Mfc.Im
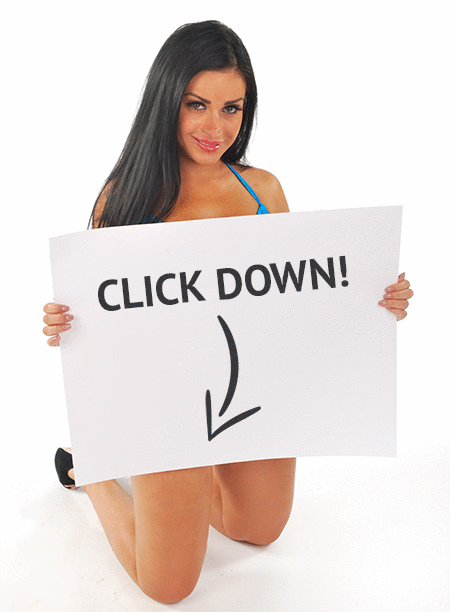
⚡ ALL INFORMATION CLICK HERE 👈🏻👈🏻👈🏻
Mfc.Im
First words from our new striker...
Almost 10,000 headed to the Riverside for the Carabao Cup tie...
Log in or sign up to watch and listen to live matches and video-on-demand
If playback doesn't begin shortly, try restarting your device.
Videos you watch may be added to the TV's watch history and influence TV recommendations. To avoid this, cancel and sign in to YouTube on your computer.
An error occurred while retrieving sharing information. Please try again later.
0:02 / 7:29 • Watch full video Live
Overview
Tasks
Data
Schedule
Leaderboard
Rules
Resources
Contact
The Open Media Forensics Challenge (OpenMFC) is a media forensics evaluation to facilitate development of systems that can automatically detect and locate manipulations in imagery (i.e., images and videos).
The NIST OpenMFC evaluation is being conducted to examine the performance of system’s accuracy and robustness over diverse datasets collected under controlled environments.
The NIST OpenMFC is open worldwide. We invite all organizations including past DARPA MediFor Program participants to submit their results using their technologies to the OpenMFC evaluation server. Participation is free. NIST does not provide funds to participants.
To take part in the OpenMFC evaluation you need to register on this website and complete the data license to download the data. Once your system is functional you will be able to upload your outputs to the challenge website and see your results displayed on the leaderboard.
OpenMFC 2022 Evaluation Plan [ Download Link ]
If you have any question, please email to the NIST MFC team:
mfc_poc@nist.gov
OpenMFC Evaluation Tentative Schedule
IMD
ISMD
VMD
IDD
VDD
StegD
Some systems on the
leaderboard are built with training data (with reference
ground-truth information known), we will report training data and
testing data performance separately in the NIST evaluation report.
We would not recommend direct comparisons.
Image Splice Manipulation Detection (ISMD)
Participation in the OpenMFC20 evaluation is voluntary and open to all who find the task of interest and are willing and able to abide by the rules of the evaluation. To fully participate a registered site must:
become familiar with, and abide by, all evaluation rules;
develop/enhance an algorithm that can process the required evaluation datasets;
submit the necessary files to NIST for scoring; and
attend the evaluation workshop (if one occurs) and openly discuss the algorithm and related research with other evaluation participants and the evaluation coordinators.
Participants are free to publish results for their own system but must not publicly compare their results with other participants (ranking, score differences, etc.) without explicit written consent from the other participants.
While participants may report their own results, participants may not make advertising claims about their standing in the evaluation, regardless of rank, or winning the evaluation, or claim NIST endorsement of their system(s). The following language in the U.S. Code of Federal Regulations (15 C.F.R. § 200.113)14 shall be respected: NIST does not approve, recommend, or endorse any proprietary product or proprietary material. No reference shall be made to NIST, or to reports or results furnished by NIST in any advertising or sales promotion which would indicate or imply that NIST approves, recommends, or endorses any proprietary product or proprietary material, or which has as its purpose an intent to cause directly or indirectly the advertised product to be used or purchased because of NIST test reports or results.
At the conclusion of the evaluation, NIST may generate a report summarizing the system results for conditions of interest. Participants may publish or otherwise disseminate these charts, unaltered and with appropriate reference to their source.
During the OpenMFC20 evaluation, a maximum of ten system slots per task can be created. There are no limits on the number of submissions per system slot.
The challenge participant can train their systems or tune parameters using any data complying with applicable laws and regulations.
The challenge participant agrees not to probe the test images/videos via manual/human means such as looking at the media to produce the manipulations from prior to the evaluation period to end of leaderboard evaluation.
All machine learning or statistical analysis algorithms must complete training, model selection, and tuning prior to running on the test data. This rule does not preclude online learning/adaptation during test data processing so long as the adaptation information is not reused for subsequent runs of the evaluation collection.
The MediScore package contains a submission checker that validates the submission in both the syntactic and semantic levels. Participants should check their submission prior to sending them to NIST. NIST will reject submissions that do not pass validation. The OpenMFC20 evaluation plan contains system output formats and instructions for how to use the validator. NIST provides the command line tools to validate OpenMFC20 submission files. Please refer to the OpenMFC20 evaluation plan for the details.
MediScore is the NIST-developed Media Forensics Challenge (MFC) scoring and evaluation toolkit. MediScore contains the source, documentation, and example data for the following tools:
Validator - Single/Double Source Detection Validator
DetectionScorer - Single/Double Source Detection Evaluation Scorer
MaskScorer - Single/Double Source Mask Evaluation (Localization) Scorer
ProvenanceFilteringScorer - Scorer for Provenance Filtering
ProvenanceGraphBuildingScorer - Scorer for Provenance Graph Building
VideoTemporalLocalisationScoer - Scorer for Video Temporal Localization
The MediScorer package git repository is available here .
OpenMFC only needs Validator, DetectionScorer, and MaskScorer.
The Journaling Tool (JT) is an automatic tool to aim data and metadata collections, annotations, and generation of automated manipulations designed according to data collection requirements. The intent of journaling is to capture a detailed history graph for each media manipulated project that results in a set of one or more final manipulated media files. The data collection process requires media manipulators to capture the detailed steps of manipulations during the manipulation process. In order to reduce the burden on human manipulators, automation is built into the capture process to record incremental changes via mask generation and change analysis. Please refer to the paper [citation-Manipulation Data Collection and Annotation Tool for Media Forensics] for the details.
You can download the Journaling Tool package from Github .
Site Privacy
Accessibility
Privacy Program
Copyrights
Vulnerability Disclosure
No Fear Act Policy
FOIA
Environmental Policy
Scientific Integrity
Information Quality Standards
Commerce.gov
Science.gov
USA.gov
Vote.gov
In general, there are multiple tasks in media forensic applications. For example, manipulation detection and localization, Generative Adversarial Network (GAN) detection, image splice detection and localization, event verification, camera verification, and provenance history analysis etc.
The OpenMFC initially focuses on manipulation detection and deepfake tasks. In future, challenges may be expanded with community interest.
The OpenMFC 2022 has following three task categories: Manipulation Detection (MD), Deepfakes Detection (DD), and Steganography Detection (StegD).
A brief summary of each category and their tasks are described below. In the summary, the evaluation media is described in the following way: A ‘base’ indicates original media with high provenance, while a ‘probe’ indicates a test media. A ‘donor’ indicates another media whose content was donated into the base media and generated the probe media. For a full description of the evaluation tasks, please refer to the OpenMFC 2022 Evaluation Plan [ Download Link ].
The objective for Manipulation Detection (MD) is to detect if a probe has been manipulated, and if so, to spatially localize the edits. Manipulation in this context is defined as deliberate modifications of media (e.g., splicing and cloning etc.) and localization is encouraged but not required for OpenMFC.
The MD task includes three tasks, namely,
The Image Manipulation Detection task is to detect if the image has been manipulated, and then to spatially localize the manipulated region. For detection, the IMD system provides a confidence score for all probe (i.e., a test image) with higher numbers indicating the image is more likely to have been manipulated. The target probes (i.e., probes that should be detected as manipulated) included potentially any image manipulations while the non-target probes (i.e., probes not containing image manipulations) include only high provenance images that are known to be original. Systems are required to process and report a confidence score for every probe.
For the localization part of the task, the system provides an image bit-plane mask (either binary or greyscale) that indicates the manipulated pixels. Only local manipulations (e.g., clone) require a mask output while global manipulations (e.g., blur) affecting the entire image do not require a mask.
The new task, Image Splice Manipulation Detection , is added in the OpenMFC 2022 to support entry-level public participants. The ISMD is designed for 'splice' manipulation operation only. The testing dataset is a small-size dataset (2K images), which contains either original images without any manipulation, or spliced images. The ISMD task will detect if a probe image has been spliced.
The Video Manipulation Detection (VMD) task is to detect if the video has been manipulated. In this task, the localization of spatial/temporal-spatial manipulated regions is not addressed. For detection, the VMD system provides a confidence score for all probes (i.e, a test video) with higher numbers indicating the video is more likely to have been manipulated. For VMD, target probes (i.e., probes that should be detected as manipulated) included potentially any video manipulations while the non-target probes (i.e., probes not containing video manipulations) include only high provenance videos that are known to be original. Systems are required to process and report a confidence score for every probe.
With recent advances in DeepFakes techniques and GAN (Generative Adversarial Network), imagery producers are able to generate realistic fake objects in media. The objective for Deepfakes Detection (DD) is to detect if a probe has been Deepfakes or GAN manipulated.
The DD task includes two tasks based on testing media type, namely,
All probes must be processed independently of each other within a given task and across all tasks, meaning content extracted from probe data must not affect another probe.
For the OpenMFC 2022 evaluation, all tasks should run under the following conditions:
For the image tasks, the system is only allowed to use the pixel-based content for images as input to the system. No image header or other information should be used.
For the video tasks, the system is only allowed to use the pixel-based content for videos and audio (if audio exists) as input. No video header or other information should be used.
For detection performance assessment, system performance is measured by Area Under Curve (AUC) which is the primary metric and the Correct Detection Rate at a False Alarm Rate of 5% (CDR@FAR = 0.05) from the Receiver Operating Characteristic (ROC) as shown Figure (a) below. This applies to both image and video tasks.
For the image localization performance assessment, the Optimum Matthews Correlation Coefficient (MCC) is the primary metric. The optimum MCC is calculated using an ideal mask-specific threshold found by computing metric scores over all pixel thresholds. Figure (b) below shows a visualization of the different mask regions used for mask image evaluations.
Figure 1. Detection System Performance Metrics: Receiver Operating Characteristic (ROC) and Area Under the Curve (AUC)
Figure 2. Localization System Performance Metrics: Optimum Matthews Correlation Coefficient (MCC)
Figure 3. An Example of Localization System Evaluation Report
Registered participants will get access to datasets created by the DARPA Media Forensics (MediFor) Program [ Website Link ]. During the registration process, registrants will get the data access credentials.
There will be both development data sets (those which include reference material) and evaluation data sets (which consist of only probe images to test systems). Each data set is structured similarly as described on the “MFC Data Set Structure Summary” section below.
NIST OpenMFC dataset is designed and used for NIST OpenMFC evaluation. The datasets include the following items:
The index files are pipe-separated CSV formatted files. The index file for the Manipulation task will have the columns:
Documenting each system is vital to interpreting evaluation results. As such, each submitted system, determined by unique submission identifiers, must be accompanied by Submission Identifier(s), System Description, OptOut Criteria, System Hardware Description and Runtime Computation, Training Data and Knowledge Sources, and References.
Using the (a team-defined label for the system submission, witout spaces or special characters), all system output submissions must be formatted according to the following directory structure:
/
.txt The system description file, described in Appendix A-a
.csv The system output file
/mask The system output mask directory
{MaskFileName1}.png The system output mask file directory
As an example, if the team is submitting baseline_3, their directory would be:
baseline_3/
baseline_3.txt
baseline_3.csv
/mask
Next, build a zip or tar file of your submission and post the file on a web-accessible URL that does not require user/password credentials.
Make your submission using the OpenMFC Web site. To so, follow these steps:
OpenMFC 2022 participant pre-challenge phase (QC testing)
OpenMFC STEG challenge dataset available
OpenMFC 2022 Leaderboard open for the next evaluation cycle
(New) OpenMFC dataset resource website
OpenMFC2021 Workshop Talks and Slides available
OpenMFC/TRECVID 2021 Virtual Workshop
OpenMFC 2021 Virtual Workshop agenda finalization
OpenMFC 2020-2021 submission deadline
OpenMFC 2020-2021 participant pre-challenge phase (QC testing)
Participant dry-run submission
NIST leaderboard testing/validation result
OpenMFC evaluation GAN image and video dataset available
OpenMFC evaluation image and video dataset available
OpenMFC development datasets resource available
This can take up to 60 seconds. Please wait...
• Mfc.im ranks 16,268,117 globally on Alexa.
• 2.0E-6% of global Internet users visit Mfc.im
• Mfc.im receives approximately 99 visitors and 99 page impressions per day.
• Mfc.im should earn about $0.40 /day from advertising revenue.
• Estimated value of Mfc.im is $339.48 .
• Mfc.im resolves to the IP addresses 204.15.8.13 .
• Mfc.im has servers located in Renton, WA, 98059, United States .
Title: MyFreeCams.com - The #1 *
Myfreeblack Videos
Fox Hentai
Raven Branwen Porn