Ncaa Mens Basketball Spreads
🛑 ALL INFORMATION CLICK HERE 👈🏻👈🏻👈🏻
Ncaa Mens Basketball Spreads
January 31, 2021 - February 6, 2021
Michigan
Roster
Statistics
Schedule
Northwestern
Roster
Statistics
Schedule
Terms of Use Privacy Policy Interest-Based Ads © 2020 ESPN Enterprises, Inc. All rights reserved.
LSU -
Shareef O'Neal Defensive Rebound.
Newton throws down fast-break dunk for Pirates
Bryant thunders down a second-chance dunk for South Carolina
2020 NCAA Division I Men 's Basketball Tournament - Wikipedia
NCAAM College Basketball Scores - NCAAM Scoreboard - ESPN
(PDF) Building an NCAA mens basketball predictive model and quantifying...
NCAA Division I Men 's Basketball Tournament - Wikipedia
Men ’s NCAA Basketball Recruiting Guidelines | Skills Needed
The old adage says that it is better to be lucky than to be good, but when it
comes to winning NCAA tournament pools, do you need to be both? This paper
attempts to answer this question using data from the 2014 men's basketball
tournament and more than 400 predictions of game outcomes submitted to a
contest hosted by the website Kaggle. We begin by describing how we built a
prediction model for men's basketball tournament outcomes under the binomial
log-likelihood loss function. Next, under different sets of true underlying
game probabilities, we simulate tournament outcomes and imputed pool standings,
in an effort to determine how much of an entry's success can be attributed to
luck. While one of our two submissions finished first in the Kaggle contest, we
estimate that this winning entry had no more than about a 12% chance of doing
so, even under the most optimistic of game probability scenarios.
Content uploaded by Gregory J. Matthews
Content may be subject to copyright.
A preview of the PDF is not available
... In their favor, the possibility to retrieve useful information from online platforms is rapidly increasing, thanks to improvements in storage capacity and computing power. In the era of Money Ball Thinking [16], scientific literature covered several aspects of research about basketball, ranging from delineating the main characteristics of a game by means of basic descriptive statistics [15] to exploring complex problems, such as forecasting the winning of a game or a tournament [3,11,18, 19, 23,34,38,39] or identifying the best game strategy [1,24]. ...
... To categorize numerical covariates, we applied TIM diagnostic. According to the results reported in Figure 4, we define the thresholds for the numerical covariates as listed below: [2,4] if +2p ≤ sc.diff ij ≤ +4p [5, 19] if +5p ≤ sc.diff ij ≤ +19p +20 or more if sc.diff ij ≥ +20p; ...
Measuring players' performance in team sports is fundamental since managers need to evaluate players with respect to the ability to score during crucial moments of the game. Using Classification and Regression Trees (CART) and play-by-play basketball data, we estimate the probabilities to score the shot with respect to a selection of game covariates related to game pressure. We use scoring probabilities to develop a player-specific shooting performance index that takes into account for the difficulty associated to score different types of shots. By applying this procedure to a large sample of 2016–2017 Basketball Champions League (BCL) and 2017–2018 National Basketball Association (NBA) games, we compare the factors affecting shooting performance in Europe and in the United States and we evaluate a selection of players in terms of the proposed shooting performance index with the final aim of providing useful guidelines for the team strategy.
... Many researchers and fans use ranking methods to complete tournament brackets [23]. While there are many aspects of the March Madness tournament that are of interest, predicting and anticipating the upsets that occur each year is of broad appeal [17, 27, 33]. For this reason, we use rankability to predict the degree to which a year will exhibit upsets. ...
... The earliest works in predicting the NCAA results (such as [26], [27]) tried to find a linear relationship between the rank differences and the probability to win a game. The winner team of the 2014's Kaggle's March Madness competition used teambased possession metrics to a logistic regression model [28] . [29] applied a classical soccer-forecasting model to make predictions for the college basketball tournament. ...
In machine learning tasks, especially in the tasks of prediction, scientists tend to rely solely on available historical data and disregard unproven insights, such as experts' opinions, polls, and betting odds. In this paper, we propose a general three-step framework for utilizing experts' insights in machine learning tasks and build four concrete models for a sports game prediction case study. For the case study, we have chosen the task of predicting NCAA Men's Basketball games, which has been the focus of a group of Kaggle competitions in recent years. Results highly suggest that the good performance and high scores of the past models are a result of chance, and not because of a good-performing and stable model. Furthermore, our proposed models can achieve more steady results with lower log loss average (best at 0.489) compared to the top solutions of the 2019 competition (>0.503), and reach the top 1%, 10% and 1% in the 2017, 2018 and 2019 leaderboards, respectively.
... We exclude important topics related to drafting players (e.g. McCann, 2003, Groothuis et al., 2007, Berri et al., 2011, Arel & Tomas III, 2012, roster construction, win probability models, tournament prediction (e.g. Brown et al., 2012, Gray & Schwertman, 2012 , Lopez & Matthews, 2015 , Yuan et al., 2015, Ruiz & Perez-Cruz, 2015, Dutta et al., 2017, Neudorfer & Rosset, 2018, and issues involving player health and fitness (e.g. Drakos et al., 2010, McCarthy et al., 2013. ...
In recent years, analytics has started to revolutionize the game of basketball: quantitative analyses of the game inform team strategy, management of player health and fitness, and how teams draft, sign, and trade players. In this review, we focus on methods for quantifying and characterizing basketball gameplay. At the team level, we discuss methods for characterizing team strategy and performance, while at the player level, we take a deep look into a myriad of tools for player evaluation. This includes metrics for overall player value, defensive ability, and shot modeling, and methods for understanding performance over multiple seasons via player production curves. We conclude with a discussion on the future of basketball analytics, and in particular highlight the need for causal inference in sports.
... Overall, the wide literature dealing with the analysis of basketball data is concerned with statistical techniques applied to investigate complex problems, with a great variety of different aims. Just to cite the most recent contributions, the main themes cover the fields of predicting the outcomes of games (Gupta 2015; Lopez and Matthews 2015; Ruiz and Perez-Cruz 2015;Yuan et al. 2015;Manner 2016;Vračar et al. 2016), identifying factors able to discriminate between successful and unsuccessful teams ( Koh et al. 2011Koh et al. , 2012García et al. 2013), investigating the patterns of scoring during the games and measuring players' shooting performance ( Gilovich et al. 1985;Fearnhead and Taylor 2011;Gabel and Redner 2012;Schwarz 2012;Özmen 2012;Avugos et al. 2013;Page et al. 2013;Erčulj and Štrumbelj 2015;Cervone et al. 2016;Deshpande and Jensen 2016;Passos et al. 2016;Franks et al. 2016;Engelmann 2017;Zuccolotto et al. 2018), analyzing playing patterns with reference to roles (Alagappan 2012;Bianchi et al. 2017), depicting the players' movements, pathways, trajectories from the in-bounds pass to the basket and the network of passing actions ( Passos et al. 2011;Lamas et al. 2011;Piette et al. 2011;Fewell et al. 2012;Shortridge et al. 2014;Ante et al. 2014;Clemente et al. 2015;Gudmundsson and Horton 2017;Metulini et al. 2017a, b;Bornn et al. 2017;Miller and Bornn 2017;Metulini et al. 2018;Wu and Bornn 2018), studying teams' tactics and optimal game strategies ( Zhang et al. 2013;Skinner and Goldman 2017), and much more. ...
Assessing the scoring probability of teams and players in different areas of a court map is an important topic in basketball analytics, in order to define both game strategies and training programmes. In this contribution we propose a spatial statistical method based on classification trees, aimed to define a partition of the court in rectangles with maximally different shooting performances. Each analyzed team/player is characterized by its/his own partition, so comparisons can be made among different teams/players. In addition, shooting efficiency measures computed within the rectangles can be used to define spatial shooting performance indicators.
... Value generating management of both structured and unstructured data in sports falls into the vast field of sport analytics. Application of predictive analytics has been used successfully on many different sports such as football [1], basketball [2, 3], cricket [4], rugby [5], and hockey [6]. Predicting outcomes of any sports game naturally is one of the most obvious objectives in sports analytics. ...
... Under different sets of true underlying game probabilities, they simulate tournament outcomes and imputed pool standings, in an effort to determine how much of an entry's success can be attributed to luck. While one of the two submissions finished first in the Kaggle contest, the researchers estimate that this winning entry had no more than about a 12% chance of doing so, even under the most optimistic of game probability scenarios [6] . ...
... This paper is concerned with basketball. Scientific literature on basketball covers several aspects of research, ranging from simply outlining the main features of a game by means of descriptive statistics (Kubatko et al. 2007) to investigating more complex problems, such as forecasting the outcome of a game or a tournament (West 2008;Loeffelholz, Bednar, and Bauer 2009;Brown et al. 2010;Gupta 2015; Lopez and Matthews 2015; Ruiz and Perez-Cruz 2015;Yuan et al. 2015;Manner 2016), analysing players' performance (Page, Fellingham, and Reese 2007;Cooper, Ruiz, and Sirvent 2009;Piette, Anand, and Zhang 2010;Fearnhead and Taylor 2011;Ozmen 2012;Page, Barney, and McGuire 2013;Deshpande and Jensen 2016), identifying optimal game strategies (Annis 2006) and describing the players' reactions to stressful moments (Crocker and Graham 1995;Zuccolotto, Manisera, and Sandri 2017). ...
Because of the advent of GPS techniques, a wide range of scientific literature on Sport Science is nowadays devoted to the analysis of players’ movement in relation to team performance in the context of big data analytics. A specific research question regards whether certain patterns of space among players affect team performance, from both an offensive and a defensive perspective. Using a time series of basketball players’ coordinates, we focus on the dynamics of the surface area of the five players on the court with a two-fold purpose: (i) to give tools allowing a detailed description and analysis of a game with respect to surface areas dynamics and (ii) to investigate its influence on the points made by both the team and the opponent. We propose a three-step procedure integrating different statistical modelling approaches. Specifically, we first employ a Markov Switching Model (MSM) to detect structural changes in the surface area. Then, we perform descriptive analyses in order to highlight associations between regimes and relevant game variables. Finally, we assess the relation between the regime probabilities and the scored points by means of Vector Auto Regressive (VAR) models. We carry out the proposed procedure using real data and, in the analyzed case studies, we find that structural changes are strongly associated to offensive and defensive game phases and that there is some association between the surface area dynamics and the points scored by the team and the opponent.
The field of sports science is highly emerging and has made the headlines in the research and development activities, with new challenges and trends in the recent past. Sports analytics is the analysis of historical big data mainly available in the form of statistics to provide a proper insight into the entire team, an individual, or even the coach. It also facilitates decision-making for both on-field and off-field performances of all the concerned. The term “sports analytics” was popularized in 2011. It has also created a platform under the confluence of many disciplines such as data mining, machine learning, big data analytics, artificial intelligence, and predictive analytics. The paper aims at analyzing the off-field behavior of players using a statistical approach. The focus of the study is to comprehend the nature of data set, explore the relation between the attributes of the data set, and create a model to understand how the data relates to the underlying population using a real world data set. It comprises motion sensor data of 19 activities among both male and female categories. The sports data set is referred from the UCI repository. It consists of motor sensor data collected for both male and female players. The data set also clearly displays the three dimensions of big data namely, volume, variety, and veracity.
Each year, millions of people fill out a bracket to predict the outcome of the popular NCAA men’s college basketball tournament, known as March Madness . In this paper we present a new methodology for team ranking and use it to predict the NCAA basketball tournament. We evaluate our model in Kaggle’s March Machine Learning Mania competition, in which contestants were required to predict the results of all possible games in the tournament. Our model combines two methods: the least squares pairwise comparison model and isotonic regression. We use existing team rankings (such as seeds, Sagarin and Pomeroy ratings) and look for a monotonic, non-linear relationship between the ranks’ differences and the probability to win a game. We use the isotonic property to get new rankings that are consistent with both the observed outcomes of past tournaments and previous knowledge about the order of the teams. In the 2016 and 2017 competitions, submissions based on our methodology consistently placed in the top 5% out of over 800 other submissions. Using simulations, we show that the suggested model is usually better than commonly used linear and logistic models that use the same variables.
December 2006 · Dynamics of Continuous, Discrete and Impulsive Systems Series A: Mathematical Analysis
In this paper, based on the FIR nonlinear adaptive filters and the deterministic and nonlinear characterization of chaotic time series, the FIR space-time neural networks nonlinear adaptive predictive filters are proposed to predict chaotic time series. The prediction model of chaotic time series is established with the FIR neural networks and the steps of the learning algorithm with the FIR ... [Show full abstract] neural networks are expressed. The prediction model and the learning algorithm are more effective and reliable than the adaptive higher-order nonlinear FIR filter. The Experimental and simulating results show that the FIR neural networks enjoy good adaptive prediction performance and can be successfully used to predict chaotic time series.
January 2014 · Lecture Notes in Electrical Engineering
The method of missile sudden fault prediction is researched. The distribution regularity of fault data is obtained by statistical inference. Furthermore, the sudden fault prediction model of missile is established to get the future sudden fault probability of missile based on the distribution function of fault data. The results of case analysis validate the rationality of sudden fault prediction ... [Show full abstract] model.
September 2009 · Journal of Power Sources
Thermal management of a solid oxide fuel cell (SOFC) stack essentially involves control of the temperature within a specific range in order to maintain good performance of the stack. In this paper, a nonlinear temperature predictive control algorithm based on an improved Takagi–Sugeon (T–S) fuzzy model is presented. The improved T–S fuzzy model can be identified by the training data and becomes a ... [Show full abstract] predictive model. The branch-and-bound method and the greedy algorithm are employed to set a discrete optimization and an initial upper boundary, respectively. Simulation results show the advantages of the model predictive control (MPC) based on the identified and improved T–S fuzzy model for an SOFC stack.
August 1996 · The Canadian Journal of Chemical Engineering
A model is developed which simulates water airlift pumps. The water flow rate can be predicted for a given airlift system, or, the required air flow can be estimated for a desired water flow rate. Predictions of the model were compared with a series of experiments performed by the Institute of Geological Research and were found to be in good agreement. The predictions were far superior to those ... [Show full abstract] of model found in the literature.
October 2011 · Guangdianzi Jiguang/Journal of Optoelectronics Laser
The waveband switching is studied in this paper. Firstly, a new multi-granular optical switching architecture is proposed. In this architecture, the waveband switching plane and the wavelength switching plane are independent. The same destination grouping is suitable for this new architecture. To increase the grooming efficiency, the traffic prediction strategy is used. The simulation results ... [Show full abstract] show that the blocking probability of the traffic grooming algorithm based on the same destination grouping can be decreased by 20% when the traffic prediction is used.
© 2008-2021 ResearchGate GmbH. All rights reserved.
Sleep Porn Gif
Nubiles Net Txxx
Overwatch Gmv
Mature Outdoor Xxx
Lola Reve Double Penetration
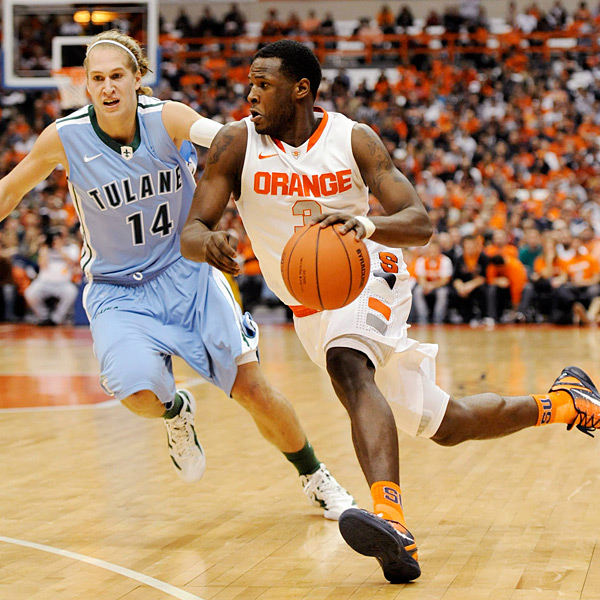
/cdn.vox-cdn.com/uploads/chorus_image/image/48596175/usa-today-9032970.0.jpg)

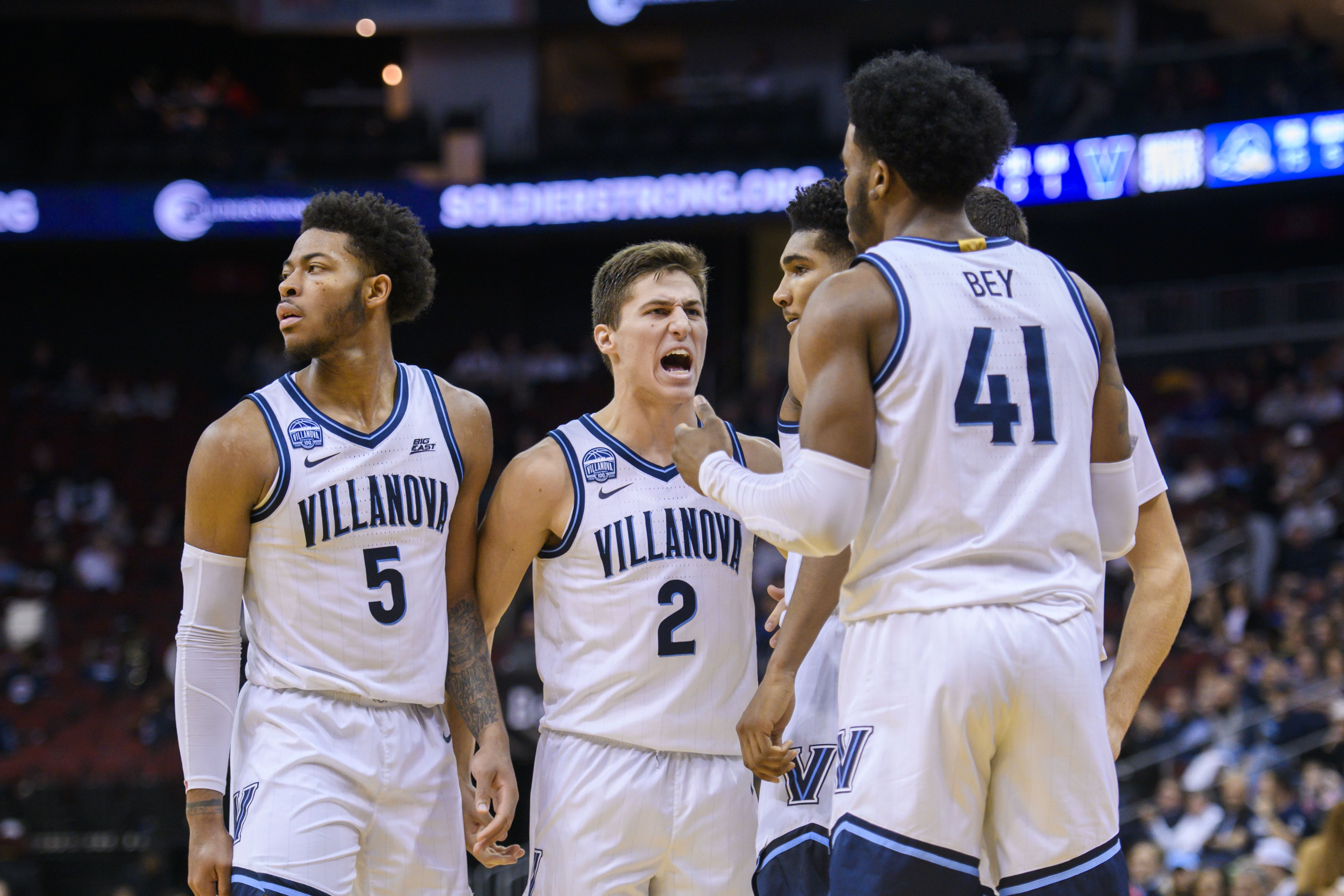





:no_upscale()/cdn.vox-cdn.com/uploads/chorus_asset/file/10320461/usa_today_10411191.jpg)


/cdn.vox-cdn.com/uploads/chorus_image/image/58515483/usa_today_10577022.0.jpg)
/cdn.vox-cdn.com/uploads/chorus_image/image/66909328/usa_today_14136536.0.jpg)

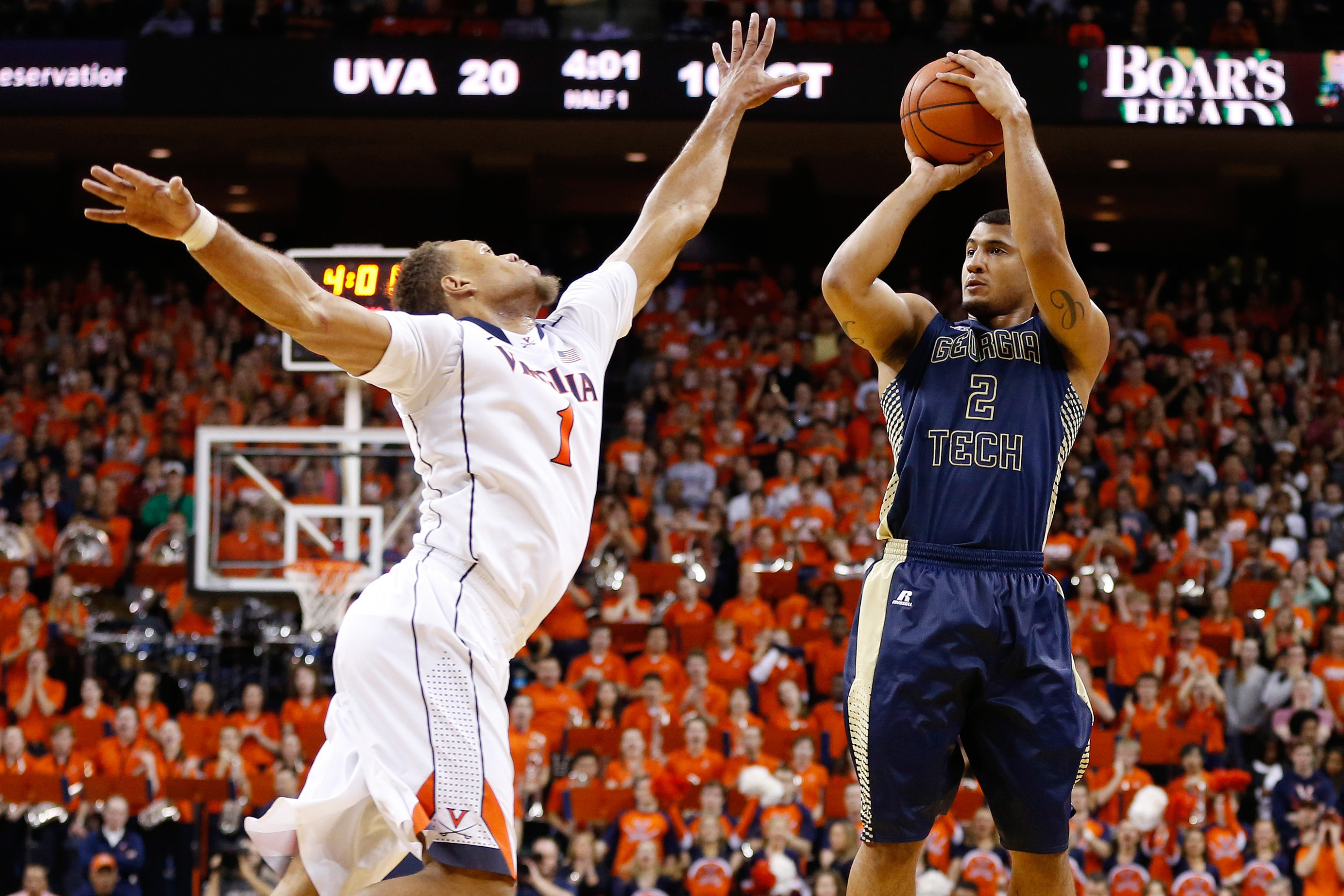
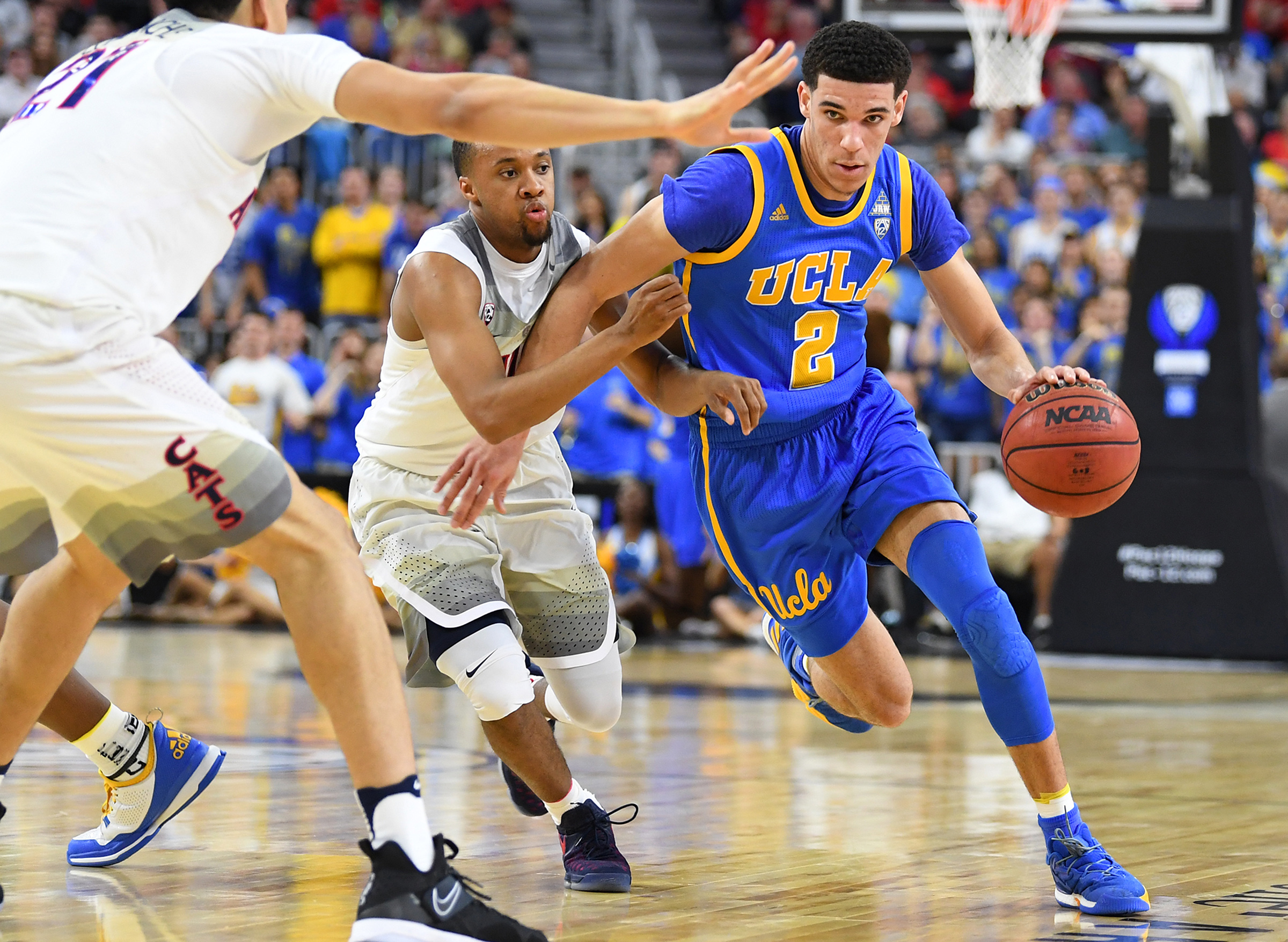

/cdn.vox-cdn.com/uploads/chorus_image/image/57558917/usa_today_9912934.0.jpg)

/cdn.vox-cdn.com/uploads/chorus_image/image/60970725/usa_today_10729597.0.jpg)
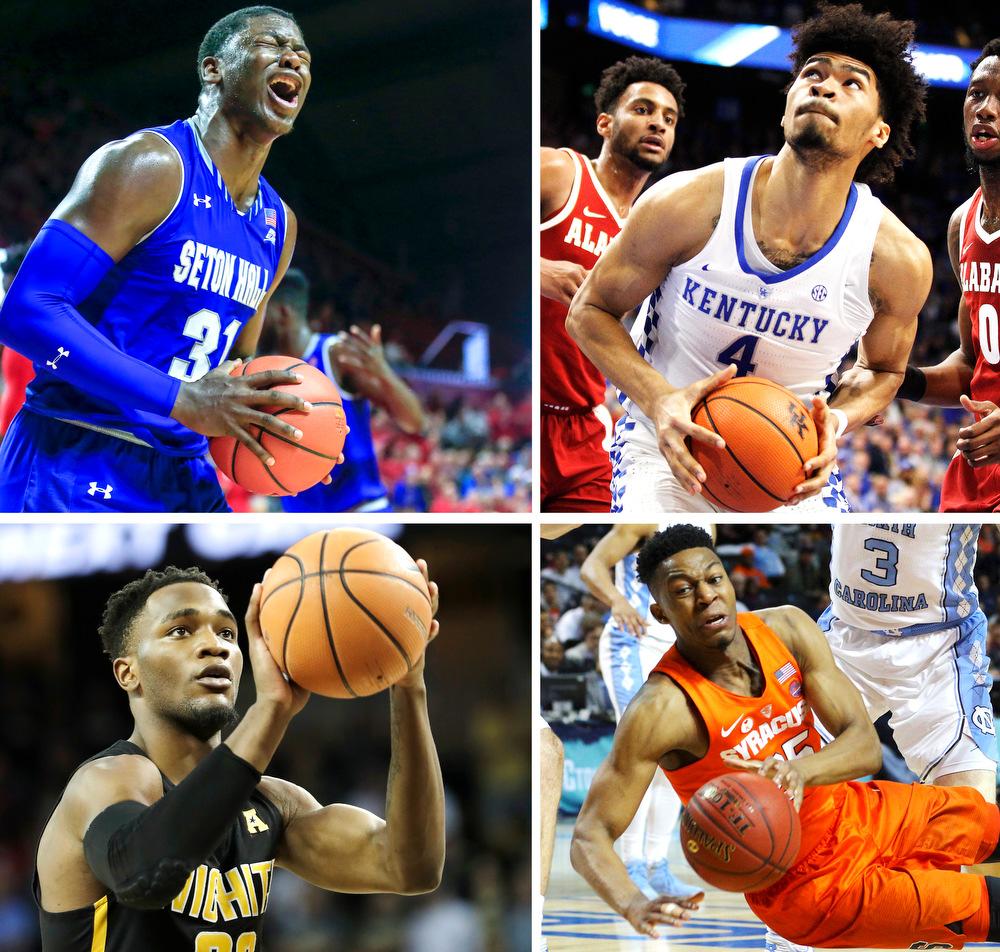
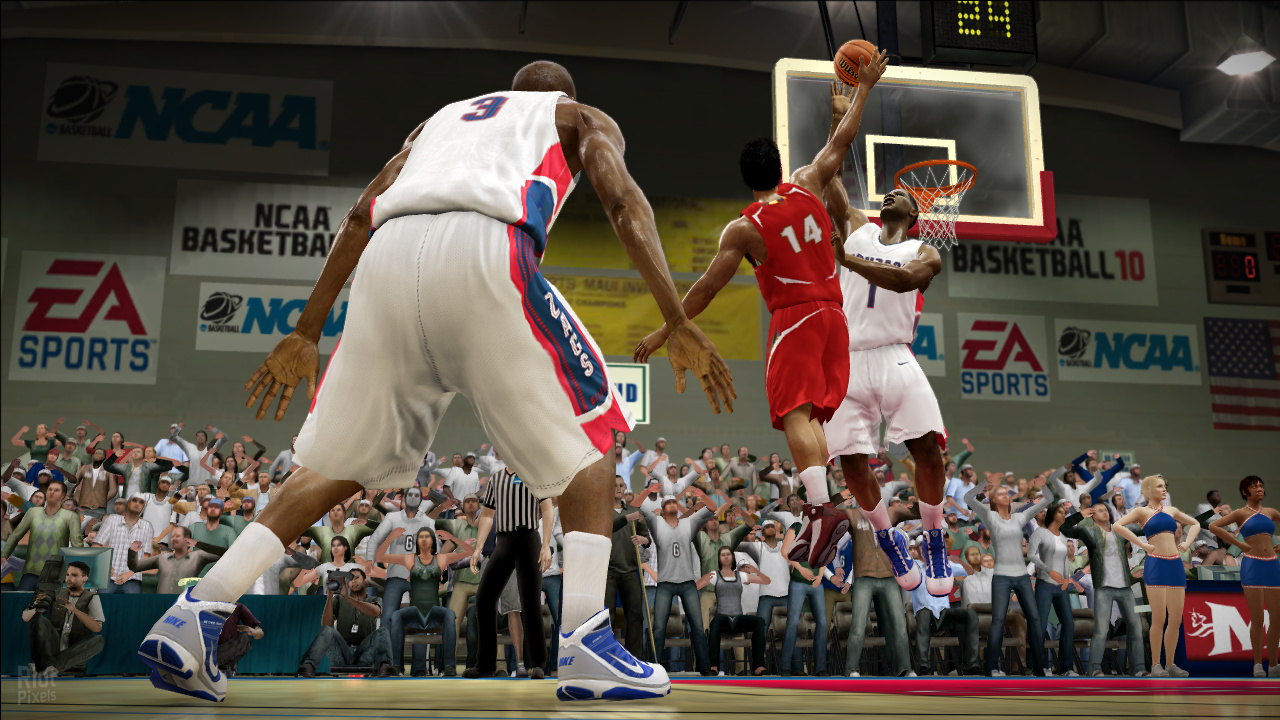




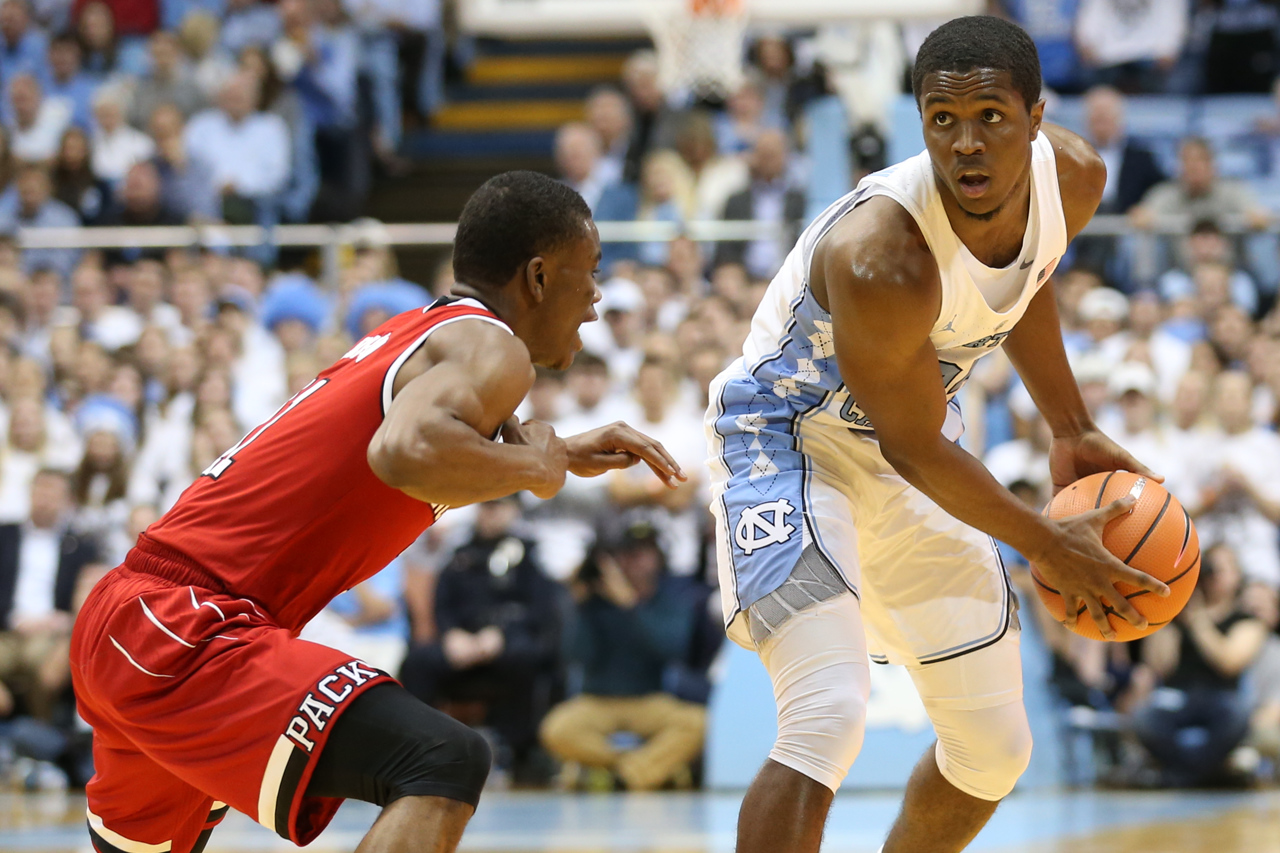



/cdn.vox-cdn.com/uploads/chorus_image/image/57396373/usa_today_9864847.0.jpg)
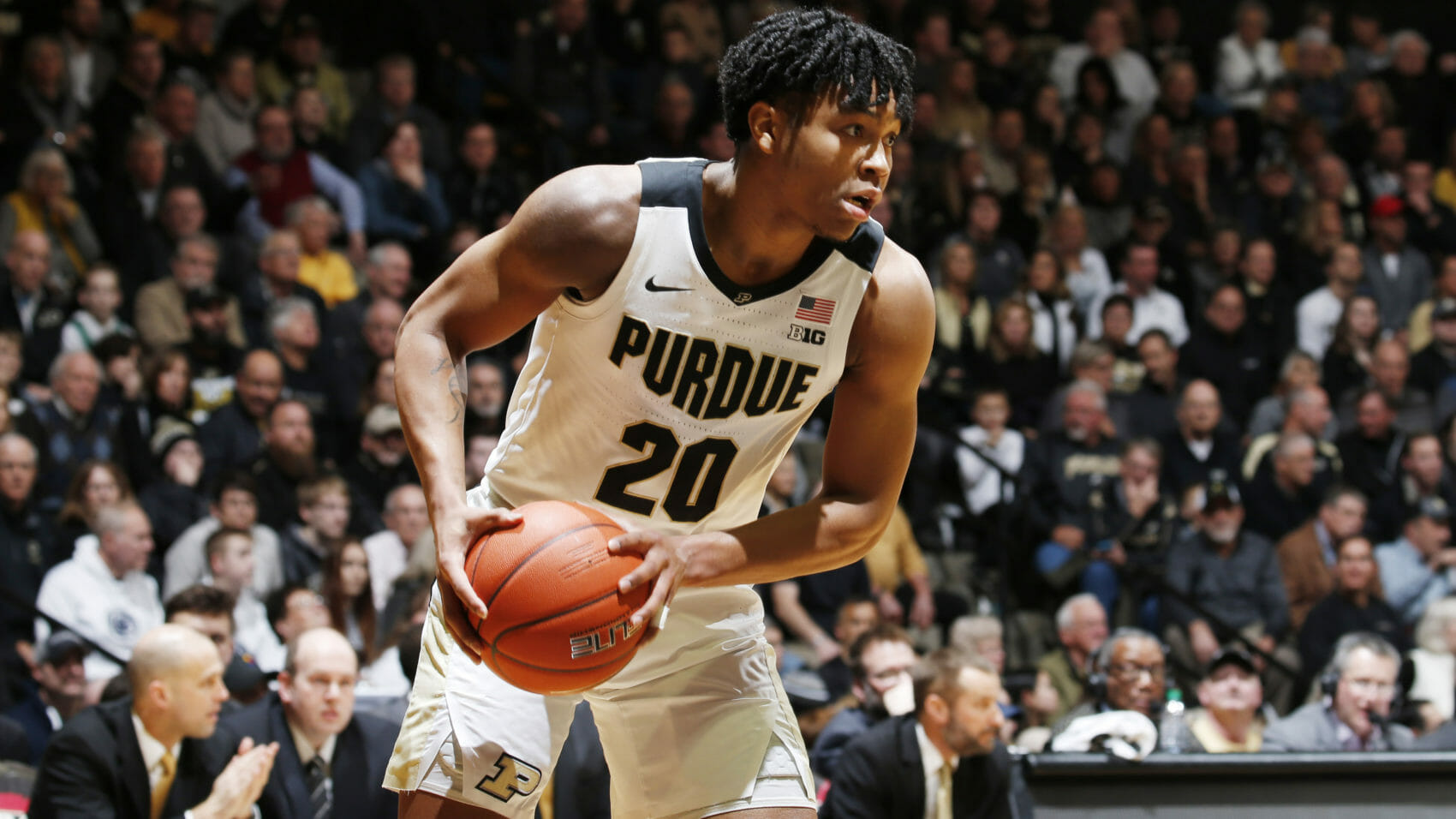
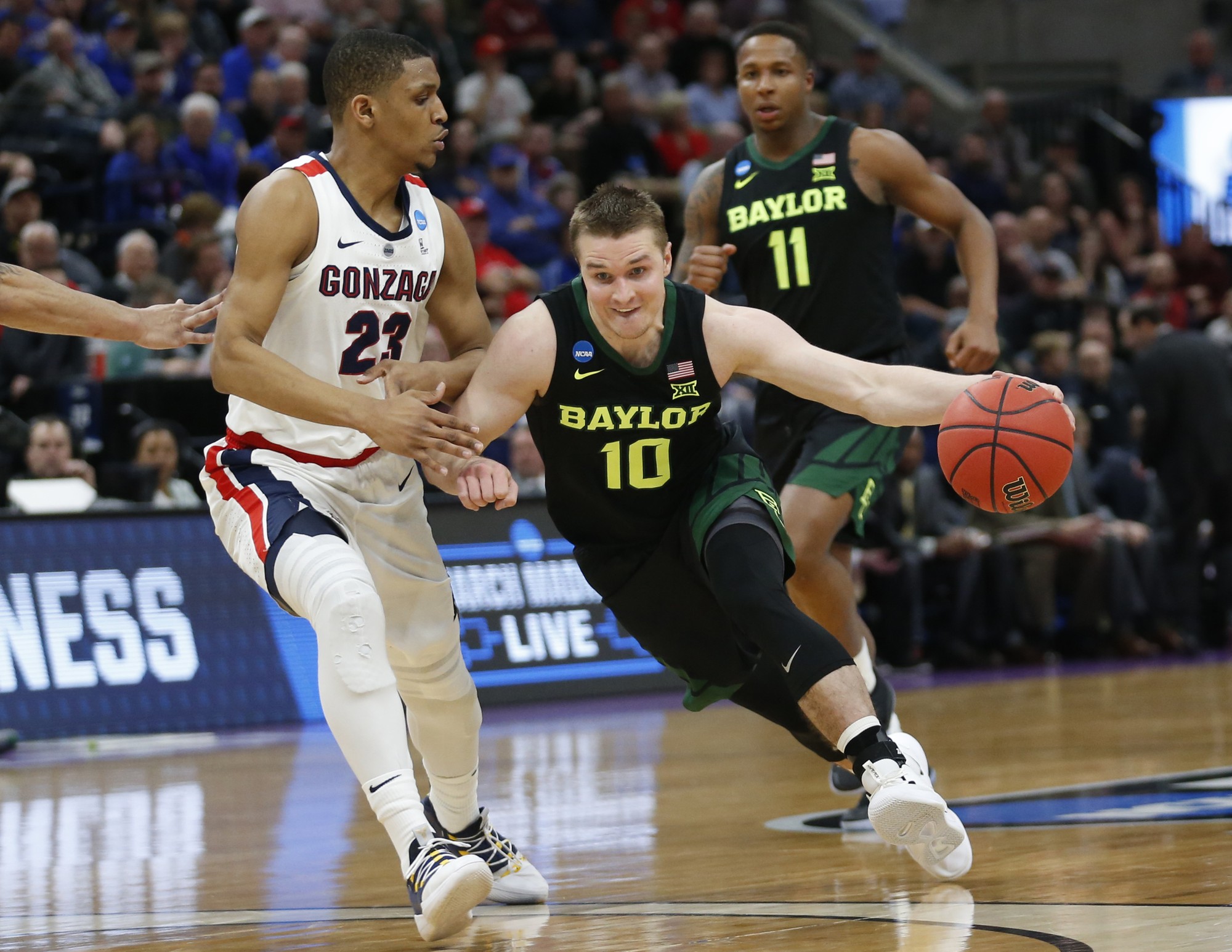
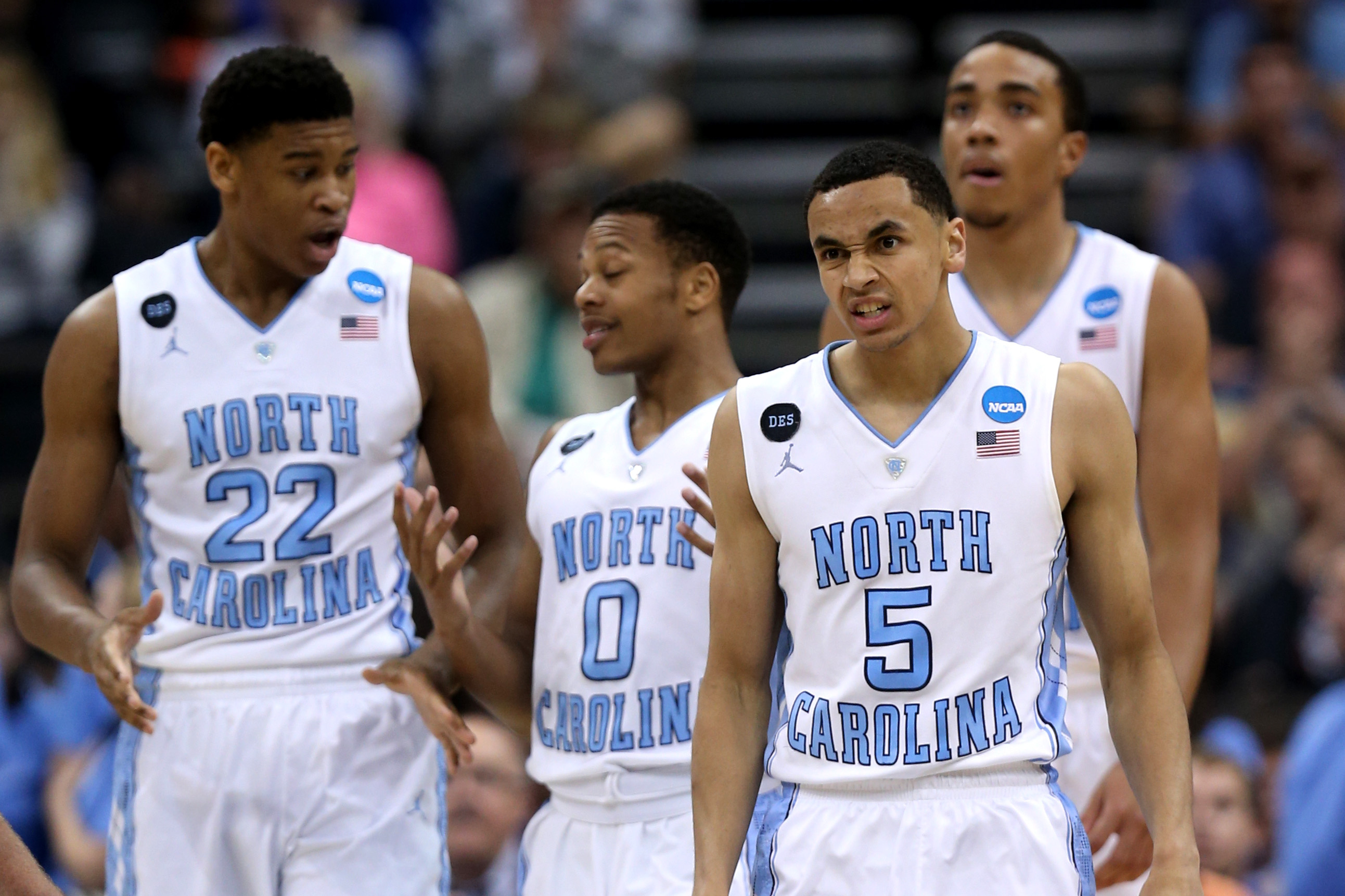

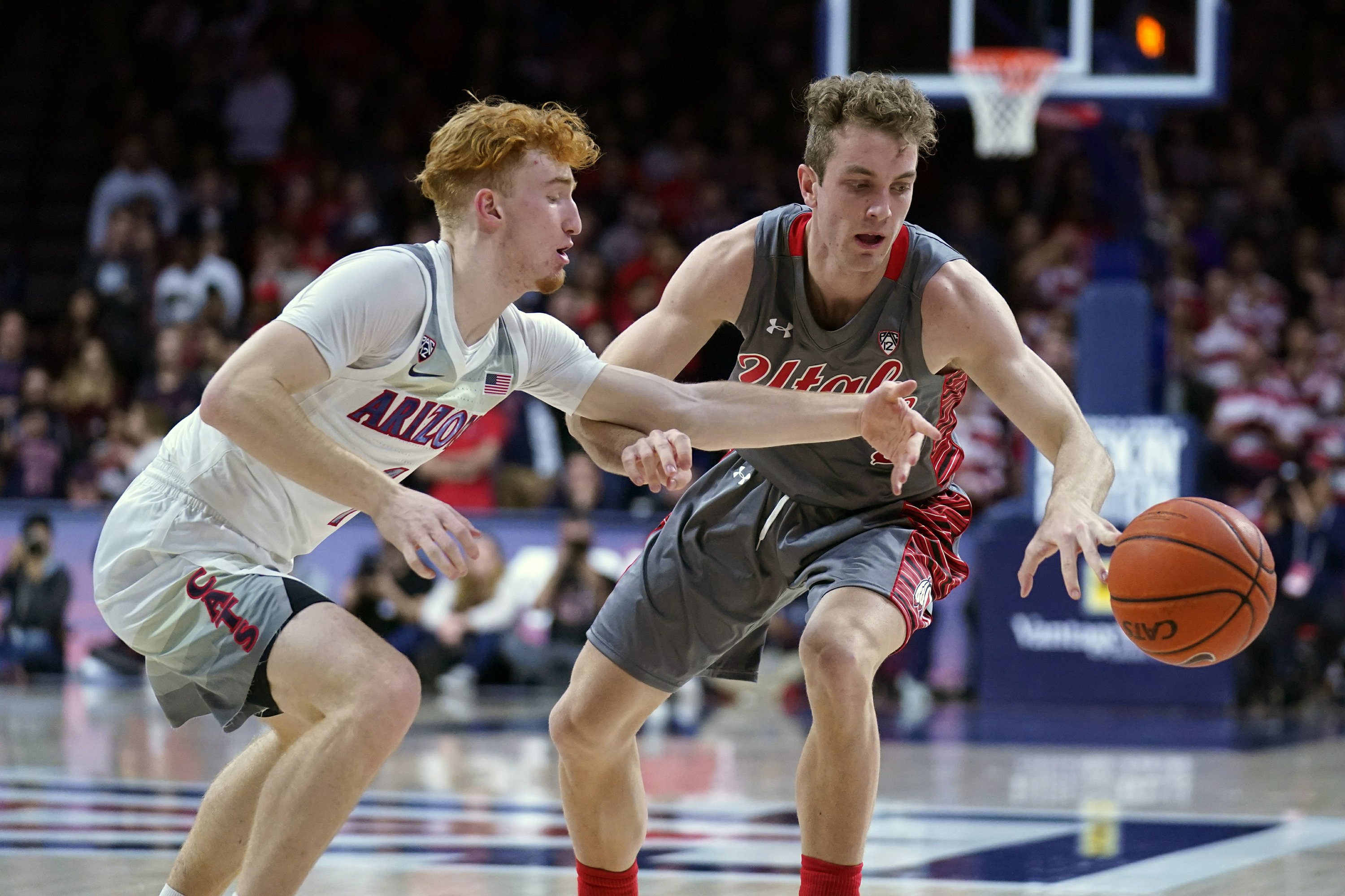