Галерея 3413971
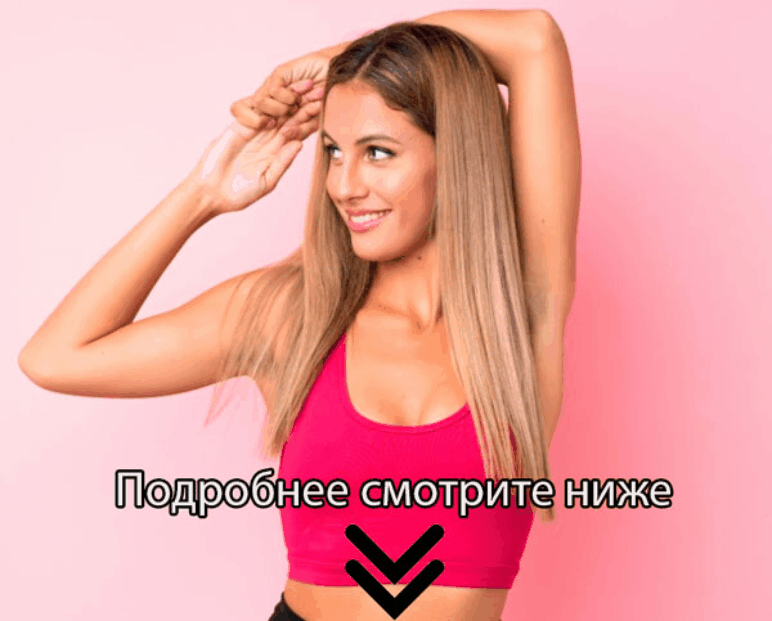
🛑 ПОДРОБНЕЕ ЖМИТЕ ЗДЕСЬ 👈🏻👈🏻👈🏻
Галерея 3413971
Sign in
Register
Label Embedding Online Hashing for Cross-Modal Retrieval
Published: 12 October 2020 Publication History
MM '20: Proceedings of the 28th ACM International Conference on Multimedia
Overall Acceptance Rate 946 of 3,859 submissions, 25%
Sponsor:
sigmm
Ottawa ,
ON ,
Canada
Funding Sources Fundamental Research Funds of Shandong University Shandong Provincial Key Research and Development Program National Natural Science Foundation of China
Fatih Cakir, Sarah Adel Bargal, and Stan Sclaroff. 2017a. Online supervised hashing. Comput. Vision Image Understanding, Vol. 156 (2017), 162--173. Google Scholar Digital Library Fatih Cakir, Kun He, Sarah Adel Bargal, and Stan Sclaroff. 2017b. MIHash: Online hashing with mutual information. In Proc. IEEE Int. Conf. Comput. Vis. 437--445. Google Scholar Cross Ref Fatih Cakir and Stan Sclaroff. 2015. Adaptive hashing for fast similarity search. In Proc. IEEE Int. Conf. Comput. Vis. 1044--1052. Google Scholar Digital Library Moses S Charikar. 2002. Similarity estimation techniques from rounding algorithms. In Proc. ACM Symposium on Theory of Compu. 380--388. Google Scholar Digital Library Junxuan Chen, Yaoyi Li, and Hongtao Lu. 2016. Online self-organizing hashing. In Proc. IEEE Int. Conf. Multimedia and Expo. 1--6. Google Scholar Cross Ref Yong Chen, Zhibao Tian, Hui Zhang, Jun Wang, and Dell Zhang. 2020 b. Strongly constrained discrete hashing. IEEE Trans. Image Process., Vol. 29, 1 (2020), 3596--3611. Google Scholar Digital Library Zhen Duo Chen, Chuan Xiang Li, Xin Luo, Liqiang Nie, Wei Zhang, and Xin Shun Xu. 2020 a. SCRATCH: A scalable discrete matrix factorization hashing framework for cross-modal retrieval. IEEE Trans. Circuits Syst. Video Technol., Vol. 30, 7 (2020), 2262--2275. Google Scholar Digital Library Zhen Duo Chen, Yongxin Wang, Hui Qiong Li, Xin Luo, Liqiang Nie, and Xin Shun Xu. 2019. A two-step cross-modal hashing by exploiting label correlations and preserving similarity in both steps. In Proc. ACM Multimedia Conf. 1694--1702. Google Scholar Digital Library Tat Seng Chua, Jinhui Tang, Richang Hong, Haojie Li, Zhiping Luo, and Yantao Zheng. 2009. NUS-WIDE: A real-world web image database from National University of Singapore. In Proc. ACM Int. Conf. Image Video Retr. 48. Google Scholar Digital Library Cheng Da, Shibiao Xu, Kun Ding, Gaofeng Meng, Shiming Xiang, and Chunhong Pan. 2017. Asymmetric multi-valued hashing. In Proc. IEEE Conf. Comput. Vis. Pattern Recognit. 736--744. Google Scholar Cross Ref Guiguang Ding, Yuchen Guo, and Jile Zhou. 2014. Collective matrix factorization hashing for multimodal data. In Proc. IEEE Conf. Comput. Vis. Pattern Recognit. 2075--2082. Google Scholar Digital Library Hugo Jair Escalante, Carlos A Hernandez, Jesus A Gonzalez, A Lopezlopez, Manuel Montes, Eduardo F Morales, L Enrique Sucar, Luis Villasenor, and Michael Grubinger. 2010. The segmented and annotated IAPR TC-12 benchmark. Comput. Vision Image Understanding, Vol. 114, 4 (2010), 419--428. Google Scholar Digital Library Jianwu Fang, Hongke Xu, Qi Wang, and Tianjun Wu. 2017. Online hash tracking with spatio-temporal saliency auxiliary. Comput. Vision Image Understanding, Vol. 160 (2017), 57--72. Google Scholar Cross Ref Yunchao Gong, Svetlana Lazebnik, Albert Gordo, and Florent Perronnin. 2013. Iterative quantization: A procrustean approach to learning binary codes for large-scale image retrieval. IEEE Trans. Pattern Anal. Mach. Intell., Vol. 35, 12 (2013), 2916--2929. Google Scholar Digital Library Mengqiu Hu, Yang Yang, Fumin Shen, Ning Xie, Richang Hong, and Heng Tao Shen. 2019. Collective reconstructive embeddings for cross-modal hashing. IEEE Trans. Image Process., Vol. 28, 6 (2019), 2770--2784. Google Scholar Long Kai Huang, Qiang Yang, and Wei Shi Zheng. 2018. Online hashing. IEEE Trans. Neural Netw. Learn. Syst., Vol. 29, 6 (2018), 2309--2322. Google Scholar Cross Ref Mark J Huiskes and Michael S Lew. 2008. The MIR flickr retrieval evaluation. In Proc. ACM Int. Conf. on Multimedia Inf. Retr. 39--43. Google Scholar Digital Library Prateek Jain, Brian Kulis, Inderjit S Dhillon, and Kristen Grauman. 2009. Online metric learning and fast similarity search. In Proc. Neural Inf. Process. Syst. 761--768. Google Scholar Rongrong Ji, Hong Liu, Liujuan Cao, Di Liu, Yongjian Wu, and Feiyue Huang. 2017. Toward optimal manifold hashing via discrete locally linear embedding. IEEE Trans. Image Process., Vol. 26, 11 (2017), 5411--5420. Google Scholar Digital Library Qing Yuan Jiang and Wu Jun Li. 2019. Discrete latent factor model for cross-modal hashing. IEEE Trans. Image Process., Vol. 28, 7 (2019), 3490--3501. Google Scholar Digital Library Zhongming Jin, Yao Hu, Yue Lin, Debing Zhang, Shiding Lin, Deng Cai, and Xuelong Li. 2013. Complementary projection hashing. In Proc. IEEE Int. Conf. Comput. Vis. 257--264. Google Scholar Digital Library Brian Kulis and Trevor Darrell. 2009. Learning to hash with binary reconstructive embeddings. In Proc. Neural Inf. Process. Syst. 1042--1050. Google Scholar Chuan Xiang Li, Ting Kun Yan, Xin Luo, Liqiang Nie, and Xin Shun Xu. 2019. Supervised robust discrete multimodal hashing for cross-media retrieval. IEEE Trans. Multimedia, Vol. 21, 11 (2019), 2863--2877. Google Scholar Cross Ref Mingbao Lin, Rongrong Ji, Shen Chen, Xiaoshuai Sun, and Chia Wen Lin. 2020 a. Similarity-preserving linkage hashing for online image retrieval. IEEE Trans. Image Process., Vol. 29 (2020), 5289--5300. Google Scholar Cross Ref Mingbao Lin, Rongrong Ji, Hong Liu, Xiaoshuai Sun, Shen Chen, and Qi Tian. 2020 b. Hadamard matrix guided online hashing. Int. J. Comput. Vision (2020). https://doi.org/10.1007/s11263-020-01332-z Google Scholar Mingbao Lin, Rongrong Ji, Hong Liu, Xiaoshuai Sun, Yongjian Wu, and Yunsheng Wu. 2019. Towards optimal discrete online hashing with balanced similarity. In Proc. AAAI Conf. Artif. Intell. 1635--1643. Google Scholar Digital Library Zijia Lin, Guiguang Ding, Mingqing Hu, and Jianmin Wang. 2015. Semantics-preserving hashing for cross-view retrieval. In Proc. IEEE Conf. Comput. Vis. Pattern Recognit. 3864--3872. Google Scholar Cross Ref Hong Liu, Rongrong Ji, Yongjian Wu, Feiyue Huang, and Baochang Zhang. 2017. Cross-modality binary code learning via fusion similarity hashing. In Proc. IEEE Conf. Comput. Vis. Pattern Recognit. 7380--7388. Google Scholar Cross Ref Wei Liu, Cun Mu, Sanjiv Kumar, and Shih Fu Chang. 2014. Discrete graph hashing. In Proc. Neural Inf. Process. Syst. 3419--3427. Google Scholar Wei Liu, Jun Wang, Rongrong Ji, and Yu Gang Jiang. 2012. Supervised hashing with kernels. In Proc. IEEE Conf. Comput. Vis. Pattern Recognit. 2074--2081. Google Scholar Wei Liu, Jun Wang, Sanjiv Kumar, and Shih Fu Chang. 2011. Hashing with graphs. In Proc. Int. Conf. Mach. Learn. 1--8. Google Scholar Mingsheng Long, Yue Cao, Jianmin Wang, and Philip S Yu. 2016. Composite correlation quantization for efficient multimodal retrieval. In Proc. Int. ACM SIGIR Conf. Res. Develop. Inf. Retr. 579--588. Google Scholar Digital Library Xu Lu, Lei Zhu, Zhiyong Cheng, Jingjing Li, Xiushan Nie, and Huaxiang Zhang. 2019. Flexible online multi-modal hashing for large-scale multimedia retrieval. In Proc. ACM Multimedia Conf. 1129--1137. Google Scholar Digital Library Xin Luo, Liqiang Nie, Xiangnan He, Ye Wu, Zhen Duo Chen, and Xin Shun Xu. 2018a. Fast scalable supervised hashing. In Proc. Int. ACM SIGIR Conf. Res. Develop. Inf. Retr. 735--744. Google Scholar Digital Library Xin Luo, Ye Wu, and Xin Shun Xu. 2018b. Scalable supervised discrete hashing for large-scale search. In Proc. World Wide Web Conf. 1603--1612. Google Scholar Digital Library Xin Luo, Xiao Ya Yin, Liqiang Nie, Xuemeng Song, Yongxin Wang, and Xin Shun Xu. 2018c. SDMCH: Supervised discrete manifold-embedded cross-modal hashing. In Proc. Int. Joint Conf. Artif. Intell. 2518--2524. Google Scholar Cross Ref Xin Luo, Peng Fei Zhang, Zi Huang, Liqiang Nie, and Xin Shun Xu. 2019. Discrete hashing with multiple supervision. IEEE Trans. Image Process., Vol. 28, 6 (2019), 2962--2975. Google Scholar Cross Ref Devraj Mandal, Kunal N Chaudhury, and Soma Biswas. 2019. Generalized semantic preserving hashing for cross-modal retrieval. IEEE Trans. Image Process., Vol. 28, 1 (2019), 102--112. Google Scholar Digital Library Mengshi Qi, Yunhong Wang, and Annan Li. 2017. Online cross-modal scene retrieval by binary representation and semantic graph. (2017), 744--752. Google Scholar Fumin Shen, Chunhua Shen, Wei Liu, and Heng Tao Shen. 2015. Supervised discrete hashing. In Proc. IEEE Conf. Comput. Vis. Pattern Recognit. 37--45. Google Scholar Cross Ref Fumin Shen, Yan Xu, Li Liu, Yang Yang, Zi Huang, and Heng Tao Shen. 2018. Unsupervised deep hashing with similarity-adaptive and discrete optimization. IEEE Trans. Pattern Anal. Mach. Intell., Vol. 40, 12 (2018), 3034--3044. Google Scholar Digital Library Di Wang, Xinbo Gao, Xiumei Wang, and Lihuo He. 2019. Label consistent matrix factorization hashing for large-scale cross-modal similarity search. IEEE Trans. Pattern Anal. Mach. Intell., Vol. 41, 10 (2019), 2466--2479. Google Scholar Digital Library Jingdong Wang, Ting Zhang, Nicu Sebe, Heng Tao Shen, et al. 2017. A survey on learning to hash. IEEE Trans. Pattern Anal. Mach. Intell., Vol. 40, 4 (2017), 769--790. Google Scholar Yair Weiss, Antonio Torralba, and Rob Fergus. 2009. Spectral hashing. In Proc. Neural Inf. Process. Syst. 1753--1760. Google Scholar Zhenyu Weng and Yuesheng Zhu. 2020. Online hashing with efficient updating of binary codes. In Proc. AAAI Conf. Artif. Intell. 12354--12361. Google Scholar Cross Ref Liang Xie, Jialie Shen, Jungong Han, Lei Zhu, and Ling Shao. 2017. Dynamic multi-view hashing for online image retrieval. In Proc. Int. Joint Conf. Artif. Intell. 3133--3139. Google Scholar Cross Ref Liang Xie, Jialie Shen, and Lei Zhu. 2016. Online cross-modal hashing for web image retrieval. In Proc. AAAI Conf. Artif. Intell. 294--300. Google Scholar Xing Xu, Fumin Shen, Yang Yang, Heng Tao Shen, and Xuelong Li. 2017. Learning discriminative binary codes for large-scale cross-modal retrieval. IEEE Trans. Image Process., Vol. 26, 5 (2017), 2494--2507. Google Scholar Digital Library Yang Yang, Fumin Shen, Heng Tao Shen, Hanxi Li, and Xuelong Li. 2015. Robust discrete spectral hashing for large-scale image semantic indexing. IEEE Trans. Big Data, Vol. 1, 4 (2015), 162--171. Google Scholar Cross Ref Tao Yao, Gang Wang, Lianshan Yan, Xiangwei Kong, Qingtang Su, Caiming Zhang, and Qi Tian. 2019. Online latent semantic hashing for cross-media retrieval. Pattern Recognit., Vol. 89 (2019), 1--11. Google Scholar Cross Ref Dongqing Zhang and Wu Jun Li. 2014. Large-scale supervised multimodal hashing with semantic correlation maximization. In Proc. AAAI Conf. Artif. Intell. 2177--2183. Google Scholar
3394171.3413971.mp4
The topic of this presentation is "Label Embedding Online Hashing for Cross-Modal Retrieval". It first introduces the backgrounds of this work, and give the proposed method with two sub-parts, and experiments, finally, the conclusion. In our paper, we propose a novel label embedding online hashing method for cross-modal retrieval, termed as LEMON, which fully exploits the semantic labels by a well-designed label embedding framework. It also periodically considers the correlation between new and existing data by a block similarity matrix. Finally it solves the optimization problem through an efficient and discrete algorithm. Extensive experimental results on three benchmark datasets demonstrate that LEMON outperforms some state-of-the-art offline and online cross-modal hashing methods in terms of accuracy and efficiency.
Browse All Return Change zoom level
Close modal New Citation Alert added!
Connect
Contact
Facebook
Twitter
Linkedin
Feedback
Bug Report
The ACM Digital Library is published by the Association for Computing Machinery. Copyright © 2023 ACM, Inc.
If you 'd like us to contact you regarding your feedback, please provide your contact details here.
Supervised cross-modal hashing has gained a lot of attention recently. However, most existing methods learn binary codes or hash functions in a batch-based scheme, which is inefficient in an online scenario, i.e., data points come in a streaming fashion. Online hashing is a promising solution; however, there still exist several challenges, e.g., how to effectively exploit semantic information, how to discretely solve the binary optimization problem, how to efficiently update hash codes and hash functions. To address these issues, in this paper, we propose a novel supervised online cross-modal hashing method, i.e., Label EMbedding ONline hashing, LEMON for short. It builds a label embedding framework including label similarity preserving and label reconstructing, which may generate discriminative binary codes and reduce the computational complexity. Furthermore, it not only preserves the pairwise similarity of incoming data, but also establishes a connection between newly coming data and existing data by the inner product minimization on a block similarity matrix. In the light of this, it can exploit more similarity information and make the optimization less sensitive to incoming data, leading to effective binary codes. In addition, we design a discrete optimization algorithm to solve the binary optimization problem without relaxation. Therefore, the quantization error can be reduced. Moreover, its computational complexity is only relevant to the size of incoming data, making it very efficient and scalable to large-scale datasets. Extensive experimental results on three benchmark datasets demonstrate that LEMON outperforms some state-of-the-art offline and online cross-modal hashing methods in terms of accuracy and efficiency.
The topic of this presentation is "Label Embedding Online Hashing for Cross-Modal Retrieval". It first introduces the backgrounds of this work, and give the proposed method with two sub-parts, and experiments, finally, the conclusion. In our paper, we propose a novel label embedding online hashing method for cross-modal retrieval, termed as LEMON, which fully exploits the semantic labels by a well-designed label embedding framework. It also periodically considers the correlation between new and existing data by a block similarity matrix. Finally it solves the optimization problem through an efficient and discrete algorithm. Extensive experimental results on three benchmark datasets demonstrate that LEMON outperforms some state-of-the-art offline and online cross-modal hashing methods in terms of accuracy and efficiency.
Check if you have access through your login credentials or your institution to get full access on this article.
Chinese University of Hong Kong, Shenzhen, China
UNITN & Fondazione Bruno Kessler, Italy
National University of Singapore, Singapore
Association for Computing Machinery
Request permissions about this article.
View this article in digital edition.
https://dl.acm.org/doi/10.1145/3394171.3413971
This alert has been successfully added and will be sent to:
You will be notified whenever a record that you have chosen has been cited.
To manage your alert preferences, click on the button below.
We use cookies to ensure that we give you the best experience on our website.
Shortcuts zu anderen Sites, um außerhalb von DuckDuckGo zu suchen Mehr erfahren
Are fashion, art & decor your interests? Do you want to publish your own designs? URSTYLE offers you a new creative home and the best alternative for Polyfam!
Find local businesses, view maps and get driving directions in Google Maps.
3394171. 3413971 .mp4. The topic of this presentation is "Label Embedding Online Hashing for Cross-Modal Retrieval". It first introduces the backgrounds of this work, and give the proposed method with two sub-parts, and experiments, finally, the conclusion. In our paper, we propose a novel label embedding online hashing method for cross-modal ...
Hilf deinen Freunden und Verwandten, der Seite der Enten beizutreten!
Schütze Deine Daten, egal auf welchem Gerät.
Bleibe geschützt und informiert mit unseren Privatsphäre-Newslettern.
Wechsel zu DuckDuckGo und hole dir deine Privatsphäre zurück!
Benutze unsere Seite, die nie solche Nachrichten anzeigt:
Bleibe geschützt und informiert mit unseren Privatsphäre-Newslettern.
Links erforschen, um auf Suchergebnisse anderer Websites zu gelangen.
Hilf deinen Freunden und Verwandten, der Seite der Enten beizutreten!
Wir zeigen Ihnen, wie du deine Privatsphäre online besser schützen kannst.
Shortcuts zu anderen Sites, um außerhalb von DuckDuckGo zu suchen Mehr erfahren
Are fashion, art & decor your interests? Do you want to publish your own designs? URSTYLE offers you a new creative home and the best alternative for Polyfam!
Find local businesses, view maps and get driving directions in Google Maps.
3394171. 3413971 .mp4. The topic of this presentation is "Label Embedding Online Hashing for Cross-Modal Retrieval". It first introduces the backgrounds of this work, and give the proposed method with two sub-parts, and experiments, finally, the conclusion. In our paper, we propose a novel label embedding online hashing method for cross-modal ...
Hilf deinen Freunden und Verwandten, der Seite der Enten beizutreten!
Schütze Deine Daten, egal auf welchem Gerät.
Bleibe geschützt und informiert mit unseren Privatsphäre-Newslettern.
Wechsel zu DuckDuckGo und hole dir deine Privatsphäre zurück!
Benutze unsere Seite, die nie solche Nachrichten anzeigt:
Bleibe geschützt und informiert mit unseren Privatsphäre-Newslettern.
Links erforschen, um auf Suchergebnisse anderer Websites zu gelangen.
Hilf deinen Freunden und Verwandten, der Seite der Enten beizutreten!
Wir zeigen Ihnen, wie du deine Privatsphäre online besser schützen kannst.
Shortcuts zu anderen Sites, um außerhalb von DuckDuckGo zu suchen Mehr erfahren
Are fashion, art & decor your interests? Do you want to publish your own designs? URSTYLE offers you a new creative home and the best alternative for Polyfam!
Find local businesses, view maps and get driving directions in Google Maps.
3394171. 3413971 .mp4. The topic of this presentation is "Label Embedding Online Hashing for Cross-Modal Retrieval". It first introduces the backgrounds of this work, and give the proposed method with two sub-parts, and experiments, finally, the conclusion. In our paper, we propose a novel label embedding online hashing method for cross-modal ...
Hilf deinen Freunden und Verwandten, der Seite der Enten beizutreten!
Schütze Deine Daten, egal auf we
Зализы пизды крупным планом
Негритянка засандалила в жопу любовнице страпон
Беременная латинка безумно хочет ебаться