follows from the fact that given a problem with n applicants , the expected payoff for some arbitrary threshold 1leq c leq n is
: V _{ n }( c )= sum _{ t = c }^{ n - 1 } left [ prod _{ s = c }^{ t - 1 } left ( frac { s - 1 }{ s }
ight )
ight ] left ( frac { 1 }{ t + 1 }
ight )+ left [ prod _{ s = c }^{ n - 1 } left ( frac { s - 1 }{ s }
ight )
ight ] frac { 1 }{ 2 }={ frac { 2cn -{ c }^{ 2 }+ c - n }{ 2cn .
Differentiating V _{ n }( c ) with respect to c , one gets partial V / partialc = left (-{ c }^{, 2 }+ n
ight )/ left ( 2 { c }^{, 2 } n
ight ) . Since partial ^{, 2 } V / partial c ^{, 2 }< 0 for all permissiblevalues of c , we find that V is maximized at c = sqrt n . Since V is convex in c , the optimal integer - valued threshold must be either lfloor sqrt n
floor or lceil sqrt n
ceil . Thus , for most values of n the interviewer will begin accepting applicants sooner in the cardinal payoff version than in the classical version where the objective is to select the single best applicant . Note that this is not an asymptotic result: It holds for all n .
A number of other variations of the classical secretary problem have been proposed . [ Many of these are reviewed in Freeman ( 1983 ). ]
Psychologists and experimental economists have studied the decision behavior of actual people in secretary problems [ Bearden , Murphy , and Rapoport , 2006 ; Bearden , Rapoport , and Murphy , 2006 ; Seale and Rapoport , 1997 ] . In large part , this work has shown that people tend to stop searching too soon . This may be explained , at least in part , by the cost of evaluating candidates . Extrapolating to real world settings , this might suggest that people do not search enough whenever they are faced with problems where the decision alternatives are encountered sequentially . For example , when trying to decide at which gas station to stop for gas , people might not search enough before stopping . If true , then they would tend to pay more for gas than they might had they searched longer . The same may be true when people search online for airline tickets , say . Experimental research on problems such as the secretary problem is sometimes referred to as behavioral operations research .
* Optimal stopping * Odds algorithm * Search theory
* [ http: // dx . doi . org / 10 . 1016 / j . jmp . 2005 . 11 . 003 J . N . Bearden . " A new secretary problem with rank - based selection and cardinal payoffs ." " Journal of Mathematical Psychology ", volume 50 , pp . 58 - 59 . 2006 . ] * [ http: // dx . doi . org / 10 . 1016 / j . jmp . 2005 . 08 . 002 J . N . Bearden , R . O . Murphy , Rapoport , A . " A multi - attribute extension of the secretary problem: Theory and experiments ." " Journal of Mathematical Psychology ", volume 49 , pp . 410 - 425 . 2005 . ] * [ http: // dx . doi . org / 10 . 1287 / mnsc . 1060 . 0535 J . N . Bearden , A . Rapoport , R . O . Murphy . " Sequential observation and selection with rank - dependent payoffs: An experimental test ." " Management Science ", volume 52 , pp . 1437 - 1449 . 2006 . ] * F . Thomas Bruss " Sum the odds to one and stop ," Annals of Probability , Vol . 28 . 1384 - 1391 . ( 2000 ) * T . S . Ferguson . [ A mathematics professor at UCLA and the father of Chris " Jesus " Ferguson , the professional poker player ] " Who solved the secretary problem ?" " Statistical science ", volume 4 , pp . 282 - 296 . 1989 . * P . R . Freeman . " The secretary problem and its extensions: A review ." International Statistical Review / Revue Internationale de Statistique , volume 51 , pp . 189 - 206 . 1983 . * [ http: // dx . doi . org / 10 . 1006 / obhd . 1997 . 2683 D . A . Seale , A . Rapoport . " Sequential decision making with relative ranks: An experimental investigation of the ' secretary problem .'" Organizational Behavior and Human Decision Processes , volume 69 , pp . 221 - 236 . 1997 . ] * [ http: // dx . doi . org / 10 . 1016 / S0377 - 2217 ( 02 ) 00601 - X W . E . Stein , D . A . Seale , A . Rapoport . " Analysis of heuristic solutions to the best choice problem ." European Journal of Operational Research , volume 151 , pp . 140 - 152 . ]
* [ http: // www . utilitymill . com / utility / Secretary _ Problem _ Optimizer Online Utility to Calculate Optimal r ] * * [ http: // www . behavioral - or . org behavioral - or . org J . Neil Bearden ' s Home Page ] * [ http: // www . math . ucla . edu /~ tom / Stopping / Contents . html Optimal Stopping and Applications book by Thomas S . Ferguson ] * [ http: // www . mathpages . com / home / kmath018 . htm Optimizing Your Wife ] at MathPages
Secretary General of NATO — Secretary General Anders Fogh Rasmussen ( right ) at the 2004 Istanbul summit , prior to his appointment The Secretary General of NATO is an international diplomat who serves as the chief official of the North Atlantic Treaty Organization . The … … Wikipedia
Robbins ' problem ( of optimal stopping ) — is a problem of optimal stopping , sometimes referred to as the fourth secretary problem or the problem of minimizing the expected rank with full information . Its statement is as follows . Let X 1 , ... , X n be independent , identically distributed … … Wikipedia
Toy problem — In mathematics and information science , a toy problem is a problem that is not of immediate scientific interest , yet is used as an expository device to illustrate a trait that may be shared by other , more complicated , instances of the problem , or … Wikipedia
Marriage problem — In mathematics , marriage problem can refer to: the assignment problem the secretary problem the stable marriage problem This disambiguation page lists articles associated with the same title . If an … Wikipedia
British Chess Problem Society — The British Chess Problem Society is the oldest chess problem society in the world . [ cite news | first = Nigel | last = Short | authorlink = Nigel Short | title = The Sunday chess column | url = http: // www . telegraph . co . uk / arts / main . jhtml ; sessionid = H3PMS0XK5QTTRQ … … Wikipedia
Condoleezza Rice ' s tenure as Secretary of State — Rice signs official papers after receiving the oath of office during her ceremonial swearing in at the Department of State . Watching on are , from left , Laura Bush , Justice Ruth Bader Ginsburg , President George W . Bush and an unidentified family … … Wikipedia
Midnight Secretary — Cover of Midnight Secretary volume 1 as published by Shogakukan featuring Kaya Satozuka ( right ) and Kyouhei Touma ( ミッドナイト · セクレタリ ) … Wikipedia
Year 2000 problem — Y2K redirects here . For other uses , see Y2K ( disambiguation ). The ( French ) sign reads 3 January 1900 instead of 3 January 2000 The Year 2000 problem ( also known as the Y2K problem , the Millennium bug , the Y2K bug , or simply Y2K ) was a problem for … Wikipedia
Harold Brown ( Secretary of Defense ) — Infobox US Cabinet official name = Harold Brown order = 14th title = United States Secretary of Defense term start = January 21 , 1977 term end = January 20 , 1981 predecessor = Donald Rumsfeld successor = Caspar Weinberger birth date = birth date and … … Wikipedia
Dimmock v Secretary of State for Education and Skills — was a case heard in September – October 2007 in the High Court of Justice of England and Wales , concerning the permissibility of the government providing Al Gore s documentary An Inconvenient Truth to English state schools as a teaching aid . The … … Wikipedia
Secretary Problem (A Optimal Stopping Problem ) - GeeksforGeeks
Secretary problem | Словари и энциклопедии на Академике
Math-Based Decision Making: The Secretary Problem | by Lorenzo... | Medium
Why is 37 the solution to the classic secretary problem ? - Quora
Secretary problem - Infogalactic: the planetary knowledge core
Math-Based Decision Making: The Secretary Problem
Physicist passionate about sustainability and applied probability. Track&field freak. PhD Student in Computational Biology.
The secretary problem is a famous riddle on the topic of decision making. It is about finding the best strategy when choosing between a sequence of alternatives of which you do not know the best one.
The statement of the secretary problem goes as follows:
You are the HR manager of a company and need to hire the best secretary out of a given number N of candidates. You can interview them one by one, in random order. However, the decision of appointing or rejecting a particular applicant must be taken immediately after the interview. If nobody has been accepted before the end, the last candidate is chosen. What strategy do you use to maximize the chances to hire the best applicant?
At first glance, this problem might appear impossible or seem like a trickery. In reality, the secretary problem has an elegant solution based on sound math.
The practical wisdom coming from the secretary problem is usually lost among the pages of books of probability theory. I think this is a true pity, because there are many situations where knowing the optimal strategy for unseen alternatives can come useful. For example,
In all these cases, you don’t know what options will come next. However, you might want to decide fast but also appropriately.
The goal of this post is to unveil the solution of the secretaty problem in intelligible words and, only when necessary, a few illustrated steps of math.
You need to make an important decision before proceeding.
You need to decide if you want to read the riddle again and think about the solution on your own. I strongly recommend this, because this is pure brain gymnastics, regardless of you realizing the best strategy or not. If instead you are impatient, then you can also jump straight to the conclusion.
But as Buddha said, “ it is better to travel well than to arrive ”.
The secretary problem is challenging because you can’t realize at any point of the interview process if the current applicant is the best one. You can only make comparisons with the candidates interviewed so far. But even when the current candidate has proven to be brilliant, the actual best one could always come right after him.
In front of this complete uncertainty, a tempting strategy is to abandon yourself to luck. You might consider to make an arbitrary decision, such as “ Whatever, I choose the first one ”. Not surprisingly, this random strategy performs poorly. You only have a probability P=1/N that the first applicant will be the best one. The same is true also when choosing always the last applicant or always the n th one: your odds are always 1/N for any prearranged position.
The random strategy gets worse fast as you increase the number of applicants. If you had just N=3 candidates, choosing at random would only work 33% of the time. With N=10 applicants, the chances of randomly picking the best candidate are a miserable 10%. With N=100 , just 1%. These numbers aren’t acceptable for a respectable HR manager.
Is there a viable strategy to outdo randomness?
At this point, you might have realized that the only control variable you have on the entire interview process is rejecting people.
Your strategy can only consist in deciding how many people you want to reject before starting to decide for real. Essentially, you want to wait long enough to get a good reference point and then choose for the next candidate that beats your reference.
More quantitatively, the strategy reads:
This strategy sounds promising but it is not yet complete: you need to establish the number R of people to reject.
If R is too large, you can build a strong benchmark but you risk to reject the best candidate too. If instead R is too small, you’ll have a dull reference point and you are likely to choose a suboptimal candidate. What we need to do is to find the optimal value R* of people to reject, given N candidates in total . In order to get this, we’ll need some math.
Before starting with math, however, it’s always smart to double-check that the overall idea makes sense. It is convenient to test the considered strategy in the case of N=3 applicants. Here, the only sensical value of people to reject is R=1 . Otherwise, you’d always choose the last candidate if R=2 , or the first one if R=0 , and both cases would be just the random strategy.
The figure below illustrates all the 6 possible arrival combinations of three candidates, together with the respective outcomes of the strategy “ reject the first applicant and then pick the next best one (otherwise the last) ”.
Our strategy is able to identify the best secretary in three out of the six scenarios. That is, we have a success probability P=1/2. This is definitely better than P=1/3 of the random strategy. Now that our method seems to work, it is worth doing some math.
First, we need to calculate the success probability P(R) of picking the best candidate for some value R of rejected candidates. The success probability can be thought as the sum of the probabilities of finding the best candidate in position n , where n can be comprised between R+1 and the total N (i.e. the remaining candidates):
Recall that the accepted candidate is the first one beating the top score of the R rejected candidates. However, this doesn’t guarantee that he is also the best candidate overall .
So, how to calculate the probability that the n th applicant is both chosen and the best one? There are two possible ways.
The chosen n th candidate is for sure also the best candidate whenever the second-best one was rejected at the beginning. This means that our benchmark was tuned so high to keep rejecting anyone else but the best applicant.
In terms of math, this reasoning translates as follows:
The last passage just brings the factors independent of n out of the sum. That’s it. We have the formula.
Let’s now consider another insightful way to get to this result.
The alternative way to require that the n th candidate is both chosen and the best one is to imagine to go sequentially through all of the remaining candidates from R+1 to N and throw a special coin for each.
The coin decides whether that candidate will register a new record score. Due to random ordering, a record happens with probability 1/m , where m is the position of the current candidate. We want only one record outcome for the coin of the n th candidate, whereas all the other coins need to fail a new record with probability 1–1/m .
The mathematical equivalent of this idea reads
The last row is just some algebraic manipulation. We verified that the formula is the same as what obtained in the previous way. In math, it is a beautiful moment when two different approaches lead to the same result: it is often a strong hint that you aren’t messing up.
Now that we have a bullet-proof formula for P(R) , we can easily compute it numerically for a given number of candidates and see what is the optimal number of rejected people R* that maximizes the success probability P(R) .
This can be easily implemented, for instance in the language Julia, as follows:
Below, you can see a plot of the optimal R* as N is increased and the corresponding success probability P(R*) .
We can note two interesting trends. First, the success probability with the optimal strategy does not miserably decay towards zero for large N , as for the random choice. This is astounding. With the optimal strategy, the probability of picking exactly the best candidate doesn’t decrease if there are arbitrarily many candidates. Just think about it: it’s more likely to pick the best candidate out of 100 with the optimal strategy than to succeed by choosing at random between 3 applicants.
Secondly, the optimal value R* follows a simple linear relationship with N . Can we extract a practical rule of the thumb out of this? Yes, it is the 1/e Law.
The 1/ e Law takes its name from the asymptotic behaviour of the success probability: the ratio 1/ e corresponds precisely to the value at which the success probability P(R*) converges for large N . Here, the letter e denotes the Euler’s number , and indeed 1/ e is about 1/2.72≈0.37, as can be seen from the plot.
Similarly, we observe that the optimal number of rejected candidates R* grows with N akin to a ladder, by taking a unit jump every 3 or sometimes 2 units. Guess what? The exact slope is again 1/ e. This means that the optimal R* can be effectively approximated by computing N/e .
The seemingly magical value 1/ e can be explicitly derived by approximating the second last row in the figure “Passage 2(b)” for large R and N , but I’ll omit this computation here. Importantly, the 1/ e Law holds true also for more general instances of the secretary problem, for example when also the total number of applicants is uknown but we are given a deadline by which the candidates can apply. For more details about the latter scenario, you can consult this open access paper .
Do you want to choose at best among a number of take-or-leave alternatives that you don’t know beforehand? Then:
1) reject approximately the first N/2.7 alternatives;
2) choose the next one that outdoes all those seen so far.
Special thanks to my dear friend Luca Amato for exposing me to this beautiful math problem.
Family Young Teen Nudist
Camping Xxx Sexy Naked Nudist Women
Big Pussy Masturbating
Sex Lesbian Women
Ass Lingerie Pics




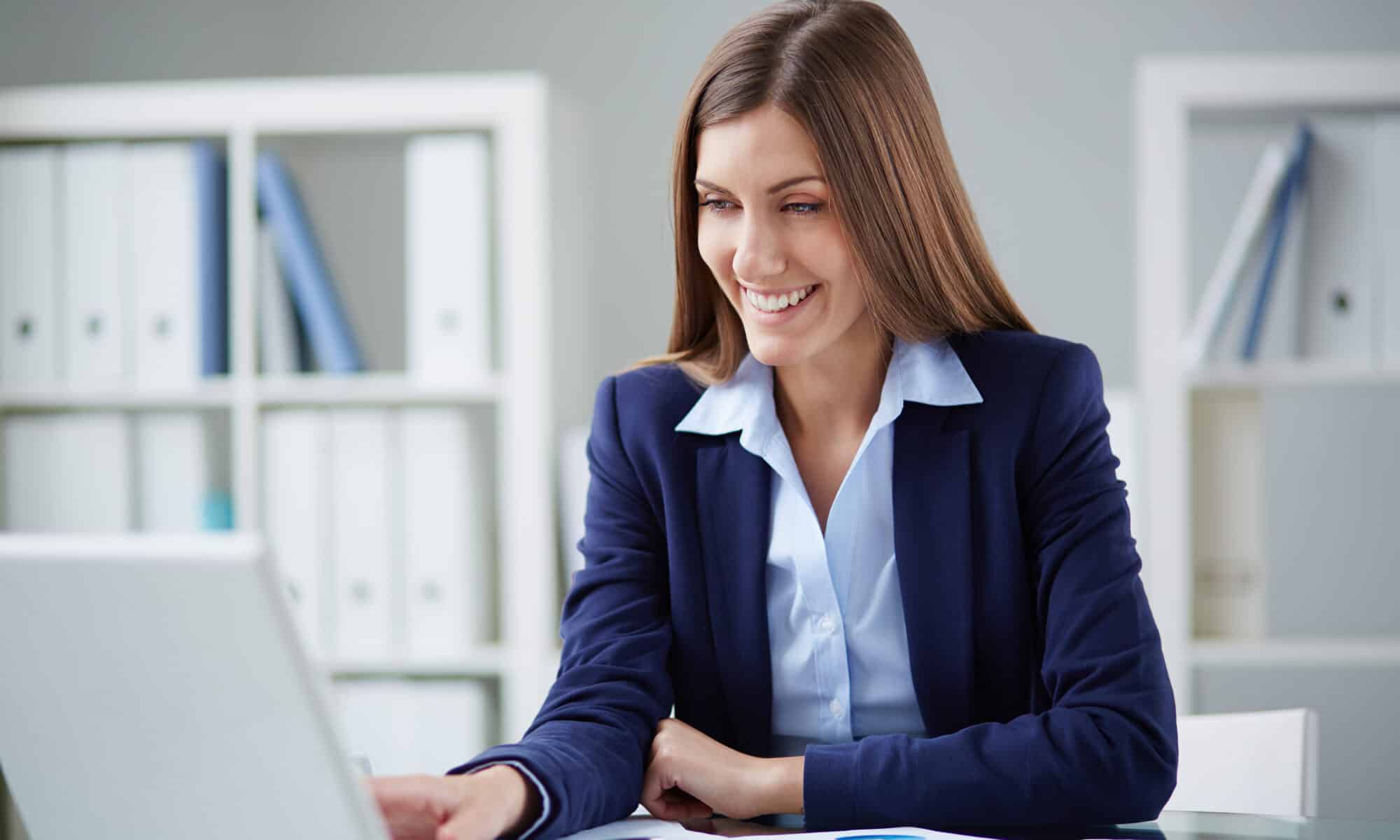

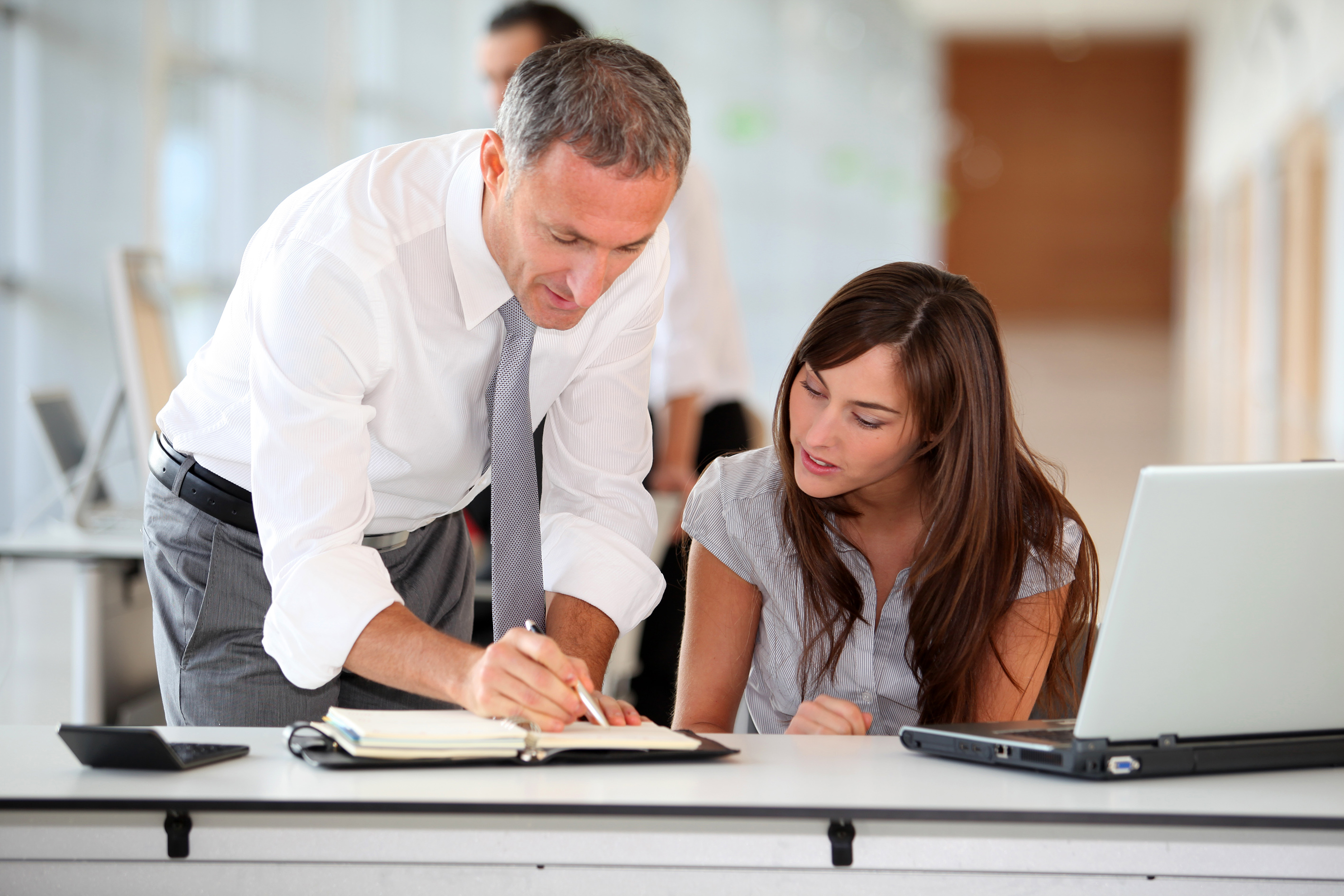
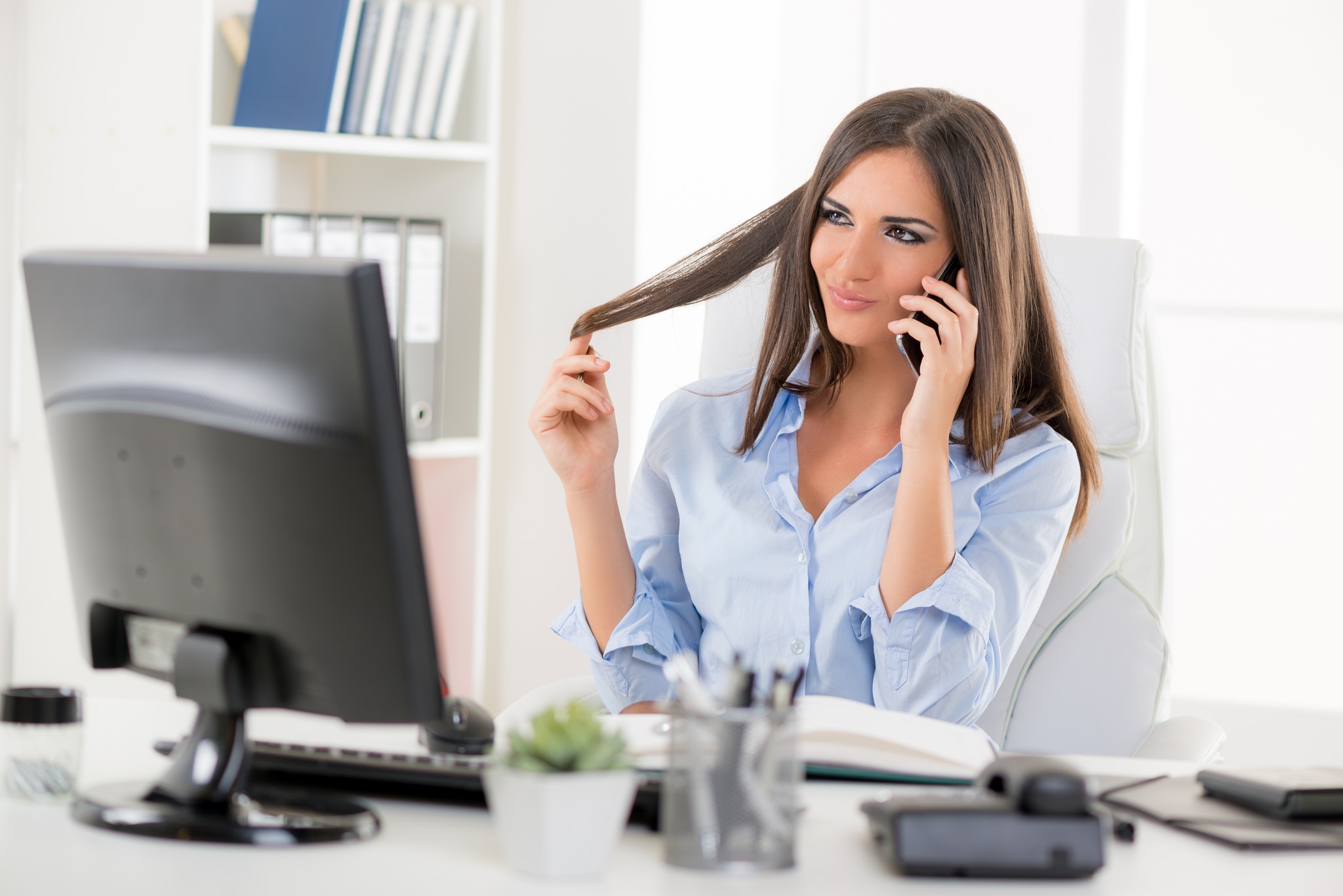


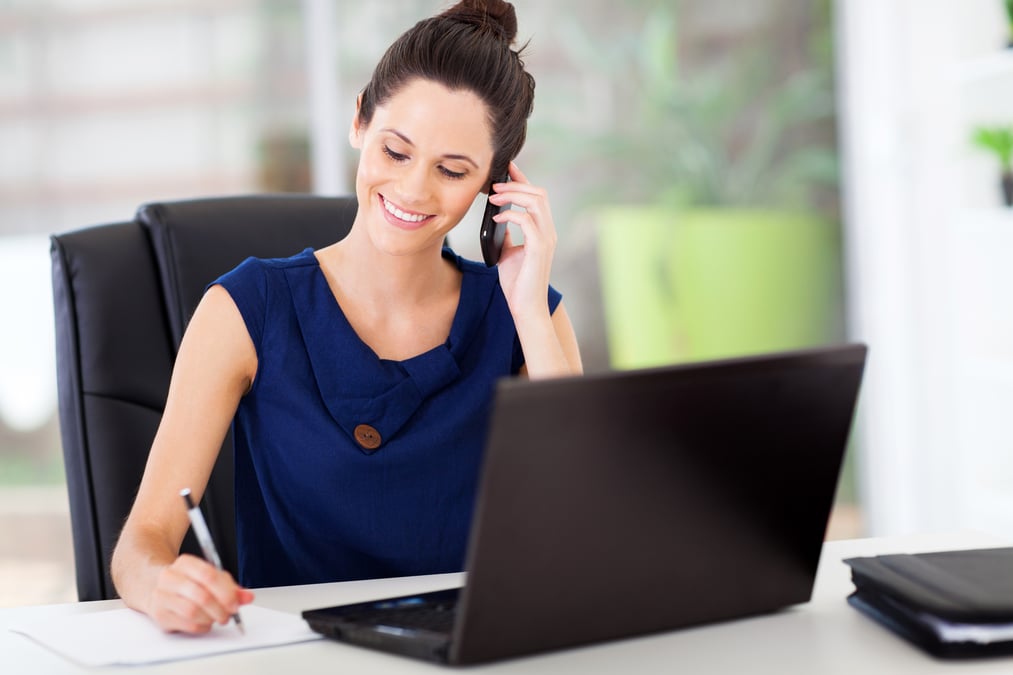



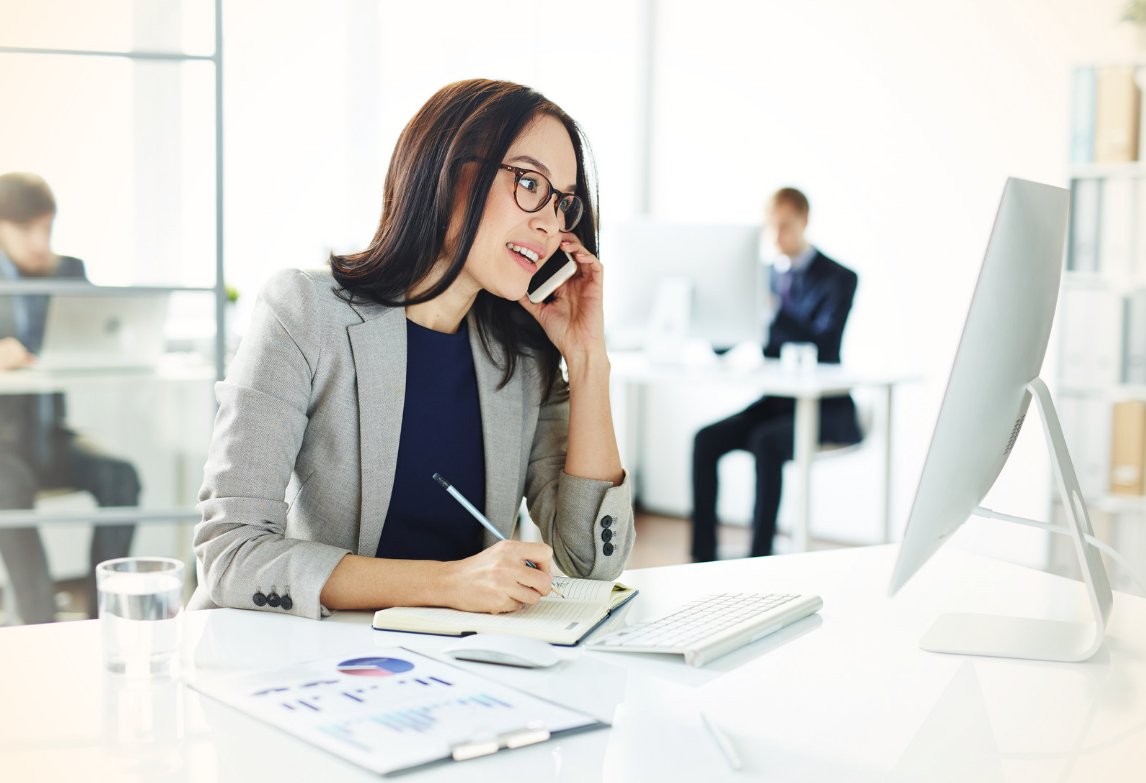





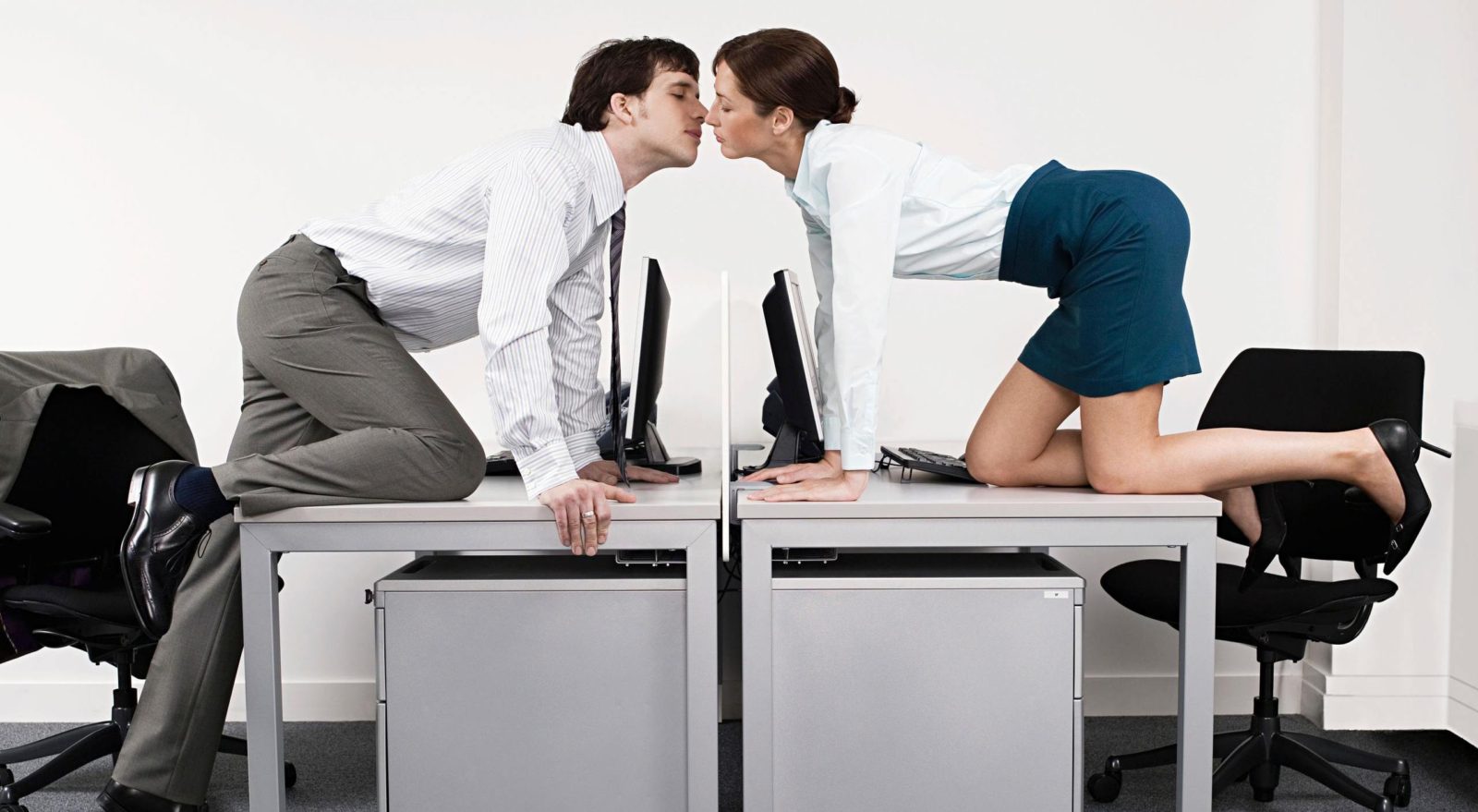









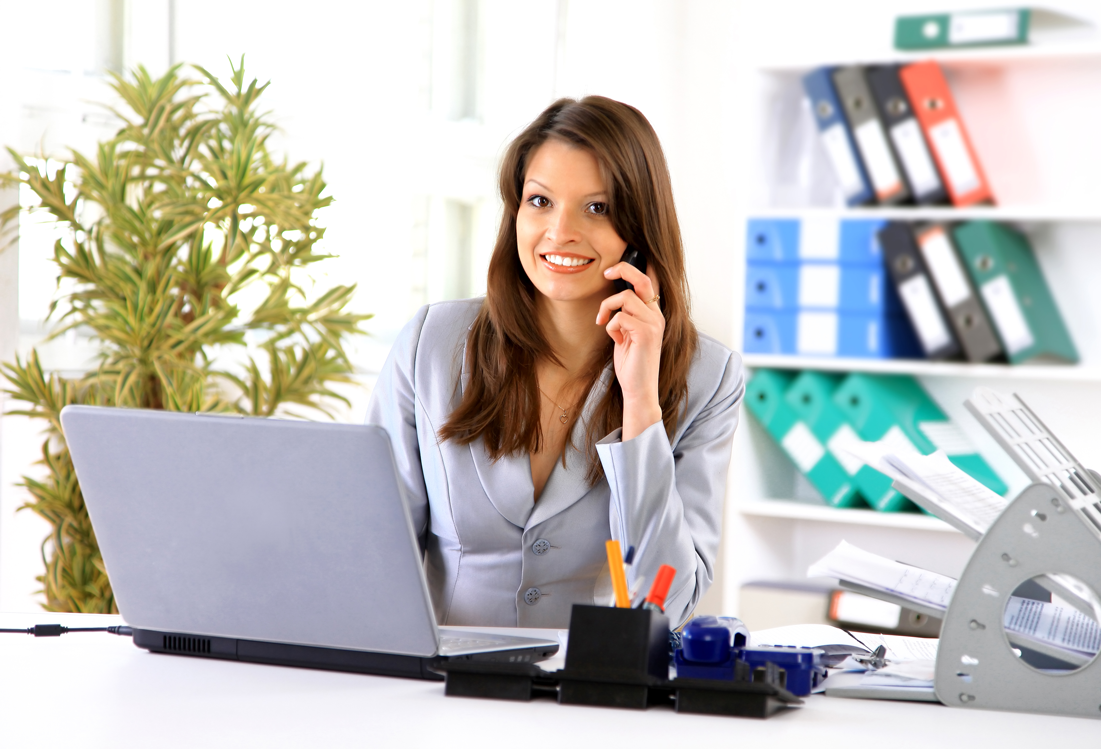
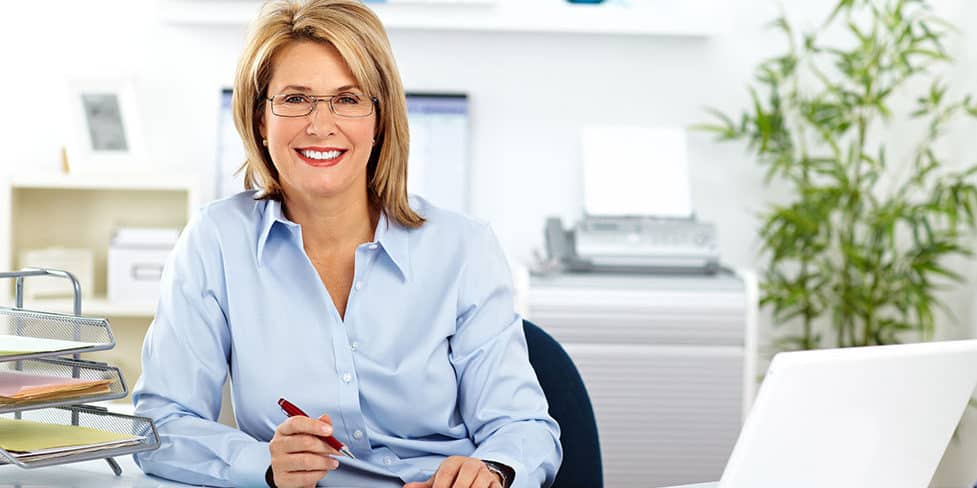
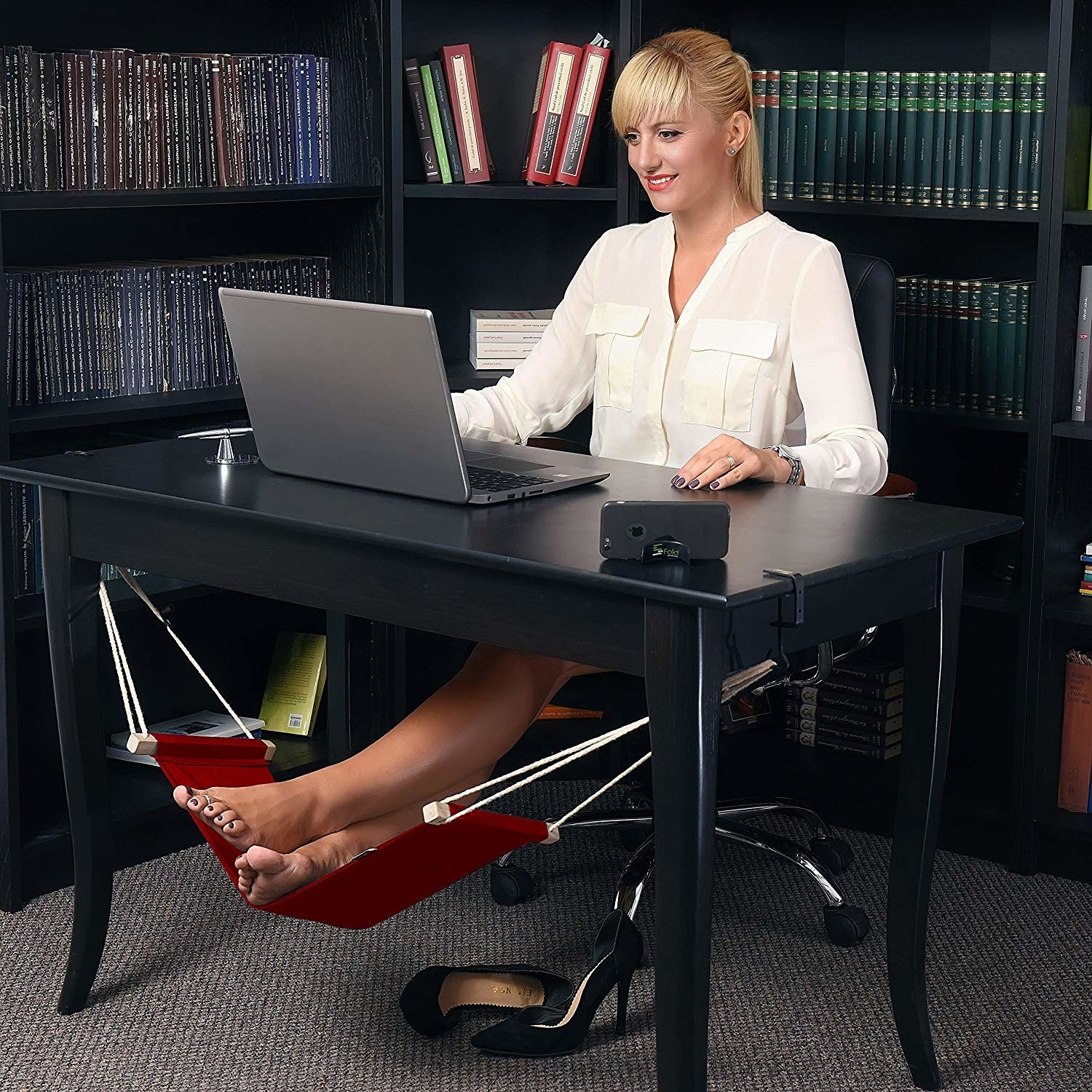

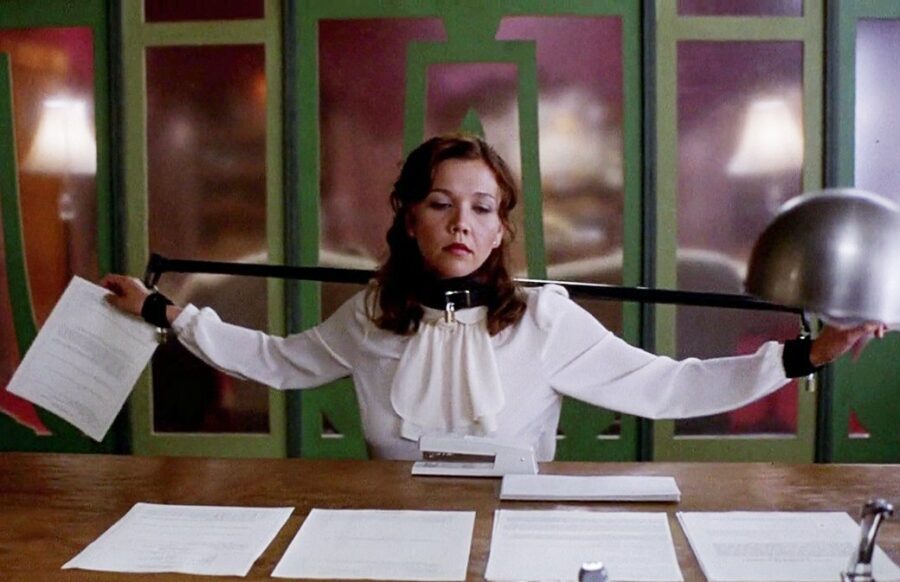
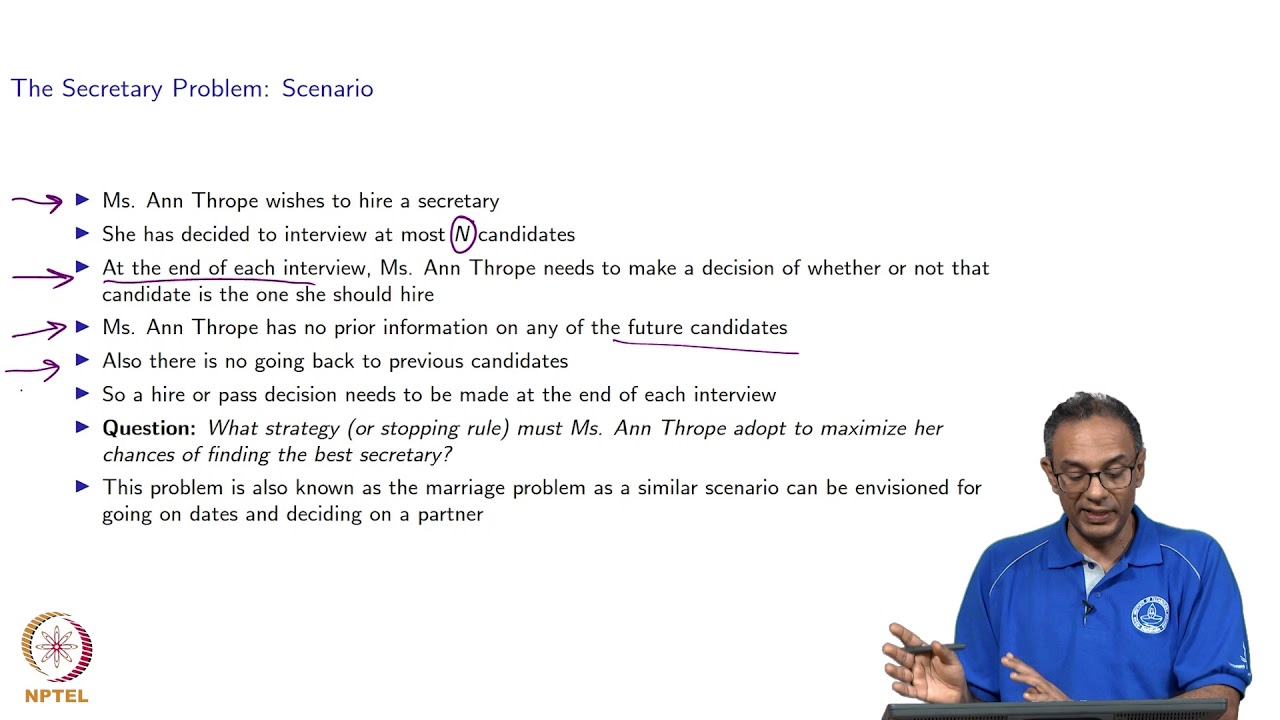
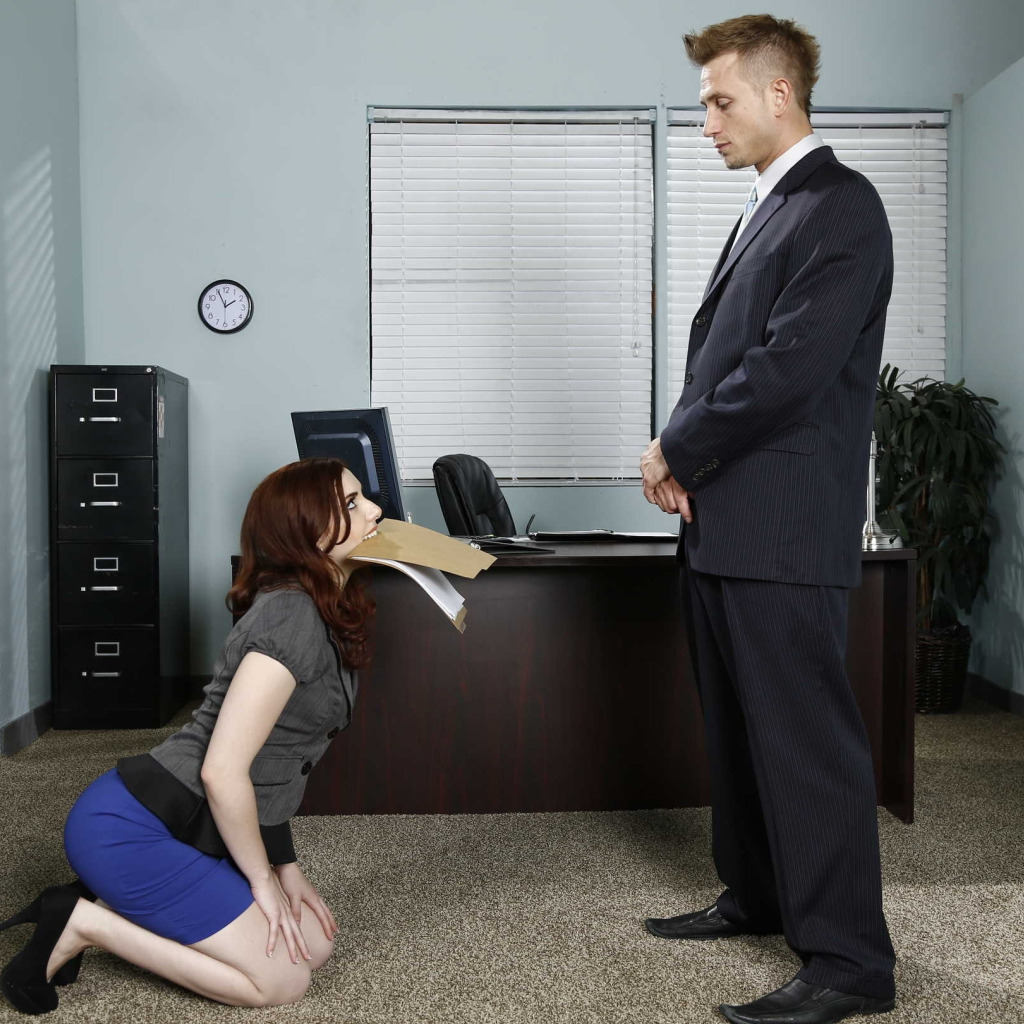

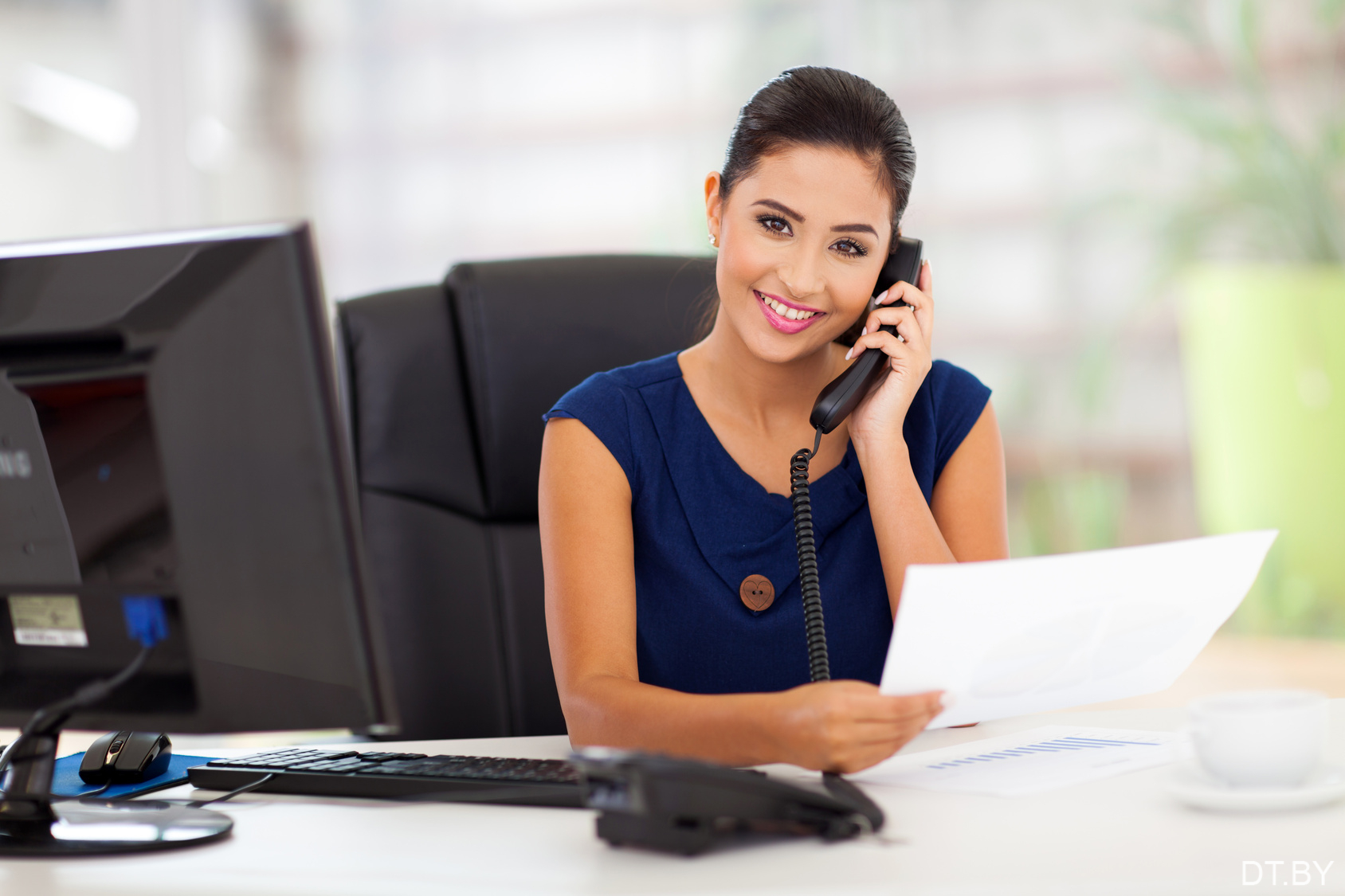
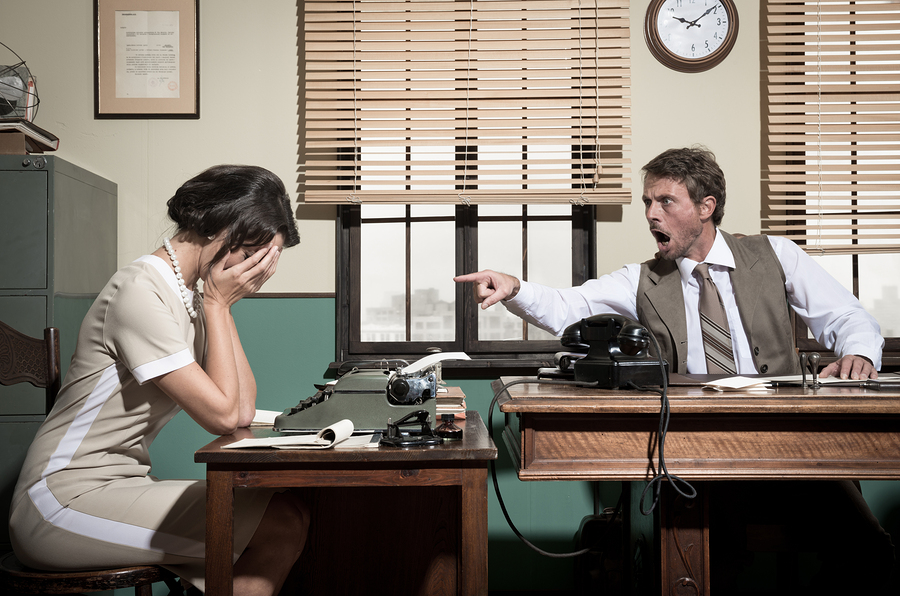
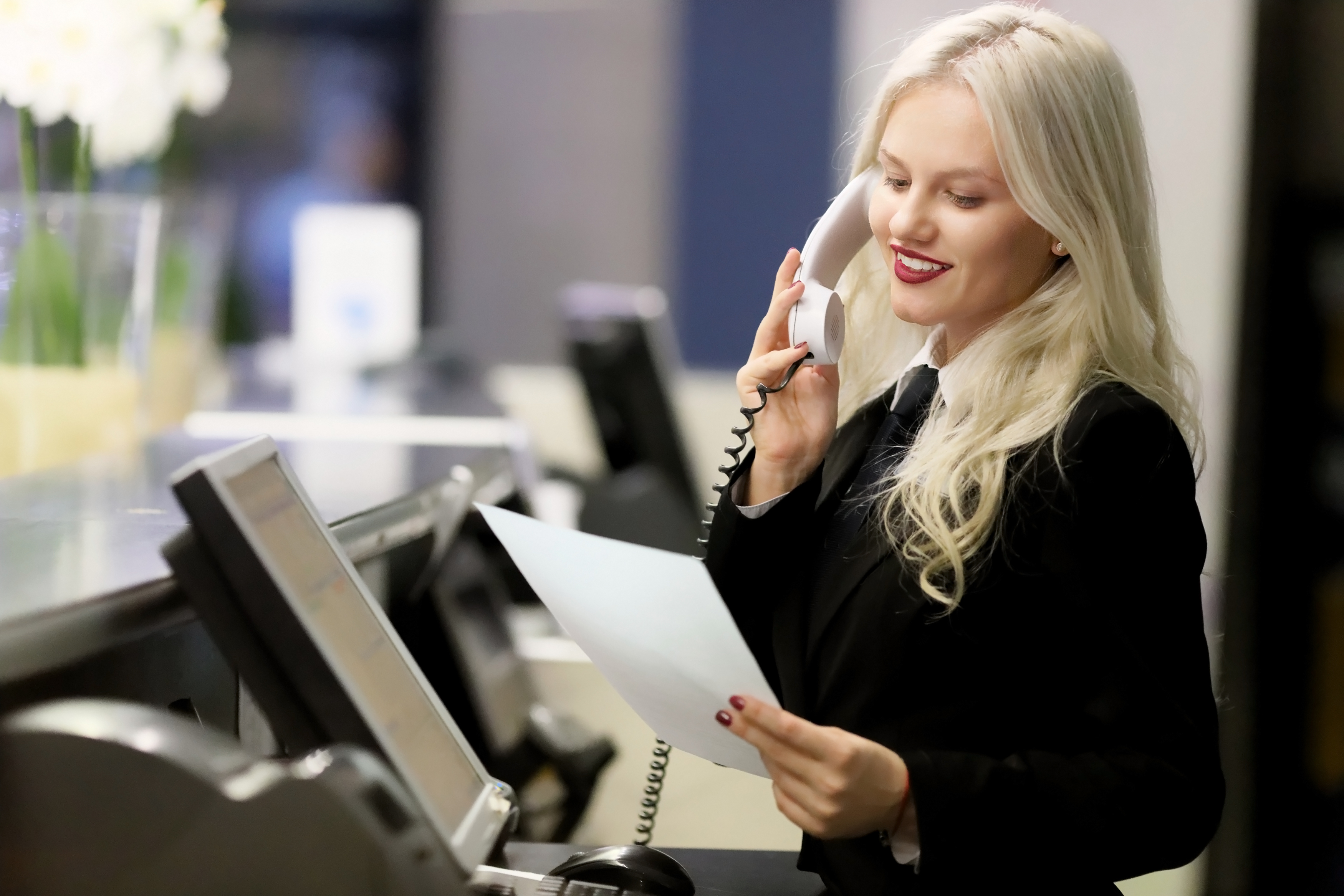
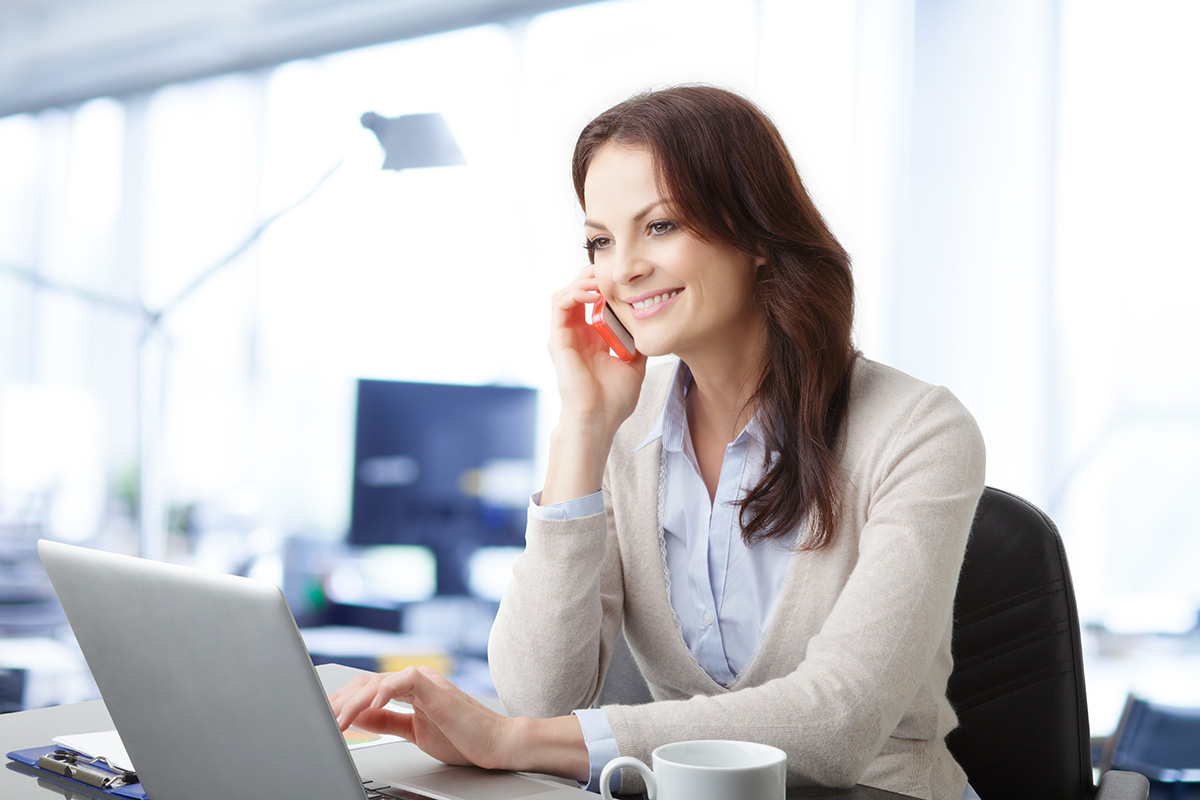
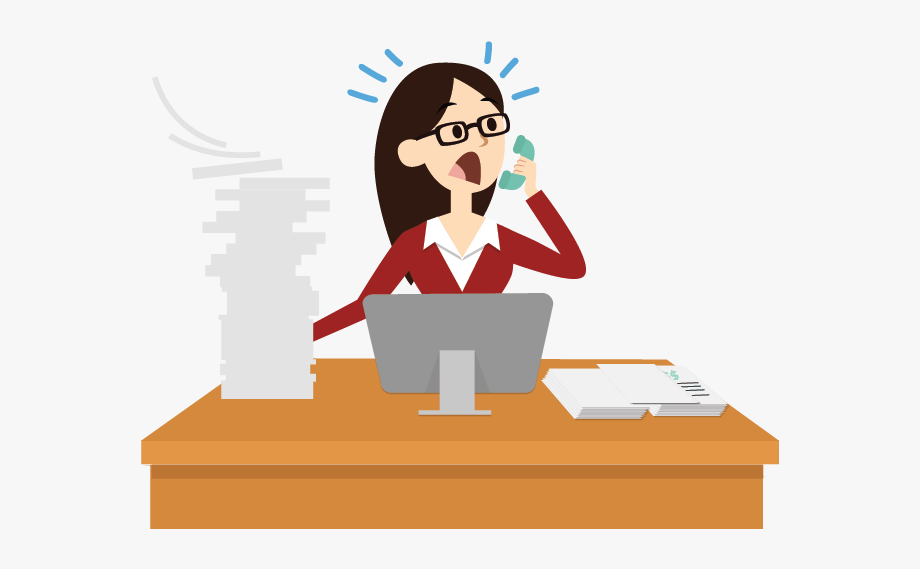
