Latin Hypercube
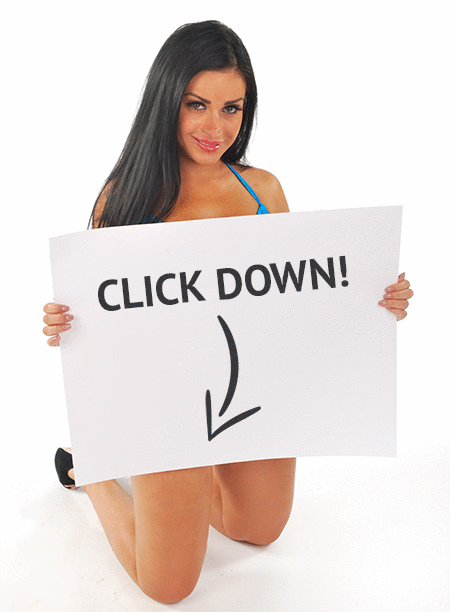
🛑 ALL INFORMATION CLICK HERE 👈🏻👈🏻👈🏻
Latin Hypercube
(2.102) T = 1 N ∑ i = 1 N g ( H i )
URL: https://www.sciencedirect.com/science/article/pii/B9780128096703000023
(50) S i = D i / D S T i = 1 − D − i / D
(51) D = 1 n ∑ k = 1 n f 2 A k − 1 n ∑ k = 1 n f A k 2 D i = 1 n ∑ k = 1 n f A k f B A j k − 1 n ∑ k = 1 n f A k 2 D − i = 1 n ∑ k = 1 n f A k f A B j k − 1 n ∑ k = 1 n f A k 2
URL: https://www.sciencedirect.com/science/article/pii/B978012813543300007X
∑ j = 1 b n ij 2 = r and ∑ j = 1 b n ij n hj = λ ( h ≠ i )
N = | n 11 n 12 … n 1 b 0 0 … 0 n 21 n 22 … n 2 b 0 0 … 0 · · … · … · … · · · … · … · … · n υ 1 n υ 2 … n υ b 0 0 … 0 |
N N T = | r λ λ … λ λ r λ … λ · · · … · · · · … · λ λ λ … r |
det ( N N T ) = [ r + λ ( υ − 1 ) ] | 1 1 1 … 1 λ r λ … λ · · · … · · · · … · λ λ λ … r |
det ( N N T ) = [ r + λ ( υ − 1 ) ] ( r − λ ) υ − 1 = kr ( r − λ ) υ − 1
URL: https://www.sciencedirect.com/science/article/pii/B9780444635556500118
E = a 1 2 l 1 a 1 + 1 2 l 1 × a 2 2 l 2 a 2 + 1 2 l 2 ,
[7.9] x a = b − 1 b − 2 ⋯ b n c 1 , 1 c 1 , 2 ⋯ c 1 , n c 2 , 1 ⋱ c 2 , n ⋮ ⋱ ⋮ c n , 1 ⋯ ⋯ c n , n d 1 a d 2 a ⋮ d n a ,
[7.10] c 1 , 1 c 1 , 2 ⋯ c 1 , n c 2 , 1 ⋱ c 2 , n ⋮ ⋱ ⋮ c n , 1 ⋯ ⋯ c n , n d 1 a d 2 a ⋮ d n a = d 1 c 1 , 1 c 2 , 1 ⋮ c n , 1 + ⋯ + d n c 1 , n c 2 , n ⋮ c n , n .
[7.11] x a = 2 − 1 2 − 2 ⋯ 2 − n v 1 v 2 ⋮ v n = ∑ i = 1 32 2 − i v i .
v = v 1 + 2 v 2 + ⋯ = ∑ i = 1 32 2 i − 1 v i .
URL: https://www.sciencedirect.com/science/article/pii/B9780128006450500075
p ( β m , t | m , y 1 : t , d 1 : t ) = p ( y 1 : t | m , β m , t , d 1 : t ) p ( β m , t | m ) / p ( y 1 : t | m , d 1 : t ) , for t = 1 , … , T ,
p ( y 1 : t + 1 | m , d 1 : t + 1 ) / p ( y 1 : t | m , d 1 : t ) = ∫ β m , t p ( y t + 1 | m , β m , t , d t + 1 ) p ( β m , t | m , y 1 : t , d 1 : t ) d β m , t ,
p ( y 1 : t + 1 | m , d 1 : t + 1 ) / p ( y 1 : t | m , d 1 : t ) ≈ ∑ i = 1 N W m , t ( i ) p ( y t + 1 | m , β m , t ( i ) , d t + 1 ) .
ψ ( d ) = ∑ m = 1 K p ( m ) ∑ z = { 0 , 1 } p ( z | m , d ) ψ ( d , m , z ) ,
∑ m = 1 K p ˆ ( m | y 1 : t , d 1 : t ) ∑ z = { 0 , 1 } p ˆ ( z | m , d , y 1 : t , d 1 : t ) ψ ( d , m , z ) .
URL: https://www.sciencedirect.com/science/article/pii/B9780128037324000064
URL: https://www.sciencedirect.com/science/article/pii/S1569190X2100126X
URL: https://www.sciencedirect.com/science/article/pii/S0303264717300400
URL: https://www.sciencedirect.com/science/article/pii/S0045782517307697
URL: https://www.sciencedirect.com/science/article/pii/S0377221707010090
URL: https://www.sciencedirect.com/science/article/pii/S0377221716304623
] A latin hypercube is the analogous concept in more than three dimensions.
The Latin Hypercube Sampling (LHS) is a type of stratified Monte Carlo (MC). The sampling region is partitioned into a specific manner by dividing the range of each component of x . We will only consider the case where the components of x are independent or can be transformed into an independent base. Moreover, the sample generation for correlated components with Gaussian distribution can be easily achieved. As originally described, in the following manner, LHS operates to generate a sample size N from the x variables x 1 , x 2 , x 3 , . . . . . . . . . x n . The range of each variable is partitioned into N non-overlapping intervals on the basis of equal probability size 1 / N . One value from each interval is selected at random with respect to the probability density in the interval. The N values thus obtained for x 1 are paired in a random manner with the N values of x 2 . These N pairs are combined in a random manner with the N values of x 3 to form Nn -triplets, and so on, until a set of Nn -tuples is formed. This set of Nn -tuples is the Latin hypercube sample. Thus, for given values of N and n , there exist ( N ! ) n − 1 possible interval combinations for an LHS. A 10-run LHS for three normalized variables (range [0, 1]) with the uniform probability density function (p.d.f.) is listed below. In this case, the equal probability spaced values are 0, ..., 0.8, 1.
Efficiency of LHSMC Considers the case that x denotes an n -vectors random variable with p.d.f. f x ( x ) for x ∈ S . Let h denote an objective function given by
Since both methods have limitations and strengths, we propose a new approach that combines both methods; the new approach will be called Monte Carlo Hypercube Sampling Method (MCHSM).
Monte Carlo hypercube sampling method It was demonstrated that the hypercube sample method was more efficient and less time consuming than the Monte Carlo simulation. However, this Monte Carlo simulation still presents some worth. We propose a methodology that combines both the Monte Carlo simulation and the Latin hypercube sampling as follows. Assume that the uncertain parameter is β and it ranges within [ a , b ] , then the first step in this method consists of generating the sampling via the Monte Carlo sampling within [ a , b ] . The next step is to reduce the number of sampling by calculating the mean, the variance, and the standard deviation of the generated sample. These statistical parameters can then further be used to construct a distribution function, for instance, the normal distribution. With constructed distribution in hand, one can further apply the hypercube sample method to generate the final samples.
Ke Fang , Ming Yang , in Model Engineering for Simulation , 2019
Sobol's method ( Sobol, 1990 ) is a global sensitivity analysis method based on variance decomposition, which was proposed by I.M. Sobol in 1990. Its principle is to decompose the multivariant function into a constant, single-variant function and a combined-variant function, and use the variance of multivariant function and each subitem to calculate the sensitivity of different variants.
If the model is modified to rectify the negative factor, it is possible to influence other factors related to the modified one. This requires sensitivity analysis on the negative factor. The more the sensitivity achieved, the more the possibility that it will have a negative influence on other related factors. Take the negative factor p 1 as an example.
First determine the factor set related to p 1 : M ( p 1 ) = { p i ∈ P | s i ∩ s 1 ≠ ∅}\{ p 1 }, i = 1, 2, …, r , and set the sampling interval of coupled factors p i as: [(1 − τ ) × v ( s i ), (1 + τ ) × v ( s i )], p i ∈ M ( p 1 ). τ represents the amplitude and usually τ = 10%.
In the sampling interval, use the Latin hypercube design ( Shields and Zhang, 2016 ) to obtain the sample set. In order to guarantee the precision of sensitivity, the sample number should be no less than 200 times of the number of coupled factors. First make two separate Latin hypercube samplings, and get two experiment plans A n × r and B n × r , where n represents the experiment times and r represents the number of coupled factors. Replacing the j -th column in plan A with the j -th column in plan B makes A B j , and replacing the j -th column in plan B with the j -th column in plan A makes B A j . Then the first-order sensitivity S i and the total sensitivity S T i of p i are
In Formula (50) , D is the total variance of the credibility aggregation function; D i is the parameter variance of p i ; and D − i is the irrelevant variance of p i . They can be calculated by
In Formula (51) , f ( x ) represents the total credibility aggregated by the experiment plan x , and the subscript k represents the k -th experiment in the plan.
Usually we take the total sensitivity as the criteria to determine whether the coupled factor p i is sensitive to the total credibility, with a threshold of 0.6. If it is sensitive, when the origin factor (negative factor) p 1 is rectified, the engineers should pay attention to the chain reaction of the coupled factor p i .
Since the 1930s, when the idea of doing so was pioneered by R.A. Fisher, latin squares and other related designs have been much used in the design of statistical experiments whose subsequent interpretation is to be effected with the aid of the procedure known as the “analysis of variance”. 4 We have already referred to such statistical applications in several earlier chapters of the present book: notably in Section 2.6 , Section 5.6 and Section 6.4 , where the uses of complete latin squares, of latin cubes and hypercubes, and of Room designs for this purpose were mentioned. By means of an example, we shall explain next the use of orthogonal latin squares for the same purpose.
Suppose that it is required to test the effect of five different treatments on yarn. Five looms are available for spinning the yarn and it is believed that both the loom used and the operator employed may affect the final texture of the spun yarn. If time were of no account, it would be desirable ideally to test the effect of every treatment with every operator and on every loom. However, by making use of the statistical technique of analysis of variance, a satisfactory test result can be obtained provided that each type of treated yarn is spun once on each loom and also once by each operator. If five operators are employed, the necessary set of twenty-five experiments can be specified by means of the 5 × 5 latin square illustrated in the left-hand diagram of Figure 11.5.1 . Here, the columns 0 1 , 0 2 , 0 3 , 0 4 , 0 5 specify the operator and the rows L 1 , L 2 , L 3 , L 4 , L 5 specify which loom is to be used for the particular experiment. The entries Y 0 , Y 1 , Y 2 , Y 3 , Y 4 in the body of the square indicate which type of treated yarn is to be used. (Usually, one sample of yarn, called the control, is left untreated and the suffix 0 is used for this type of treatment.) Thus, in the first experiment, yarn Y 1 is to be spun by operator 0 1 , on loom L 1 . In another experiment, yarn Y 3 is to be spun by operator 0 2 on loom L 4 . If it were also the case that the efficiency of the loom operators varied with the day of the week (five weekdays), this extra source of variation could be allowed for by using a 5 × 5 latin square which was orthogonal to the first to specify the day of the week on which each experiment was to be performed. Thus, in the right-hand square illustrated in Figure 11.5.1 , the integers 0, 1, 2, 3, 4 are used to specify the five days of a week. Then each loom, each operator, and each type of treated yarn are associated with each day of the week just once.
The use of a latin square design for an experiment of the above kind is somewhat restrictive. It might happen, for example, that only four operators were available. This situation could be accommodated by using the same latin square with its last column deleted. It would still be the case that each type of yarn would be spun by each of the four remaining operators but now only four of the five different yarns would be spun on any one of the looms. A development of this observation gives rise to the concept of a balanced incomplete block design .
A balanced incomplete block design comprises a set of υ varieties (or treatments ) arranged in b blocks (or rows) in such a way that (i) each block has the same number k of treatments ( k < υ ), no treatment occurring twice in the same block; (ii) each treatment occurs in exactly r (= λ 1 ) blocks; (iii) each pair of treatments occur together in exactly λ (= λ 2 ) blocks.
Balanced incomplete block designs were first introduced by F. Yates in Yates (1936) . An example of such a design is given by the latin square used above when the last column is deleted. This has parameters b = 5, υ = 5, k = 4, r = 4, λ = 3.
A design for which property (iii) is satisfied is called pairwise balanced . More generally, if each set of t treatments occurs in exactly λ t blocks, the design is called t-wise balanced or, more briefly, a t-design . A very well-known result is the following:
The parameters b, v, r, k , λ of a (pairwise) balanced incomplete block design (usually written briefly as a BIBD) satisfy the relations bk = υr , λ ( υ − 1) = r ( k − 1), r > λ , b ≥ υ .
Each side of the first relation is an expression for the total number of treatments to be found in all the blocks. Each side of the second relation is an expression for the total number of treatments (other than υ i ) which occur in the various blocks which contain an assigned treatment υ i . Next, if r > λ were untrue, then with any given treatment every other treatment would occur in every block, contradicting k < υ . Finally, to show that b ≥ υ it is easiest to make use of the incidence matrix of the design. Let a BIBD with parameters b , υ , r , k , λ be given and let n ij = 1 or 0 according as the i th treatment υ i does or does not occur in the j th block. The υ × b matrix so obtained is the incidence matrix of the design. Clearly,
by definition of r and λ . If possible, let b < υ and consider the υ × υ matrix
where the last υ − b columns consist of zeros. It follows from the relations above that
by addition of rows. Then, subtracting the first column from each other one, we get
since λ ( υ − 1) = r ( k − 1). But det( NN T ) = (det N )(det N T ) = 0 which implies that r = λ . This contradiction shows that the supposition k < υ is impossible. Therefore, b ≥ υ as required. □
A BIBD for which b = υ and consequently r = k is said to be symmetric and such a design may also be referred to as a ( υ , k , λ )- design .
Two obvious problems concerning BIBD ’s are to provide methods of constructing them and to determine for what values of the parameters such designs exist. The second problem is not completely solved. As regards the first, many methods have been devised. Here, we mention that a symmetric BIBD is a finite projective plane or a complete set of MOLS . (See Section 5.2 .) Also, a BIBD with k = 3 and λ = 1 is a Steiner triple system . (See Section 2.3 .)
Chapter 10 of [DK2] is devoted to a quite detailed explanation of the appropriateness of the use of latin squares and other block designs in the design of experiments of various kinds and to an account of the analysis of the results, so we shall not repeat that account here.
An earlier account of the same topic is that of Hedayat and Shrikhande(1971) .
Matt Pharr , ... Greg Humphreys , in Physically Based Rendering (Third Edition) , 2017
Another approach for generating high-quality samples takes advantage of a remarkable property of certain low-discrepancy sequences that allows us to satisfy two desirable properties of samples (only one of which was satisfied with the StratifiedSampler ): they generate sample vectors for a pixel’s worth of image samples such that the sample values for each pixel sample are well distributed with respect to each other, and simultaneously such that the aggregate collection of sample values for all of the pixel samples in the pixel are collectively well distributed.
HaltonSampler::PermutationForDimension() 452
This sequence uses the first two dimensions of a low-discrepancy sequence derived by Sobol′. 9 This sequence is a special type of low-discrepancy sequence known as a (0, 2)-sequence. (0, 2)-sequences are stratified in a very general way. For example, the first 16 samples in a (0, 2)-sequence satisfy the stratification constraint from stratified sampling in Section 7.3 , meaning there is just one sample in each of the boxes of extent 1 4 1 4 . However, they also satisfy the Latin hypercube constraint, as only one of them is in each of the boxes of extent 1 16 1 and 1 1 16 . Furthermore, there is only one sample in each of the boxes of extent 1 2 1 8 and 1 8 1 2 . Figure 7.28 shows all of the possibilities for dividing the domain into regions where the first 16 samples of a (0, 2)-sequence satisfy the stratification properties. Each succeeding sequence of 16 samples from this pattern also satisfies these distribution properties.
Figure 7.28 . A sampling pattern that has a single sample in all of the base 2 elementary intervals. It satisfies both the 4 × 4 stratification and Latin hypercube constraints as well as the other two stratification constraints shown.
In general, any sequence of length 2 l 1 + l 2 (where l i is a nonnegative integer) from a (0, 2)-sequence satisfies this general stratification constraint. The set of elementary intervals in two dimensions, base 2, is defined as
where the integer a i = 0 , 1 , 2 , 4 , … , 2 l i − 1 . One sample from each of the first 2 l 1 + l 2 values in the sequence will be in each of the elementary intervals. Furthermore, the same property is true for each subsequent set of 2 l 1 + l 2 values.
To understand now how (0, 2)-sequences can be applied to generating 2D samples, consider a pixel with 2 × 2 image samples, each with an array of 4 × 4 2D samples. The first (2 × 2) × (4 × 4) = 2 6 values of a (0, 2)-sequence are well distributed with respect to each other according to the corresponding set of elementary intervals. Furthermore, the first 4 × 4 = 2 4 samples are themselves well distributed according to their corresponding elementary intervals, as are the next 2 4 of them, and the subsequent ones, and so on. Therefore, we can use the first 16 (0, 2)-sequence samples for the samples for the 4 × 4 array for the first image sample for a pixel, then the next 16 for the next image sample, and so forth. The result is an extremely well-distributed set of sample points.
The Sobol′ sequence is based on a different mechanism for generating sample points than the HaltonSampler , which used the radical inverse in various dimensions. Even with the integer divides in the radical inverse function converted to multiplies and shifts, the amount of computation needed to compute the billions of samples that can be needed for high-quality, high-resolution renderings can still be significant. Most of the computational expense comes from the cost of performing non-base-2 computation on computers that natively operate in base 2. (Consider the contrast between the 〈 Compute base-2 radical inverse 〉 fragment and the RadicalInverseSpecialized() template function.)
Given the high cost of non-base-2 operations, it’s natural to try to develop sample generation algorithms that operate entirely in base 2. One such approach that has been effective has been to use generator matrices that allow all computation to be done in the same base. Instead of using a different base in each dimension, as the Halton sampler did, a different generator matrix is used in each dimension. With well-chosen matrices for each sampled dimension, it’s possible to generate very good low-discrepancy distributions of points. For example, (0, 2)-sequences can be defined using two specific generator matrices in base 2.
To see how generator matrices are used, consider an n -digit number a in base b , where the i th digit of a is d i ( a ) and where we have an n × n generator matrix C . Then the corresponding sample point x a ∈ [0, 1) is defined by
where all arithmetic is performed in the ring Z b (in other words, when all operations are performed modulo b ). This construction gives a total of b n points as a ranges from 0 to b n − 1. If the generator matrix is the identity matrix, then this definition corresponds to the regular radical inverse, base b . (It’s worth pausing to make sure you see this connection between Equations (7.7) and (7.9) before continuing.)
In this section, we will exclusively use b = 2 and n = 32. While introducing a 32 × 32 matrix to the sample generation algorithm may not seem like a step toward better performance, we’ll see that in the end the sampling code can be map
Heels Sound 1.3
Latin Kvartal Cup 2022
Porn Brother S Hard Cock