Галерея 3427777
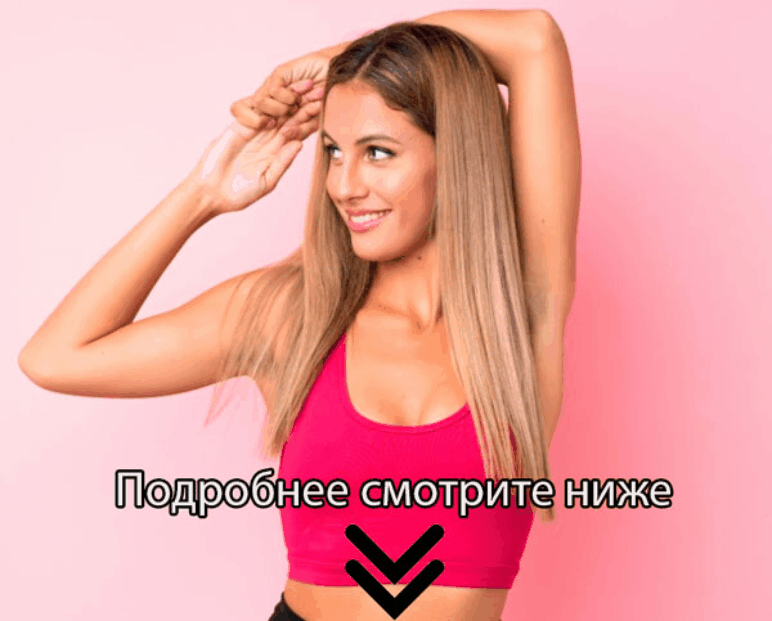
Галерея 3427777
Возможно, сайт временно недоступен или перегружен запросами. Подождите некоторое время и попробуйте снова.
Если вы не можете загрузить ни одну страницу – проверьте настройки соединения с Интернетом.
Если ваш компьютер или сеть защищены межсетевым экраном или прокси-сервером – убедитесь, что Firefox разрешён выход в Интернет.
Firefox не может установить соединение с сервером www.premiersothebysrealty.com.
Отправка сообщений о подобных ошибках поможет Mozilla обнаружить и заблокировать вредоносные сайты
Сообщить
Попробовать снова
Отправка сообщения
Сообщение отправлено
использует защитную технологию, которая является устаревшей и уязвимой для атаки. Злоумышленник может легко выявить информацию, которая, как вы думали, находится в безопасности.
Sign up or log in to customize your list.
more stack exchange communities
company blog
Stack Overflow for Teams
– Start collaborating and sharing organizational knowledge.
Create a free Team
Why Teams?
Asked
9 years, 2 months ago
31k 21 21 gold badges 105 105 silver badges 126 126 bronze badges
54.5k 41 41 gold badges 77 77 silver badges 95 95 bronze badges
Sorted by:
Reset to default
Highest score (default)
Trending (recent votes count more)
Date modified (newest first)
Date created (oldest first)
52.2k 32 32 gold badges 133 133 silver badges 143 143 bronze badges
45.5k 5 5 gold badges 61 61 silver badges 49 49 bronze badges
31k 21 21 gold badges 105 105 silver badges 126 126 bronze badges
365k 92 92 gold badges 677 677 silver badges 731 731 bronze badges
14.2k 13 13 gold badges 79 79 silver badges 127 127 bronze badges
123k 20 20 gold badges 215 215 silver badges 185 185 bronze badges
31k 21 21 gold badges 105 105 silver badges 126 126 bronze badges
6,914 13 13 gold badges 48 48 silver badges 63 63 bronze badges
30.5k 8 8 gold badges 89 89 silver badges 98 98 bronze badges
55.1k 17 17 gold badges 158 158 silver badges 140 140 bronze badges
387 3 3 silver badges 5 5 bronze badges
31k 21 21 gold badges 105 105 silver badges 126 126 bronze badges
470 6 6 silver badges 14 14 bronze badges
1,700 11 11 silver badges 29 29 bronze badges
31k 21 21 gold badges 105 105 silver badges 126 126 bronze badges
1,696 18 18 silver badges 21 21 bronze badges
4,275 4 4 gold badges 24 24 silver badges 43 43 bronze badges
1,627 13 13 silver badges 17 17 bronze badges
31k 21 21 gold badges 105 105 silver badges 126 126 bronze badges
2,332 17 17 silver badges 29 29 bronze badges
31k 21 21 gold badges 105 105 silver badges 126 126 bronze badges
319 1 1 gold badge 4 4 silver badges 16 16 bronze badges
31k 21 21 gold badges 105 105 silver badges 126 126 bronze badges
549 3 3 silver badges 16 16 bronze badges
31k 21 21 gold badges 105 105 silver badges 126 126 bronze badges
47.1k 20 20 gold badges 85 85 silver badges 107 107 bronze badges
31k 21 21 gold badges 105 105 silver badges 126 126 bronze badges
31k 21 21 gold badges 105 105 silver badges 126 126 bronze badges
466 4 4 silver badges 6 6 bronze badges
31k 21 21 gold badges 105 105 silver badges 126 126 bronze badges
6,220 3 3 gold badges 12 12 silver badges 40 40 bronze badges
31k 21 21 gold badges 105 105 silver badges 126 126 bronze badges
794 5 5 silver badges 9 9 bronze badges
31k 21 21 gold badges 105 105 silver badges 126 126 bronze badges
331 1 1 gold badge 2 2 silver badges 7 7 bronze badges
Highly active question . Earn 10 reputation (not counting the association bonus ) in order to answer this question. The reputation requirement helps protect this question from spam and non-answer activity.
Not the answer you're looking for? Browse other questions tagged python pandas dataframe chained-assignment or ask your own question .
Stack Overflow
Questions
Help
Products
Teams
Advertising
Collectives
Talent
Company
About
Press
Work Here
Legal
Privacy Policy
Terms of Service
Contact Us
Cookie Settings
Cookie Policy
Stack Exchange Network
Technology
Culture & recreation
Life & arts
Science
Professional
Business
API
Data
Accept all cookies
Necessary cookies only
Find centralized, trusted content and collaborate around the technologies you use most.
Connect and share knowledge within a single location that is structured and easy to search.
I just upgraded my Pandas from 0.11 to 0.13.0rc1. Now, the application is popping out many new warnings. One of them like this:
I want to know what exactly it means? Do I need to change something?
How should I suspend the warning if I insist to use quote_df['TVol'] = quote_df['TVol']/TVOL_SCALE ?
The SettingWithCopyWarning was created to flag potentially confusing "chained" assignments, such as the following, which does not always work as expected, particularly when the first selection returns a copy . [see GH5390 and GH5597 for background discussion.]
The warning offers a suggestion to rewrite as follows:
However, this doesn't fit your usage, which is equivalent to:
While it's clear that you don't care about writes making it back to the original frame (since you are overwriting the reference to it), unfortunately this pattern cannot be differentiated from the first chained assignment example. Hence the (false positive) warning. The potential for false positives is addressed in the docs on indexing , if you'd like to read further. You can safely disable this new warning with the following assignment.
This post is meant for readers who,
To know how to deal with this warning, it is important to understand what it means and why it is raised in the first place.
When filtering DataFrames, it is possible slice/index a frame to return either a view , or a copy , depending on the internal layout and various implementation details. A "view" is, as the term suggests, a view into the original data, so modifying the view may modify the original object. On the other hand, a "copy" is a replication of data from the original, and modifying the copy has no effect on the original.
As mentioned by other answers, the SettingWithCopyWarning was created to flag "chained assignment" operations. Consider df in the setup above. Suppose you would like to select all values in column "B" where values in column "A" is > 5. Pandas allows you to do this in different ways, some more correct than others. For example,
These return the same result, so if you are only reading these values, it makes no difference. So, what is the issue? The problem with chained assignment, is that it is generally difficult to predict whether a view or a copy is returned, so this largely becomes an issue when you are attempting to assign values back. To build on the earlier example, consider how this code is executed by the interpreter:
With a single __setitem__ call to df . OTOH, consider this code:
Now, depending on whether __getitem__ returned a view or a copy, the __setitem__ operation may not work .
In general, you should use loc for label-based assignment, and iloc for integer/positional based assignment, as the spec guarantees that they always operate on the original. Additionally, for setting a single cell, you should use at and iat .
More can be found in the documentation .
Note
All boolean indexing operations done with loc can also be done with iloc . The only difference is that iloc expects either
integers/positions for index or a numpy array of boolean values, and
integer/position indexes for the columns.
Consider a simple operation on the "A" column of df . Selecting "A" and dividing by 2 will raise the warning, but the operation will work.
There are a couple ways of directly silencing this warning:
(recommended) Use loc to slice subsets :
Change pd.options.mode.chained_assignment
Can be set to None , "warn" , or "raise" . "warn" is the default. None will suppress the warning entirely, and "raise" will throw a SettingWithCopyError , preventing the operation from going through.
@Peter Cotton in the comments, came up with a nice way of non-intrusively changing the mode (modified from this gist ) using a context manager, to set the mode only as long as it is required, and the reset it back to the original state when finished.
A lot of the time, users attempt to look for ways of suppressing this exception without fully understanding why it was raised in the first place. This is a good example of an XY problem , where users attempt to solve a problem "Y" that is actually a symptom of a deeper rooted problem "X". Questions will be raised based on common problems that encounter this warning, and solutions will then be presented.
I want to assign values in col "A" > 5 to 1000. My expected output is
Question 2 1
I am trying to set the value in cell (1, 'D') to 12345. My expected output is
I have tried different ways of accessing this cell, such as
df['D'][1] . What is the best way to do this?
1. This question isn't specifically related to the warning, but
it is good to understand how to do this particular operation correctly
so as to avoid situations where the warning could potentially arise in
future.
You can use any of the following methods to do this.
Question 3
I am trying to subset values based on some condition. I have a
DataFrame
I would like to assign values in "D" to 123 such that "C" == 5. I
tried
Which seems fine but I am still getting the
SettingWithCopyWarning ! How do I fix this?
This is actually probably because of code higher up in your pipeline. Did you create df2 from something larger, like
? In this case, boolean indexing will return a view, so df2 will reference the original. What you'd need to do is assign df2 to a copy :
Question 4
I'm trying to drop column "C" in-place from
Throws SettingWithCopyWarning . Why is this happening?
This is because df2 must have been created as a view from some other slicing operation, such as
The solution here is to either make a copy() of df , or use loc , as before.
In general the point of the SettingWithCopyWarning is to show users (and especially new users) that they may be operating on a copy and not the original as they think. There are false positives (IOW if you know what you are doing it could be ok ). One possibility is simply to turn off the (by default warn ) warning as @Garrett suggest.
You can set the is_copy flag to False , which will effectively turn off the check, for that object :
If you explicitly copy then no further warning will happen:
The code the OP is showing above, while legitimate, and probably something I do as well, is technically a case for this warning, and not a false positive. Another way to not have the warning would be to do the selection operation via reindex , e.g.
Here I answer the question directly. How can we deal with it?
Make a .copy(deep=False) after you slice. See pandas.DataFrame.copy .
Wait, doesn't a slice return a copy? After all, this is what the warning message is attempting to say? Read the long answer:
Both df0 and df1 are DataFrame objects, but something about them is different that enables pandas to print the warning. Let's find out what it is.
Using your diff tool of choice, you will see that beyond a couple of addresses, the only material difference is this:
The method that decides whether to warn is DataFrame._check_setitem_copy which checks _is_copy . So here you go. Make a copy so that your DataFrame is not _is_copy .
The warning is suggesting to use .loc , but if you use .loc on a frame that _is_copy , you will still get the same warning. Misleading? Yes. Annoying? You bet. Helpful? Potentially, when chained assignment is used. But it cannot correctly detect chain assignment and prints the warning indiscriminately.
When you go and do something like this:
pandas.ix in this case returns a new, stand alone dataframe.
Any values you decide to change in this dataframe, will not change the original dataframe.
This is what pandas tries to warn you about.
The .ix object tries to do more than one thing, and for anyone who has read anything about clean code, this is a strong smell.
Behavior one: dfcopy is now a stand alone dataframe. Changing it will not change df
Behavior two: This changes the original dataframe.
The pandas developers recognized that the .ix object was quite smelly[speculatively] and thus created two new objects which helps in the accession and assignment of data. (The other being .iloc )
.loc is faster, because it does not try to create a copy of the data.
.loc is meant to modify your existing dataframe inplace, which is more memory efficient.
.loc is predictable, it has one behavior.
What you are doing in your code example is loading a big file with lots of columns, then modifying it to be smaller.
The pd.read_csv function can help you out with a lot of this and also make the loading of the file a lot faster.
This will only read the columns you are interested in, and name them properly. No need for using the evil .ix object to do magical stuff.
This topic is really confusing with Pandas. Luckily, it has a relatively simple solution.
The problem is that it is not always clear whether data filtering operations (e.g. loc) return a copy or a view of the DataFrame. Further use of such filtered DataFrame could therefore be confusing.
The simple solution is (unless you need to work with very large sets of data):
Whenever you need to update any values, always make sure that you explicitly copy the DataFrame before the assignment.
I had been getting this issue with .apply() when assigning a new dataframe from a pre-existing dataframe on which I've used the .query() method. For instance:
Would return this error. The fix that seems to resolve the error in this case is by changing this to:
However, this is not efficient especially when using large dataframes, due to having to make a new copy.
If you're using the .apply() method in generating a new column and its values, a fix that resolves the error and is more efficient is by adding .reset_index(drop=True) :
To remove any doubt, my solution was to make a deep copy of the slice instead of a regular copy.
This may not be applicable depending on your context (Memory constraints / size of the slice, potential for performance degradation - especially if the copy occurs in a loop like it did for me, etc...)
To be clear, here is the warning I received:
I had doubts that the warning was thrown because of a column I was dropping on a copy of the slice. While not technically trying to set a value in the copy of the slice, that was still a modification of the copy of the slice.
Below are the (simplified) steps I have taken to confirm the suspicion, I hope it will help those of us who are trying to understand the warning.
We knew that already but this is a healthy reminder. This is NOT what the warning is about.
It is possible to avoid changes made on df1 to affect df2. Note: you can avoid importing copy.deepcopy by doing df.copy() instead.
This actually illustrates the warning.
It is possible to avoid changes made on df2 to affect df1
Some may want to simply suppress the warning:
As this question is already fully explained and discussed in existing answers, I will just provide a neat pandas approach to the context manager using pandas.option_context (links to documentation and example ) - there is absolutely isn't any need to create a custom class with all the dunder methods and other bells and whistles.
First the context manager code itself:
It is worth noticing is that both approaches do not modify a , which is a bit surprising to me, and even a shallow df copy with .copy(deep=False) would prevent this warning to be raised (as far as I understand, shallow copy should at least modify a as well
Опытная шалава снимает трусики и предлагает сделать ей приятно парень не стал теряться он вставил пенис в ее промежность
Писающие телочки
Молодая и зрелая красотки решили поделить между собой большой член и старательно его ублажают