Latin Square
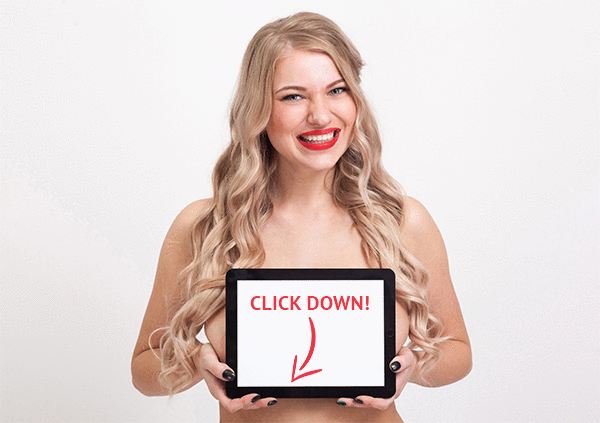
⚡ ALL INFORMATION CLICK HERE 👈🏻👈🏻👈🏻
Latin Square
URL: https://www.sciencedirect.com/science/article/pii/B9780444636881000124
Day 1 A B C Day 2 C A B Day 3 B C A
URL: https://www.sciencedirect.com/science/article/pii/B0123693977000790
URL: https://www.sciencedirect.com/science/article/pii/B9780124157590000303
URL: https://www.sciencedirect.com/science/article/pii/B9780123302151500168
URL: https://www.sciencedirect.com/science/article/pii/B9780128023877000263
URL: https://www.sciencedirect.com/science/article/pii/B9780123864543009301
URL: https://www.sciencedirect.com/science/article/pii/S1871512520300170
URL: https://www.sciencedirect.com/science/article/pii/B9780123756749100072
URL: https://www.sciencedirect.com/science/article/pii/B9780444899491500146
URL: https://www.sciencedirect.com/science/article/pii/B0080430767004174
A latin square is a design in which each treatment is assigned to each time period the same number of times and to each subject the same number of times (see Dean and Voss 1999, chap.
The Latin square design is a general version of the dye-swapping design for samples from more than two biological conditions. The Latin square design requires that the number of experimental conditions equals the number of different labels. The same number of experimental runs as the number of treatment conditions is also used. The treatment conditions are labeled once using each label and sampled once under each experimental run. Fig. 12.1B shows one way of experiment layout when Latin square design is used for three-label experiments studying the protein expressions under three biological conditions. The advantage of the Latin square design is to control the variation from different labels and different experimental runs. The Latin square also provides better efficiency than the RCBD [5] .
A Latin square is a block design with the arrangement of v Latin letters into a v × v array (a table with v rows and v columns). Latin square designs are often used in experiments where subjects are allocated treatments over a given time period where time is thought to have a major effect on the experimental response. Suppose the treatments are labeled A, B, and C. In this particular situation, the design would be
This type of design allows the separation of an additional factor from an equal number of blocks and treatments. If there are more than three blocks and treatments, then a number of Latin square designs are possible. It can be noted that Latin square designs are equivalent to specific fractional factorial designs (e.g., the 4×4 Latin square design is equivalent to a 4 3−1 fractional factorial design).
The Latin Square Design is the second experimental design that addresses sources of systematic variation other than the intended treatment. It assumes that one can characterize treatments, whether intended or otherwise, as belonging clearly to separate sets. These categories are arranged into two sets of rows. An example would be a developmental toxicity study in which the rows give the source litter of the test animal (with the first litter as row 1, the next as row 2, etc.), while the columns define a secondary category like the ages of the test animals (with 6–8 weeks as column 1, 8–10 weeks as column 2, and so on). Experimental units are then assigned so that each major treatment, such as control, low dose, intermediate dose, etc., appears once and only once in each row and each column. If one denotes test groups as A (control), B (low), C (intermediate) and D (high), such an assignment would resemble that shown in Table 30.10 . Here it can be seen that randomized experimental units are assigned to a treatment group, and then randomly assigned to a place in the Latin square.
TABLE 30.10 . Diagrammatic Representation of Treatment Allocation in a Latin Square Design
Shayne C. Gad , Colin G. Rousseaux , in Handbook of Toxicologic Pathology (Second Edition) , 2002
The Latin square design is the second experimental design that addresses sources of systematic variation other than the intended treatment. It assumes that one can characterize treatments, whether intended or otherwise, as belonging clearly to separate sets. These categories are arranged into two sets of rows, e.g., source litter of test animal, with the first litter as row 1, the next as row 2, etc. The secondary set of categories is arranged as columns, e.g., ages of test animals, with 6 to 8 weeks as column 1, 8, to 10 weeks as column 2, and so on. Experimental units are then assigned so that each major treatment, such as control, low dose, and intermediate dose, appears once and only once in each row and each column. If one denotes test groups as A (control), B (low), C (intermediate), and D (high), such as assignment would appear as in Table VIII , where it can be seen that randomized experimental units are assigned to a treatment group and then assigned randomly to a place in the Latin square.
TABLE VIII . Diagrammatic Representation of Treatment Allocation in a Latin Square Design
In the study on the effect of a xenobiotic on development given in Table VIII , treatment, age grouping, and litter sources of variation have been addressed in the design.
More than two factors can be analyzed by using Latin squares . The name (based on the name of an early mathematical puzzle) was introduced into statistical analysis for use in agricultural experiments. To allow for possible underlying differences in soil fertility, the plot of land is divided into smaller blocks to which seeds and treatments (fertilizers) are allocated at random, but in such a way that each treatment occurs once in each row and each column, and each seed occurs once in each row and each column. This principle can be applied to biological experiments as well. In an experiment with testicular spreading factor (now known to be hyaluronidase), Bacharach et al. (1940) injected it subcutaneously in six different sites on each of six rabbits. This example appears in the book by Finney ( Finney, 1955 ). The study was done so that each site and each rabbit had the injection in a different order. As a result, each site (symbolized by A to F) occurred once with each rabbit and once with each order of injection, each rabbit occurred once with each order and each site; and each order occurred once with each rabbit and each site. (Individual data are in the original publication.) Then a three-factor ANOVA was performed ( Table 26.7 ).
There are three factors that contribute to the total sums of squares: order, animal, and site. Adding up their contributions and subtracting that sum from the total produces a residual error independent of site, order, or animal. The contribution of each factor is compared with the residual mean square to provide an F ratio. In Table 26.7 , there are significant differences among animals, with rabbit number 2 having the largest bleb and thus the slowest fluid absorption. Neither site nor order had any major effect, so that in planning future experiments site and order can be ignored, but precautions are needed to allow for interanimal variability.
It is possible to do an ANOVA with four factors, by allocating the fourth factor (symbolized by Greek letters) once to each of the other three factors. This is known as a Graeco-Latin square, and is analyzed in similar fashion. Latin squares must have the same number of cells for each factor. The requirements for ratio numbers, normality, and homogeneity of variance apply equally to these more complex ANOVA.
There are four basic experimental design types used in toxicology. These are the randomized block, latin square , factorial design, and nested design. Other designs that are used are really combinations of these basic designs, and are very rarely employed in toxicology. Before examining these four basic types, however, we must first examine the basic concept of blocking.
Blocking is, simply put, the arrangement or sorting of the members of a population (such as all of an available group of test animals) into groups based on certain characteristics that may (but are not sure to) alter an experimental outcome. Such characteristics, which may cause a treatment to give a differential effect, include genetic background, age, sex, overall activity levels, and so on. The process of blocking then acts (or attempts to act) so that each experimental group (or block) is assigned its fair share of the members of each of these subgroups.
Remember that randomization is aimed at spreading out the effect of undetectable or unsuspected characteristics in a population of animals or some portion of this population. The merging of the two concepts of randomization and blocking leads to the first basic experimental design, the randomized block. This type of design requires that each treatment group have at least one member of each recognized group (such as age), the exact members of each block being assigned in an unbiased (or random) fashion.
The second type of experimental design assumes that it is possible to characterize treatments (whether intended or otherwise) as belonging clearly to separate sets. In the simplest case, these categories are arranged into two sets that may be thought of as rows (for, say, source litter of test animal, with the first litter as row 1, the next as row 2, etc.) and the secondary set of categories as columns (for, say, ages of test animals, with 6–8 weeks as column 1, 8–10 weeks as column 2, and so on). Experimental units are then assigned so that each major treatment (control, low dose, intermediate dose, etc.) appears once and only once in each row and each column. If the test groups are denoted as A (control), B (low), C (intermediate), and D (high), such an assignment would appear as shown in Table 4 .
Table 4 . Group assignments for sample experimental design
The third type of experimental design is the factorial design, in which there are two or more clearly understood treatments, such as exposure level to test chemical, animal age, or temperature. The classical approach to this situation (and to that described under the latin square) is to hold all but one of the treatments constant, and at any one time to vary just that one factor. Instead, in the factorial design, all levels of a given factor are combined with all levels of every other factor in the experiment. When a change in one factor produces a different change in the response variable at one level of a factor than at other levels of this factor, there is an interaction between these two factors, which can then be analyzed as an interaction effect.
The last of the major varieties of experimental design are the nested designs, where the levels of one factor are nested within (or are subsamples of) another factor. That is, each subfactor is evaluated only within the limits of its single larger factor.
Assay design that can be applied for parallel-methods are: Completely randomized design, Randomized block design, Latin square design and Cross-over design. The requirements for validity test using this parallel method are: (1) linier regression term is significant ( P < 0.05), (2) the term of non-parallelism is not significant ( P > 0.05), and (3) the term of non-linearity is not significant ( P > 0.05). Completed ANOVA equations for calculations of the validity of the method, estimation of potency of sample, and the confidence limit have been described in detail. Examples of calculations that were given are (1) Two-dose multiple assay with completely randomized design, (2) Three-dose Latin square design, (3) Four-dose randomized block design, (4) Five-dose multiple assay with completely randomized design, (5) Twin cross over design.
Douglas Wahlsten , in Mouse Behavioral Testing , 2011
When six objects are to be arranged in different orders and the objects cannot communicate with each other or exert an influence on those adjacent in the order, the Latin square can be used ( Table 7.1 ). Objects can be shifted to the right on each step or to the left on each step, but left and right should not be mixed in the same balancing operation. In the square for six objects, objects are shifted to the right by one position for each order. For six objects, there are six orders, and each object occurs only once in any one place in the sequence. For the example with six sex–treatment combinations, the squad numbers can be shifted to the left by one step, as shown in the spreadsheet ( 4 × 2 × 3 Balanced , Sheet 2 ). This yields three unique sequences. Then append these three end to end in three different sequences and assign them to the first replication. The fourth strain must get a duplicate sequence, perhaps 123123, the same as strain 129. This method yields two duplicate sex–treatment combinations in every squad, a 129-DBA duplication in each case. Now, across replications, assign the sequences so that different pairs of strains are duplicated in a replication. In the spreadsheet the duplicated strains are highlighted in yellow. The nine replications have five duplications for two strains and four for the other two. It cannot be perfectly balanced when there are four strains and nine replications.
Table 7.1 . Latin Square to Determine Order of Six Tests
There are probably many other ways to balance the sequences. An expert in combinatorics or someone who enjoys working with puzzles may devise a more elegant solution. A criterion for an acceptable level of balancing can be proposed: if there are no biases in the end result and no human could possibly determine how one made the sequences just by looking at the end product, it is good enough for use with lab animals. It is not necessary to adopt extreme measures to achieve perfection.
M. Brysbaert , C. Meyers , in Studies in Visual Information Processing , 1993
The stimuli consisted of 700 five-letter words randomly divided over seven groups. Care was taken to incorporate words with which young children were likely to be familiar. Subjects were distributed over seven latin-square groups with each group seeing the words at a different fixation location (see below). All subjects processed the whole sample of stimuli, but in a different order (for the randomization procedure used, see Brysbaert, 1991a ). Stimulus presentation was controlled by an IBM XT microcomputer and displayed on a Philips monochrome CRT screen.
A latin square design is ideal for any experiment in which it is possible to measure each subject under every treatment and, in addition, it is necessary to control for changing conditions over the course of the experiment. A latin square is a design in which each treatment is assigned to each time period the same number of times and to each subject the same number of times (see Dean and Voss 1999 , chap. 12). If there are t treatments, t time periods, and mt subjects then m latin squares (each with t treatment sequences) would be used.
Carry-over effects are controlled by using latin squares that are ‘counterbalanced’ (Cotton 1993 ). This means that, looking at the sequences of treatments assigned to all the subjects taken together, every treatment is preceded by every other treatment for the same number of subjects. Counterbalanced latin squares exist for any even number of treatments and for some odd numbers of treatments (e.g., t =9, 15, 21, 27; see Jones and Kenward 1989 Sect. 5.2.2, for references). For other odd numbers, a pair of latin squares can be used which between them give a set of 2 t counterbalanced sequences.
If a carry-over effect is expected to persist for more than one time period, then the counterbalancing must be extended to treatements occurring more than one time period prior to the current treatment.
We use cookies to help provide and enhance our service and tailor content and ads. By continuing you agree to the use of cookies .
Copyright © 2022 Elsevier B.V. or its licensors or contributors. ScienceDirect ® is a registered trademark of Elsevier B.V.
ScienceDirect ® is a registered trademark of Elsevier B.V.
Come write articles for us and get featured
Learn and code with the best industry experts
Get access to ad-free content, doubt assistance and more!
Come and find your dream job with us
Difficulty Level :
Basic Last Updated :
07 Oct, 2022
// C++ program to print Latin Square
// Function to print n x n Latin Square
// A variable to control the rotation
// This loops runs only after first
// iteration of outer loop. It prints
// This loop prints numbers from 1 to k-1.
for ( int j=1; j" );
// Invoking printLatin function
Check whether a Matrix is a Latin Square or not
Maximum size of square such that all submatrices of that size have sum less than K
Minimum steps to move all 1 to a single cell of given square Matrix
Find Maximum Length Of A Square
Korean Girl Pussy
Japan Korean Sex
Horny Teacher Porn