Галерея 3119325
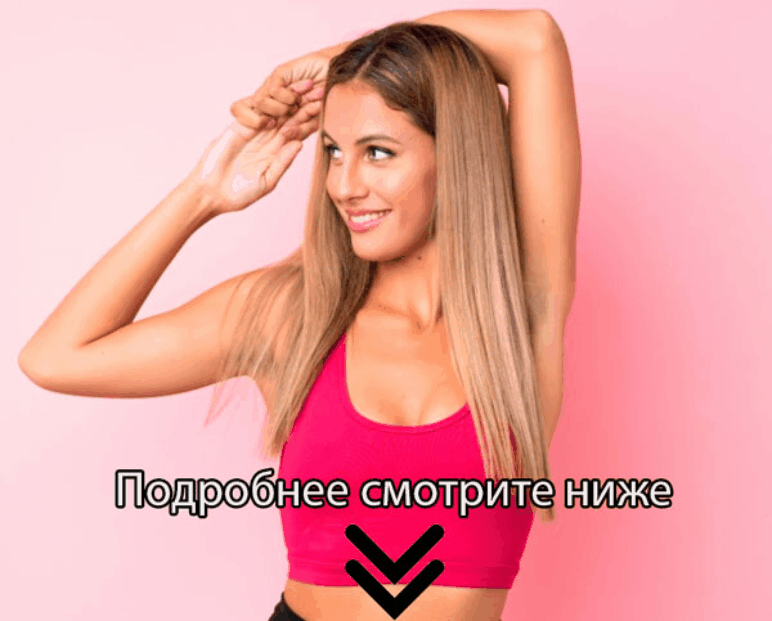
⚡ ПОДРОБНЕЕ ЖМИТЕ ЗДЕСЬ 👈🏻👈🏻👈🏻
Галерея 3119325
Clipboard, Search History, and several other advanced features are temporarily unavailable.
Dashboard
Publications
Account settings
Log out
Advanced
Clipboard
Format
Abstract
PubMed
PMID
Format:
Summary (text)
PubMed
PMID
Abstract (text)
CSV
Subject:
1 selected item: 34637384 - PubMed
Format:
Summary
Summary (text)
Abstract
Abstract (text)
Create a new collection
Add to an existing collection
Name must be less than 100 characters
Unable to load your collection due to an error
Please try again
Unable to load your delegates due to an error
Please try again
Would you like email updates of new search results?
Saved Search Alert Radio Buttons
Yes
No
Frequency:
Monthly
Weekly
Daily
Which day?
The first Sunday
The first Monday
The first Tuesday
The first Wednesday
The first Thursday
The first Friday
The first Saturday
The first day
The first weekday
Which day?
Sunday
Monday
Tuesday
Wednesday
Thursday
Friday
Saturday
Report format:
Summary
Summary (text)
Abstract
Abstract (text)
PubMed
Send at most:
1 item
5 items
10 items
20 items
50 items
100 items
200 items
Send even when there aren't any new results
Number of items displayed:
5
10
15
20
50
100
Create a new collection Add to an existing collection
Name must be less than 100 characters
Unable to load your collection due to an error Please try again
Page navigation
Title & authors
Abstract
Figures
Similar articles
Cited by
References
MeSH terms
Related information
Grant support
LinkOut - more resources
Yuexiang Li et al.
IEEE J Biomed Health Inform .
2022 Jan .
Format
Abstract
PubMed
PMID
The data bias problem: certain sources of data may comprise only a single class of data ( e.g., Source 2 has only positive cases (COVID-19), whereas Sources 1 and 3 have only negative cases—non-pneumonia and community acquired pneumonia (CAP), respectively), introducing a source-related bias in network training. The data bias problem occurred in reality while developing the CAD for COVID-19. Hospitals exclusively designated for accommodation of COVID-19 cases (such as the emergency specialty field hospitals built in Wuhan, China) provide mostly COVID-19 scans, whereas a physical examination institution provides mostly non-pneumonia cases, and the cases from conventional hospitals are usually CAP. Models trained with such multi-source data without dealing with the source bias cannot generalize to the data collected from an unseen centre (Source 4).
Illustration of the proposed MINI. The proposed approach consists of three steps—mix, trilinear interpolation and nearest interpolation. A hyperparameter is used to control the amount of information to fuse along the z-axis.
Visualization of samples generated by different augmentation approaches. The samples generated by Cutout and CutMix face the risk of mistakenly discarding the lesion areas. Mixup and our MINI can alleviate the problem—maintaining the lesion areas via voxel-summation and trilinear interpolation, respectively.
Training curves for models using existing data augmentation approaches and our MINI. The model with MINI is observed to better converge ( i.e., more stable with the lower loss values).
Comparison of t-SNE between models without (a) and with MINI (b). Our MINI is observed to compact the cluster of negative in the feature space.
The of COVID-19 identification yielded by networks trained with data collected from different numbers of centres. Here, soft labeling is adopted to yield the labels for generated volumes.
Visualization of the activation maps of neural network for COVID-19 samples. The annotations provided by radiologists are drawn with red rectangles.
Li Y, Wei D, Chen J, Cao S, Zhou H, Zhu Y, Wu J, Lan L, Sun W, Qian T, Ma K, Xu H, Zheng Y.
Li Y, et al.
IEEE J Biomed Health Inform. 2020 Oct;24(10):2787-2797. doi: 10.1109/JBHI.2020.3018181. Epub 2020 Aug 20.
IEEE J Biomed Health Inform. 2020.
PMID: 32816680
V J S, D JF.
V J S, et al.
Comput Math Methods Med. 2021 Nov 9;2021:9269173. doi: 10.1155/2021/9269173. eCollection 2021.
Comput Math Methods Med. 2021.
PMID: 34795794
Free PMC article.
Ko H, Chung H, Kang WS, Kim KW, Shin Y, Kang SJ, Lee JH, Kim YJ, Kim NY, Jung H, Lee J.
Ko H, et al.
J Med Internet Res. 2020 Jun 29;22(6):e19569. doi: 10.2196/19569.
J Med Internet Res. 2020.
PMID: 32568730
Free PMC article.
Khan AI, Shah JL, Bhat MM.
Khan AI, et al.
Comput Methods Programs Biomed. 2020 Nov;196:105581. doi: 10.1016/j.cmpb.2020.105581. Epub 2020 Jun 5.
Comput Methods Programs Biomed. 2020.
PMID: 32534344
Free PMC article.
Li C, Yang Y, Liang H, Wu B.
Li C, et al.
Knowl Based Syst. 2021 Apr 22;218:106849. doi: 10.1016/j.knosys.2021.106849. Epub 2021 Feb 6.
Knowl Based Syst. 2021.
PMID: 33584016
Free PMC article.
Alrebdi N, Alabdulatif A, Iwendi C, Lian Z.
Alrebdi N, et al.
Sci Rep. 2022 Jan 7;12(1):266. doi: 10.1038/s41598-021-04124-8.
Sci Rep. 2022.
PMID: 34997109
Free PMC article.
Related information
MedGen
This work was supported by the Key-Area Research and Development Program of Guangdong Province, China under grant 2018B010111001, National Key R&D Program of China under grant 2018YFC2000702, and the Scientific and Technical Innovation 2030- “New Generation Artificial Intelligence” Project under grant 2020AAA0104100.
Format:
AMA
APA
MLA
NLM
Send To
Clipboard
Email
Save
My Bibliography
Collections
Citation Manager
[x]
NLM
NIH
HHS
USA.gov
An official website of the United States government
The .gov means it’s official.
Federal government websites often end in .gov or .mil. Before
sharing sensitive information, make sure you’re on a federal
government site.
The site is secure.
The https:// ensures that you are connecting to the
official website and that any information you provide is encrypted
and transmitted securely.
Till March 31st, 2021, the coronavirus disease 2019 (COVID-19) had reportedly infected more than 127 million people and caused over 2.5 million deaths worldwide. Timely diagnosis of COVID-19 is crucial for management of individual patients as well as containment of the highly contagious disease. Having realized the clinical value of non-contrast chest computed tomography (CT) for diagnosis of COVID-19, deep learning (DL) based automated methods have been proposed to aid the radiologists in reading the huge quantities of CT exams as a result of the pandemic. In this work, we address an overlooked problem for training deep convolutional neural networks for COVID-19 classification using real-world multi-source data, namely, the data source bias problem. The data source bias problem refers to the situation in which certain sources of data comprise only a single class of data, and training with such source-biased data may make the DL models learn to distinguish data sources instead of COVID-19. To overcome this problem, we propose MIx-aNd-Interpolate (MINI), a conceptually simple, easy-to-implement, efficient yet effective training strategy. The proposed MINI approach generates volumes of the absent class by combining the samples collected from different hospitals, which enlarges the sample space of the original source-biased dataset. Experimental results on a large collection of real patient data (1,221 COVID-19 and 1,520 negative CT images, and the latter consisting of 786 community acquired pneumonia and 734 non-pneumonia) from eight hospitals and health institutions show that: 1) MINI can improve COVID-19 classification performance upon the baseline (which does not deal with the source bias), and 2) MINI is superior to competing methods in terms of the extent of improvement.
The data bias problem: certain sources of data may comprise only a single…
Illustration of the proposed MINI.…
Illustration of the proposed MINI. The proposed approach consists of three steps—mix, trilinear…
Visualization of samples generated by…
Visualization of samples generated by different augmentation approaches. The samples generated by Cutout…
Training curves for models using existing data augmentation approaches and our MINI. The…
Comparison of t-SNE between models…
Comparison of t-SNE between models without (a) and with MINI (b). Our MINI…
The of COVID-19 identification yielded…
The of COVID-19 identification yielded by networks trained with data collected from different…
Visualization of the activation maps…
Visualization of the activation maps of neural network for COVID-19 samples. The annotations…
MeSH
PMC
Bookshelf
Disclaimer
The PubMed wordmark and PubMed logo are registered trademarks of the U.S. Department of Health and Human Services (HHS). Unauthorized use of these marks is strictly prohibited.
Help
Accessibility
Careers
All Books Conferences Courses Journals & Magazines Standards Authors Citations
Abstract: While high-resolution pathology images lend themselves well to ‘data hungry’ deep learning algorithms, obtaining exhaustive annotations on these images for learning is a ... View more
While high-resolution pathology images lend themselves well to ‘data hungry’ deep learning algorithms, obtaining exhaustive annotations on these images for learning is a major challenge. In this article, we propose a self-supervised convolutional neural network (CNN) framework to leverage unlabeled data for learning generalizable and domain invariant representations in pathology images. Our proposed framework, termed as Self-Path, employs multi-task learning where the main task is tissue classification and pretext tasks are a variety of self-supervised tasks with labels inherent to the input images. We introduce novel pathology-specific self-supervision tasks that leverage contextual, multi-resolution and semantic features in pathology images for semi-supervised learning and domain adaptation. We investigate the effectiveness of Self-Path on 3 different pathology datasets. Our results show that Self-Path with the pathology-specific pretext tasks achieves state-of-the-art performance for semi-supervised learning when small amounts of labeled data are available. Further, we show that Self-Path improves domain adaptation for histopathology image classification when there is no labeled data available for the target domain. This approach can potentially be employed for other applications in computational pathology, where annotation budget is often limited or large amount of unlabeled image data is available.
Published in: IEEE Transactions on Medical Imaging ( Volume: 40 , Issue: 10 , October 2021 )
Date of Publication: 01 February 2021
References is not available for this document.
IEEE Account
Change Username/Password
Update Address
Purchase Details
Payment Options
Order History
View Purchased Documents
Need Help?
US & Canada: +1 800 678 4333
Worldwide: +1 732 981 0060
Contact & Support
The recent surge in the area of computational pathology can be attributed to the increasing ubiquity of digital slide scanners and the consequent rapid rise in the amount of raw pixel data acquired by scanning of histology slides into digital whole-slide images (WSIs). These developments make the area of computational pathology ripe ground for deep neural network (DNN) models. In recent years, there have been notable successes in training DNNs for pathology image analysis and automated diagnosis of disease in the histopathology domain [1]. The performance and generalizability of most DNNs is, however, highly dependent on the availability of large and diverse amounts of annotated data. Although the use of digital slide scanners have made large amounts of raw data available, development of DNN based algorithms remains bottlenecked by the need for extensive annotations on diverse datasets.
2020 IEEE 17th International Symposium on Biomedical Imaging (ISBI)
A not-for-profit organization, IEEE is the world's largest technical professional organization dedicated to advancing technology for the benefit of humanity.
© Copyright 2023 IEEE - All rights reserved.
A not-for-profit organization, IEEE is the world's largest technical professional organization dedicated to advancing technology for the benefit of humanity. © Copyright 2023 IEEE - All rights reserved. Use of this web site signifies your agreement to the terms and conditions.
Shortcuts zu anderen Sites, um außerhalb von DuckDuckGo zu suchen Mehr erfahren
3120 Gallery. 2021 г. Санкт-Петербург. 3120 Gallery — галерея современного искусства, расположенная в самом сердце Петроградской стороны и представляющая проекты молодых российских и зарубежных ...
Русский олигарх ебет жопу красивой сучки. Молодуха с сочной задницей и в черной футболке легла на живот, чтобы поклонник отодрал ее
Mix-and-Interpolate: A Training Strategy to Deal with Source-biased Medical Data. IEEE J Biomed Health Inform. 2021 Oct 12;PP. doi: 10.1109/JBHI.2021. 3119325 .
While high-resolution pathology images lend themselves well to 'data hungry' deep learning algorithms, obtaining exhaustive annotations on these images for learning is a major challenge. In this article, we propose a self-supervised convolutional neural network (CNN) framework to leverage unlabeled data for learning generalizable and domain invariant representations in pathology images ...
Hilf deinen Freunden und Verwandten, der Seite der Enten beizutreten!
Schütze Deine Daten, egal auf welchem Gerät.
Bleibe geschützt und informiert mit unseren Privatsphäre-Newslettern.
Wechsel zu DuckDuckGo und hole dir deine Privatsphäre zurück!
Benutze unsere Seite, die nie solche Nachrichten anzeigt:
Du hast die Kontrolle. Passe das Aussehen von DuckDuckGo an.
Erfahre, wie wir uns dafür einsetzen, dass du online sicher sind.
Erfahre, wie du dich für immer von Google befreien kannst.
Wir zeigen Ihnen, wie du deine Privatsphäre online besser schützen kannst.
Shortcuts zu anderen Sites, um außerhalb von DuckDuckGo zu suchen Mehr erfahren
3120 Gallery. 2021 г. Санкт-Петербург. 3120 Gallery — галерея современного искусства, расположенная в самом сердце Петроградской стороны и представляющая проекты молодых российских и зарубежных ...
Русский олигарх ебет жопу красивой сучки. Молодуха с сочной задницей и в черной футболке легла на живот, чтобы поклонник отодрал ее
Mix-and-Interpolate: A Training Strategy to Deal with Source-biased Medical Data. IEEE J Biomed Health Inform. 2021 Oct 12;PP. doi: 10.1109/JBHI.2021. 3119325 .
While high-resolution pathology images lend themselves well to 'data hungry' deep learning algorithms, obtaining exhaustive annotations on these images for learning is a major challenge. In this article, we propose a self-supervised convolutional neural network (CNN) framework to leverage unlabeled data for learning generalizable and domain invariant representations in pathology images ...
Hilf deinen Freunden und Verwandten, der Seite der Enten beizutreten!
Schütze Deine Daten, egal auf welchem Gerät.
Bleibe geschützt und informiert mit unseren Privatsphäre-Newslettern.
Wechsel zu DuckDuckGo und hole dir deine Privatsphäre zurück!
Benutze unsere Seite, die nie solche Nachrichten anzeigt:
Du hast die Kontrolle. Passe das Aussehen von DuckDuckGo an.
Erfahre, wie wir uns dafür einsetzen, dass du online sicher sind.
Erfahre, wie du dich für immer von Google befreien kannst.
Wir zeigen Ihnen, wie du deine Privatsphäre online besser schützen kannst.
Черные мужчины влюбленные в белых дам
Секс на лужайке с великолепной блядью Esperanza Gomez
Шалава блондинка может кончать струей