Галерея 3203993
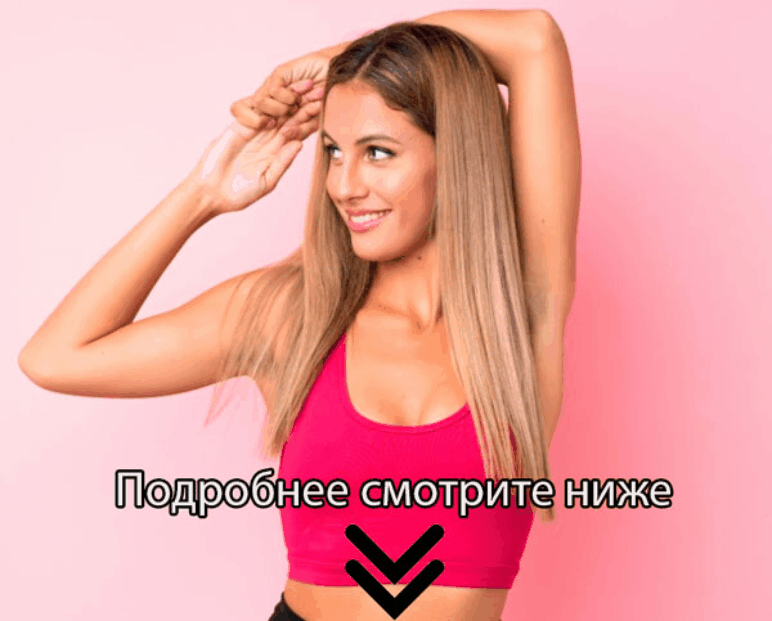
Галерея 3203993
To navigate to Skip menu use control + q shortcut.
Free cancellation up to 60 days before check-in
Beachfront Poolside Condo! Ground floor access, No stairs or elevator! IP 107
About Rooms & beds Amenities Reviews Map Host Policies Rates & availability
Okaloosa Island, Florida, United States of America
3. Emerald Coast Science Center 0.6 mi
4. Indian Temple Mound Museum 0.6 mi
6. Emerald Coast Convention Center 1 mi
Previous page 1 - 6 of 12 Next page
Gretchen Seamons is a Premier Host · They consistently provide great experiences for their guests
100% refund of amount paid if you cancel at least 60 days before check-in. 50% refund of amount paid (minus the service fee) if you cancel at least 30 days before check-in. No refund if you cancel less than 30 days before check-in. Free cancellation deadlines are in the property's timezone. Learn more about cancellation policies.
If you have upcoming trips, you can manage or cancel your booking in your traveler account. View upcoming trip
Brett and I own a leadership development firm in Milwaukee, Wisconsin. We have been married for 31 years. We have four children (28, 18, 15, and 13) and two grandchildren (3 and 6 months). We are so excited to share our beautiful beachfront condo with you! We hope that you enjoy the powdery sand and warm sunshine as you relax and take a break from your busy life! The condo has been completely renovated with all new ceramic hardwood floors, all new stainless steel appliances, and all new cabinets. The back door is poolside, and just 55 steps from the sand. Because we have young children and teenagers in our family, we know how great it is to go on vacation and stay somewhere where everything is provided for you! That's why we manage this property ourselves. We hope to anticipate your every need. We provide beach towels, a travel crib, high chair, stroller, baby monitor, beach toys, and kid plates, bowls & utensils. There are USB ports and TVs in every room. Just pack a swimsuit and come! I (Gretchen) manage the property. Give me a call or sent me a message with any questions you might have.
This condo unit is especially good for families with small children or older adults who do not want to climb stairs or wait for elevators. It is on the ground floor just steps away from the pool, hot tub, outdoor shower, and access to the beach. The patio is another living area and makes great storage for all of your sandy beach gear. It can be accessed directly from the pool area.
You will be responsible for any damage to the rental property caused by you or your party during your stay.
Maximum overnight guests: 9 (sleeps up to 6 adults) Check in after 4:00 PM
Check out before 10:00 AM
All towels and bedding washed in hot water that’s at least 60ºC/140ºF
High-touch surfaces cleaned with disinfectant (like countertops, light switches, handles, and faucets)
Follows industry health association: COVID-19 Guidelines (CDC)
Update your browser for a better experience.
Online Sequencer
Make music online
1
1/2
1/3
1/4
1/6
1/8
1/12
1/16
2/4
3/4
4/4
5/4
Smooth
Fast
Off
Filter recorded notes using the key guide.
Play a metronome. When recording, gives a 4 beat lead in.
OnlineSequencer.net is an online music sequencer. Make tunes in your browser and share them with friends!
Made by Jacob Morgan and George Burdell
·
Hosting 3,144,550 sequences since 2013 ·
Buy me a ☕
·
Hong Kong authorities hope free screenings will encourage residents to visit the new district centres. Photo: Shutterstock
Residents will be encouraged to visit health centres planned for each district to undergo free screening for diabetes and hypertension
For those deemed at high risk, government will cover half their bill for treatment by private doctors, Undersecretary for Health Libby Lee says
Hong Kong authorities hope free screenings will encourage residents to visit the new district centres. Photo: Shutterstock
All Books Conferences Courses Journals & Magazines Standards Authors Citations
Atmospheric Simulation and Network Setup
Numerical Simulation and Discussion
Abstract: Correction performance of an adaptive optics (AO) system is severely limited by its system latency under high temporal frequency distortions. Wavefront prediction methods... View more
Correction performance of an adaptive optics (AO) system is severely limited by its system latency under high temporal frequency distortions. Wavefront prediction methods has been proven to be an effective way to compensate system delay. A novel wavefront prediction method based on residual learning fusion network (RLFNet) is proposed in this paper. The network is able to eliminate redundant information between adjacent wavefront frames and fuse refined features, which provides a higher prediction accuracy under fewer priori wavefront input. The method is tested on a 1km laser atmospheric transmission experimental setup through high frequency atmospheric turbulence, where wavefront data is collected from a 1kHz Shack-Hartmann wavefront sensor (SHWS). We show that the proposed architecture is able to predict the second frame wavefront after the previous 6 frames with a root-mean-square (RMS) wavefront error reduction up to 53% compare to non-predictive method.
Published in: IEEE Photonics Journal ( Volume: 14 , Issue: 5 , October 2022 )
Date of Publication: 05 September 2022
TABLE I Simulation Data Results Display
TABLE II Comparison of the RMSe/RMS_Ture Results By Different Modules in Ablation Studies
TABLE III This is a Sample of a Table Title
TABLE IV Experimental Data Results Display
G. Sivo, C. Kulcsár and J.-M. Conan, "First on-sky SCAO validation of full LQG control with vibration mitigation on the CANARY pathfinder", Opt. Exp. , vol. 22, no. 19, pp. 23565-23591, 2014.
D. L. Fried, "Time-delay-induced mean-square error in adaptive optics", JOSA A , vol. 7, no. 7, pp. 1224-1225, Jul. 1990.
C. Kulcsár, H.-F. Raynaud and C. Petit, "Minimum variance prediction and control for adaptive optics", automatica , vol. 48, no. 9, pp. 1939-1954, Sep. 2012.
Z. J. Yan, X. Y. Li and C. H. Rao, "Numerical simulation of a prediction control algorithm for close-loop adaptive optical system", Acta Optica Sinica , vol. 31, no. 1, Jan. 2011.
C. Liu, L. F. Hu and Q. Q. Mu, "Modal prediction for open-loop liquid-crysta adaptive optics systems", Acta Physica Sinica , vol. 61, no. 12, 2012.
M. D. Wiberg, C. E. Max and D. T. Gavel, "A spatial non-dynamic LQG controller: Part I application to adaptive optics", Proc. 43rd IEEE Conf. Decis. Control , pp. 3326-3332, 2004.
M. D. Wiberg, C. E. Max and D. T. Gavel, "A spatial non-dynamic LQG controller: Part II theory", Proc. 43rd IEEE Conf. Decis. Control , pp. 3333-3338, 2004.
P. Douglas, "Linear-quadratic-Gaussian control for adaptive optics systems using a hybrid model", JOSA A , vol. 26, no. 1, pp. 1-9, Dec. 2009.
C. Petit et al., "Kalman-filter-based control for adaptive optics", Proc. Advancements Adaptive Opt. , pp. 1414-1425, Oct. 2004.
M. Gray et al., "Local ensemble transform Kalman filter a fast non-stationary control law for adaptive optics on ELTs: Theoretical aspects and first simulation results", Opt. Exp.. , vol. 22, no. 17, pp. 20894-20913, Aug. 2014.
P. Massioni, C. Kulcsár, H.-F. Raynaud and J.-M. Conan, "Fast computation of an optimal controller for large-scale adaptive optics", J. Opt. Soc. Amer. A , vol. 28, no. 11, pp. 2298-2309, Oct. 2011.
L. Poyneer, B. A. Macintosh and J. Véran, "Fourier transform wavefront control with adaptive prediction of the atmosphere", JOSA A , vol. 24, no. 9, pp. 2645-2660, Jul. 2007.
L. C. Johnson, D. T. Gavel and D. M. Wiberg, "Bulk wind estimation and prediction for adaptive optics control systems", JOSA A , vol. 28, no. 8, pp. 1566-1577, Jul. 2011.
O. Guyon and J. Males, "Adaptive optics predictive control with empirical orthogonal functions (EOFs)", Jul. 2017.
L. Poyneer and J.-P. Véran, "Predictive wavefront control for adaptive optics with arbitrary control loop delays", JOSA A , vol. 28, no. 8, pp. 1486-1496, Jun. 2008.
X. Zhou and X. Y. Li, "Wavefront distortion prediction method based on motion estimation", Opto-Electron. Eng. , vol. 48, no. 10, 2021.
M. A. Chen, X. Q. Jin, S. B. Li and Z. Y. Xu, "Compensation of turbulence-induced wavefront aberration with convolutional neural networks for FSO systems", Chin. Opt. Lett. , vol. 19, no. 11, Nov. 2021.
Y. M. Guo et al., "Adaptive optics based on machine learning: A review", Opto-Electron. Adv. , vol. 5, 2022.
J. G. Chen, V. Shah and L. L. Liu, "Performance of a U-Net-based neural network for predictive adaptive optics", Opt. Lett. , vol. 46, no. 10, pp. 2513-2516, May 2021.
X. Y. Shi, Y. Feng and Y. Chen, "Predicting control voltages of deformable mirror in adaptive optics system", High Power Laser Part. Beams , vol. 24, no. 6, pp. 1281-1286, Jun. 2012.
Z. J. Yan and X. Y. Li, "Neural network prediction algorithm for control voltage of deformable mirror in adaptive optical system", Acta Optica Sinica , vol. 30, no. 4, pp. 911-916, Apr. 2010.
Y. Chen, "Voltages prediction algorithm based on LSTM recurrent neural network", Optik , vol. 220, no. 16, pp. 4869-4880, Oct. 2020.
P. Lara-Benítez, M. Carranza-García and J. C. Riquelme, "An experimental review on deep learning architectures for time series forecasting", Int. J. Neural Syst. , vol. 31, no. 3, Feb. 2021.
S. Matthias and J. Earl, "Method for a quantitative investigation of the frozen flow hypothesis", JOSA A , vol. 17, no. 9, pp. 1650-1658, Sep. 2000.
L. Poyneer, M. V. Dam and J. P. Véran, "Experimental verification of the frozen flow atmospheric turbulence assumption with use of astronomical adaptive optics telemetry", JOSA A , vol. 26, no. 4, pp. 833-846, Mar. 2009.
E. Gendron and P. Léna, "Single layer atmospheric turbulence demonstrated by adaptive optics observations", Astrophys. Space Sci. , vol. 239, no. 2, pp. 221-228, Sep. 1996.
B. Kern, T. A. Laurence, C. Martin and P. E. Dimotakis, "Temporal coherence of individual turbulent patterns in atmospheric seeing", Appl. Opt. , vol. 39, no. 27, pp. 4879-4885, Sep. 2000.
Z. H. Li and X. Y. Li, "Performance of predictive correction foe adaptive optics systems with frozen flow turbulence", Opt. Precis. Eng. , vol. 26, no. 3, pp. 548-555, 2018.
A. N. Kolmogorov, "The local structure of turbulence in incompressible viscous fluid for very large reynolds numbers[J]", Proc. Roy. Soc. London. Ser. A: Math. Phys. Sci. , vol. 434, no. 1890, pp. 9-13, 1991.
J. Y. Wang, X. C. Liu, R. Z. Rao and Z. B. Gong, "Measurement of atmospheric coherence length in daytime and at night", High Power Laser Part. Beams , vol. 16, no. 1, pp. 1-4, 2004.
W. D. Xiang, P. Yang, S. Wang, B. Xu and H. Liu, "Underwater image enhancement based on red channel weighted compensation and gamma correction model", Opto-Electron. Adv. , vol. 1, 2018.
K. He, X. Zhang, S. Ren and J. Sun, "Delving deep into rectifiers: Surpassing human-level performance on imagenet classification", Proc. IEEE Int. Conf. Comput. Vis. , pp. 1026-1034, 2015.
K. Simonyan and A. Zisserman, "Very deep convolutional networks for large-scale image recognition", 2014.
S. Ma, N. Wang, L. C. Zhu, W. Shuai, Y. Ping and X. Bing, "Light field depth estimation using weighted side window angular coherence[J]", Opto-Electron. Eng. , vol. 2021, no. 12, 2021.
X. Shi, Z. Chen, H. Wang, D. Yeung, W. Wong and W. Woo, "Convolutional LSTM network: A machine learning approach for precipitation nowcasting", Adv. Neural Inf. Process. Syst. , vol. 28, pp. 802-810, 2015.
G. Sun, N. Q. Weng and L. M. Xiao, "Statistical characteristics of vertical distribution of atmospheric strucure constant of refractive index Cn2", J. Atmos. Environ. Opt. , vol. 6, no. 2, Mar. 2011.
O. Soloviev and G. Vdovin, "Hartmann-Shack test with random masks for modal wavefront reconstruction", Opt. Exp. , vol. 13, no. 23, pp. 9570-9583, Nov. 2005.
R. Girshick, J. Donahue, T. Darrell and J. Malik, "Rich feature hierarchies for accurate object detection and semantic segmentation", Proc. IEEE Conf. Comput. Vis. Pattern Recognit. , pp. 580-587, 2014.
B. Biswas, R. Mukherjee and I. Chakrabarti, "A high-speed VLSI architecture for motion estimation using modified adaptive rood pattern search algorithm", Circuits Syst. Signal Process. , vol. 37, no. 10, pp. 4548-4567, 2018.
IEEE Account
Change Username/Password
Update Address
Purchase Details
Payment Options
Order History
View Purchased Documents
Need Help?
US & Canada: +1 800 678 4333
Worldwide: +1 732 981 0060
Contact & Support
Suppressing the degrading effect of atmospheric turbulence on laser beam transmission has been a major research focus in the fields of laser communications, and laser detection among others. AO technique is an effective way to compensate atmospheric turbulence in real time. However, real AO systems usually assume that the distorted wavefront is static during a control period, which leads to a servo-lag error of 2-3 sampling periods in the control loop [1] , [2] , [3] . The time delay in the loop mainly comes from signal processing and control tasks such as wavefront sensor data acquisition, wavefront reconstruction and control operation. Such time delay will cause the compensation wavefront on the deformable mirror (DM) lag behind the actual measured wavefront, which severely limits the correction performance of the AO system.
A large number of prediction methods have been studied to compensate the time delay. Among these prediction methods, the iterative prediction method like Recursive Least Squares (RLS) algorithm is to minimize the systematic error, which provides a simple calculation process and avoids to fall into the local minimum point. However, when the mode order of wavefront aberrations is too high, it cannot converge in a short time [4] , [5] . Model-based prediction methods use atmospheric turbulence model to predict the change of wavefront, such as the Linear Quadratic Gaussian control algorithm, which has a good approximation performance, but it is difficult to balance the prediction accuracy of the model between the control complexity of the controller [6] , [7] , [8] . In addition, there are more complex Kalman Filter based methods [9] , [10] , [11] , [12] and extensions of various approach [13] , [14] , [15] , [16] . In fact, the majority of these algorithms can be described as analytical applications of various estimation frameworks. But amid a large wave of general advances in Neural-network (NN), NN-based methods have been widely used for wavefront reconstruction and prediction of AO systems [17] , [18] . It turns out that NN-based methods have the advantage over analytical estimation techniques in terms of alleviating time delay, because NN predictors are self-optimizing, generalizable and have strong nonlinear fitting ability [18] , [19] . Shi Xiaoyu [20] and Yan Zaojun [21] et al. used a two-layer back-propagation (BP) neural network prediction algorithm to predict the open-loop control voltage of the DM, and the correction effect was about 30 times better than that of the RLS algorithm for the same wind speed. In 2020, Chen Ying et al. used Long Short-term Memory (LSTM) network for voltage prediction, and its prediction residual was about 8 times lower than that of BP network [22] .
In terms of network input and output types, direct prediction of the wavefront can be more advantageous, because the prediction model is largely unaffected by changes in SHWS and DM, thus, there is no need to design specific network models for different AO systems. Therefore, the way of directly predicting the wavefront has higher compatibility and applicability in most of AO systems. From the perspective of network model, for dealing with the time series prediction problem of wavefront prediction, LSTM network has more advantages under the same environment configuration [23] . This is because LSTM network has a strong memory ability and can accurately learn the temporal features between successive distorted wavefront frames to meet the prediction requirements. However, due to the temporal features of continuous wavefront, the direct feature extraction from the unrefined continuous wavefront will cause redundant errors, which damages the prediction accuracy. Therefore, a deep learning-based RLFNet is proposed for the first time in this paper, which eliminates the redundant information between frames by means of residual learning, then extracts the refined features for fusion, and fuses the features at all levels again to obtain a high accuracy prediction result. The prediction performance of our proposed RLFNet is tested from simulation and experiment results under different types of atmospheric turbulence conditions.
The atmospheric frozen flow hypothesis is a widely adopted modeling approach for atmospheric turbulence that has been verified by a large number of experiments [24] , [25] , [26] , [27] . It has been shown that the time scale on which the frozen flow hypothesis holds is 10∼20 ms, reaching 50∼100 ms in a few cases [28] . In the AO system with a sampling frequency of more than 1 kHz, the time delay of the system is basically less than 3 ms, so the frozen flow hypothesis in such a system is reasonable.
Among them, generally take: v\ = \ 21m/s , A\ = \ 1.7 \times {10}^{ - 14} . The formula shows that atmospheric turbulence is the strongest at near the ground. As the altitude increases, C_n^2 experiences a process that will first become weaker, then stronger, and finally gradually weaken.
Where k is the wavenumber, L is the transmission distance, and \varphi is the zenith angle.
Among them, generally take: {v}_g = \ 5 \, m/s , {v}_t = \ 30 \, m/s , {h}_{pk} = \ 9400 , {h}_{scale} = \ 4800 .
In this paper, the simulation data is generated by the time evolution of the Fourier series method based on the above atmospheric turbulence theory. We simulate the distorted wavefront according to the experimental scene of the 1 km near-ground laser atmospheric near-horizontal transmission system. Since the recovery accuracy of SHWS is limited, the phase screen has more detailed phase structure information than the wavefront recovered by SHWS, therefore, we use the phase screen as the training data of our proposed RLFNet, which can improve the generalization of the network model.
The principle of our proposed RLFNet applied to the AO system is shown in Fig. 1 . The SHWS is used to detect the slope information of the wavefront, and then after wavefront reconstruction, the control signal is output by the control system with prediction model to drive the DM to produce deformation, so as to compensate the actual distorted wavefront. In this paper, we focus only on the implementation of the wavefront prediction neural network model, which belongs to the open-loop prediction process, so the present study procedure is shown by the solid black arrows.
AO system with wavefront prediction model.
Wh
Киара сует в свою бритую пизду пальцы
Знаменитая британская порно звезда показывает свою большую грудь
Жена смазала жопу маслом и готова к аналу