Галерея 3086151
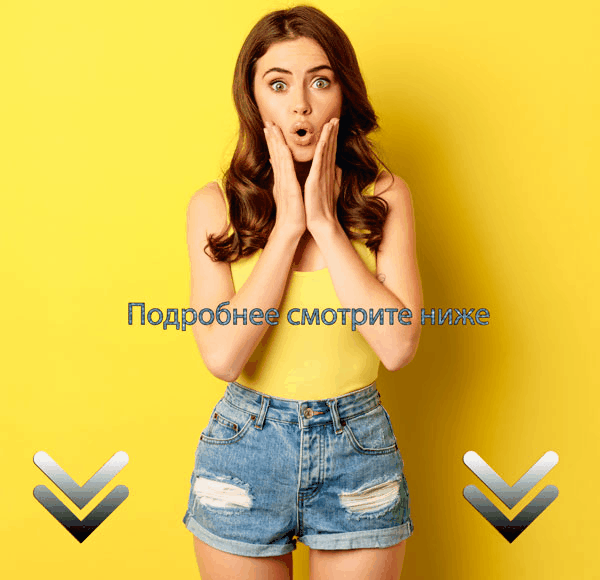
🛑 ПОДРОБНЕЕ ЖМИТЕ ЗДЕСЬ 👈🏻👈🏻👈🏻
Галерея 3086151
You do not have access to www.partzilla.com.
The site owner may have set restrictions that prevent you from accessing the site.
Provide the site owner this information.
I got an error when visiting www.partzilla.com/product/polaris/3086151.
Performance & security by Cloudflare
National Center for Biotechnology Information
But-2-enedioic acid--2-methyl-1,2,3,4,4a,9-hexahydrodibenzo[c,f]pyrimido[1,6-a]azepine (1/1)
4 Related Records Expand this section
5 Classification Expand this section
Computed by Lexichem TK 2.7.0 (PubChem release 2021.05.07)
Computed by InChI 1.0.6 (PubChem release 2021.05.07)
Computed by InChI 1.0.6 (PubChem release 2021.05.07)
Computed by OEChem 2.3.0 (PubChem release 2021.05.07)
Computed by PubChem 2.1 (PubChem release 2021.05.07)
EPA DSSTox; European Chemicals Agency (ECHA)
But-2-enedioic acid--2-methyl-1,2,3,4,4a,9-hexahydrodibenzo[c,f]pyrimido[1,6-a]azepine (1/1)
Use of the information, documents and data from the ECHA website is subject to the terms and conditions of this Legal Notice, and subject to other binding limitations provided for under applicable law, the information, documents and data made available on the ECHA website may be reproduced, distributed and/or used, totally or in part, for non-commercial purposes provided that ECHA is acknowledged as the source: "Source: European Chemicals Agency, http://echa.europa.eu/". Such acknowledgement must be included in each copy of the material. ECHA permits and encourages organisations and individuals to create links to the ECHA website under the following cumulative conditions: Links can only be made to webpages that provide a link to the Legal Notice page.
(±)-1,2,3,4,4a,9-hexahydro-2-methyldibenzo[c,f]pyrimido[1,6-a]azepine fumarate
Data: CC-BY 4.0; Code (hosted by ECI, LCSB): Artistic-2.0
NORMAN Suspect List Exchange Classification
8600 Rockville Pike , Bethesda , MD , 20894 USA
PubChem ® is a registered trademark of the National Library of Medicine
But-2-enedioic acid--2-methyl-1,2,3,4,4a,9-hexahydrodibenzo[c,f]pyrimido[1,6-a]azepine (1/1)
but-2-enedioic acid;4-methyl-2,4-diazatetracyclo[13.4.0.0 2,7 .0 8,13 ]nonadeca-1(19),8,10,12,15,17-hexaene
InChI=1S/C18H20N2.C4H4O4/c1-19-11-10-18-16-8-4-2-6-14(16)12-15-7-3-5-9-17(15)20(18)13-19;5-3(6)1-2-4(7)8/h2-9,18H,10-13H2,1H3;1-2H,(H,5,6)(H,7,8)
CN1CCC2C3=CC=CC=C3CC4=CC=CC=C4N2C1.C(=CC(=O)O)C(=O)O
Computed by PubChem 2.1 (PubChem release 2021.05.07)
Computed by Cactvs 3.4.8.18 (PubChem release 2021.05.07)
Computed by Cactvs 3.4.8.18 (PubChem release 2021.05.07)
Computed by Cactvs 3.4.8.18 (PubChem release 2021.05.07)
Computed by PubChem 2.1 (PubChem release 2021.05.07)
Computed by PubChem 2.1 (PubChem release 2021.05.07)
Computed by Cactvs 3.4.8.18 (PubChem release 2021.05.07)
Computed by Cactvs 3.4.8.18 (PubChem release 2021.05.07)
Computed by PubChem (release 2019.01.04)
All Books Conferences Courses Journals & Magazines Standards Authors Citations
The Proposed Cross-Domain I-ReliefF
Abstract: In the classification of hyperspectral images (HSIs), too many spectral bands (features) cause feature redundancy, resulting in a reduction in classification accuracy. In... View more
In the classification of hyperspectral images (HSIs), too many spectral bands (features) cause feature redundancy, resulting in a reduction in classification accuracy. In order to solve this problem, it is a good method to use feature selection to search for a feature subset which is useful for classification. Iterative ReliefF (I-ReliefF) is a traditional single-scene-based algorithm, and it has good convergence, efficiency, and can handle feature selection problems well in most scenes. Most single-scene-based feature selection methods perform poorly in some scenes (domains) which lack labeled samples. As the number of HSIs increases, the cross-scene feature selection algorithms which utilize two scenes to deal with the high dimension and low sample size problem are more and more desired. The spectral shift is a common problem in cross-scene feature selection. It leads to difference in spectral feature distribution between source and target scenes even though these scenes are highly similar. To solve the above problems, we extend I-ReliefF to a cross-scene algorithm: cross-domain I-ReliefF (CDIRF). CDIRF includes a cross-scene rule to update feature weights, which considers the separability of different land-cover classes and the consistency of the spectral features between two scenes. So CDIRF can effectively utilize the information of source scene to improve the performance of feature selection in target scene. The experiments are conducted on three cross-scene datasets for verification, and the experimental results demonstrate the superiority and feasibility of the proposed algorithm.
TABLE I Number of Labeled Samples in Each Land-Cover Class Within EShanghai-EHangzhou Dataset and the Number of Used Samples
TABLE II Number of Labeled Samples in Each Land-Cover Class Within DPaviaU-DPaviaC Dataset and the Number of Used Samples
TABLE III Number of Labeled Samples in Each Land-Cover Class Within RPaviaC-RPaviaU Dataset and the Number of Used Samples
TABLE IV Accuracies on EShanghai-EHangzhou Dataset
TABLE V Accuracies on DPaviaU-DPaviaC Dataset
TABLE VI Accuracies on RPaviaC-RPaviaU Dataset
TABLE VII Robustness Analysis on Outlier Samples. significance of bold entities are highest accuracy among compared methods.
TABLE VIII Computational Complexity
W. Sun, L. Tian, Y. Xu, D. Zhang and Q. Du, "Fast and robust self-representation method for hyperspectral band selection", IEEE J. Sel. Topics Appl. Earth Observ. Remote Sens. , vol. 10, no. 11, pp. 5087-5098, Nov. 2017.
Y. Qian, F. Yao and S. Jia, "Band selection for hyperspectral imagery using affinity propagation", IET Comput. Vis. , vol. 3, no. 9, pp. 213-222, 2009.
J. Fan and J. Lv, "A selective overview of variable selection in high dimensional feature space", Statistica Sinica , vol. 20, no. 1, pp. 101-148, 2010.
M. Pal and G. M. Foody, "Feature selection for classification of hyperspectral data by SVM", IEEE Trans. Geosci. Remote Sens. , vol. 48, no. 5, pp. 2297-2307, May 2010.
S. A. Medjahed and M. Ouali, "Band selection based on optimization approach for hyperspectral image classification", Egypt. J. Remote Sens. Space Sci. , vol. 21, no. 3, pp. 413-418, 2018.
A. Datta, S. Ghosh and A. Ghosh, "Combination of clustering and ranking techniques for unsupervised band selection of hyperspectral images", IEEE J. Sel. Topics Appl. Earth Observ. Remote Sens. , vol. 8, no. 6, pp. 2814-2823, Jun. 2015.
G. Chandrashekar and F. Sahin, "A survey on feature selection methods", Comput. Elect. Eng. , vol. 40, no. 1, pp. 16-28, 2014.
R. Kohavi and G. H. John, "Wrappers for feature subset selection", Artif. Intell. , vol. 97, no. 1, pp. 273-324, 1997.
C. Lai, W. Yeh and C. Chang, "Gene selection using information gain and improved simplified swarm optimization", Neurocomputing , vol. 218, pp. 331-338, 2016.
H. Marwa, B. Slim, H. Chih-Cheng and B. S. Lamjed, "A multi-objective hybrid filter-wrapper evolutionary approach for feature selection", ” Memetic Comput. , vol. 11, no. 2, pp. 193-208, 2018.
M. Ghosh, R. Guha, R. Sarkar and A. Abraham, "A wrapper-filter feature selection technique based on ant colony optimization", Neural Comput. Appl. , vol. 32, no. 12, pp. p. 7839–7857, 2020.
X. Cao, C. Wei, Y. Ge, J. Feng and J. A. Zhao, "Semi-supervised hyperspectral band selection based on dynamic classifier selection", IEEE J. Sel. Topics Appl. Earth Observ. Remote Sens. , vol. 12, no. 4, pp. 1289-1298, Apr. 2019.
M. Mafarja and S. Mirjalili, "Hybrid whale optimization algorithm with simulated annealing for feature selection", Neurocomputing , vol. 260, pp. 302-312, 2017.
M. Wang, C. Wu, L. Wang, D. Xiang and X. Huang, "A feature selection approach for hyperspectral image based on modified ant lion optimizer", Knowl.-Based Syst. , vol. 168, pp. 39-48, 2019.
X. Chen, G. Yuan, F. Nie and Z. Ming, "Semi-supervised feature selection via sparse rescaled linear square regression", IEEE Trans. Knowl. Data Eng. , vol. 32, no. 1, pp. 165-176, Jan. 2020.
H. Liu, M. Zhou and Q. Liu, "An embedded feature selection method for imbalanced data classification", IEEE/CAA J. Automatica Sinica , vol. 6, no. 3, pp. 703-715, May 2019.
W. Sun, J. Peng and G. Yang, "Correntropy-based sparse spectral clustering for hyperspectral band selection", IEEE Geosci. Remote Sens. Lett. , vol. 17, no. 3, pp. 484-488, Mar. 2020.
Q. Wang, F. Zhang and X. Li, "Optimal clustering framework for hyperspectral band selection", IEEE Trans. Geosci. Remote Sens. , vol. 56, no. 10, pp. 5910-5922, Oct. 2018.
Y. Yuan, J. Lin and W. Qi, "Dual-clustering-based hyperspectral band selection by contextual analysis", IEEE Trans. Geosci. Remote Sens. , vol. 54, no. 3, pp. 1431-1445, Mar. 2016.
M. Bevilacqua and Y. Berthoumieu, "Multiple-feature kernel-based probabilistic clustering for unsupervised band selection", IEEE Trans. Geosci. Remote Sens. , vol. 57, no. 9, pp. 6675-6689, Sep. 2019.
H. Zhai, H. Zhang, L. Zhang and P. Li, "Laplacian-regularized low-rank subspace clustering for hyperspectral image band selection", IEEE Trans. Geosci. Remote Sens. , vol. 57, no. 3, pp. 1723-1740, Mar. 2019.
W. Sun, L. Zhang, L. Zhang and Y. M. Lai, "A dissimilarity-weighted sparse self-representation method for band selection in hyperspectral imagery classification", IEEE J. Sel. Topics Appl. Earth Observ. Remote Sens. , vol. 9, no. 9, pp. 4374-4388, Sep. 2016.
S. Mallik, T. Bhadra and U. Maulik, "Identifying epigenetic biomarkers using maximal relevance and minimal redundancy based feature selection for multi-omics data", IEEE Trans. Nanobiosci. , vol. 16, no. 1, pp. 3-10, Jan. 2017.
J. Feng, L. Jiao, F. Liu, T. Sun and X. Zhang, "Mutual-information-based semi-supervised hyperspectral band selection with high discrimination high information and low redundancy", IEEE Trans. Geosci. Remote Sens. , vol. 53, no. 5, pp. 2956-2969, May 2015.
C. I. Chang, Y. M. Kuo, S. Chen, C. C. Liang, K. Y. Ma and P. F. Hu, "Self-mutual information-based band selection for hyperspectral image classification", IEEE Trans. Geosci. Remote Sens. .
B. Xu, X. Li, W. Hou, Y. Wang and Y. Wei, "A similarity-based ranking method for hyperspectral band selection", IEEE Trans. Geosci. Remote Sens. .
K. Sun, X. Geng, L. Ji and Y. Lu, "A new band selection method for hyperspectral image based on data quality", IEEE J. Sel. Topics Appl. Earth Observ. Remote Sens. , vol. 7, no. 6, pp. 2697-2703, Jun. 2014.
M. Ye, Y. Qian, J. Zhou and Y. Y. Tang, "Dictionary learning-based feature-level domain adaptation for cross-scene hyperspectral image classification", IEEE Trans. Geosci. Remote Sens. , vol. 55, no. 3, pp. 1544-1562, Mar. 2017.
J. Peng, W. Sun, L. Ma and Q. Du, "Discriminative transfer joint matching for domain adaptation in hyperspectral image classification", IEEE Geosci. Remote Sens. Lett. , vol. 16, no. 6, pp. 972-976, Jun. 2019.
W. Wang, L. Ma, M. Chen and Q. Du, "Joint correlation alignment-based graph neural network for domain adaptation of multitemporal hyperspectral remote sensing images", IEEE J. Sel. Topics Appl. Earth Observ. Remote Sens. , vol. 14, pp. 3170-3184, 2021.
H. Wei, L. Ma, Y. Liu and Q. Du, "Combining multiple classifiers for domain adaptation of remote sensing image classification", IEEE J. Sel. Topics Appl. Earth Observ. Remote Sens. , vol. 14, pp. 1832-1847, 2021.
C. Ni, W. Liu, Q. Gu, X. Chen and D. Chen, "A cluster based feature selection method for cross-project software defect prediction", J. Comput. Sci. Technol. , vol. 32, no. 6, pp. 1090-1107, 2017.
M. Ye, Y. Xu, H. Lu, K. Yan and Y. Qian, "Cross-scene feature selection for hyperspectral images based on cross-domain information gain", Proc. Int. Geosci. Remote Sens. Symp. , pp. 4764-4767, 2018.
R. J. Urbanowicz, M. Meeker, W. La Cava, R. S. Olson and J. H. Moore, "Relief-based feature selection: Introduction and review", J. Biomed. Inform. , vol. 85, pp. 189-203, 2018.
M. Ye, Y. Xu, C. Ji, H. Chen, H. Lu and Y. Qian, "Feature selection for cross-scene hyperspectral image classification using cross-domain ReliefF", Int. J. Wavelets Multiresolution Inf. Process. , vol. 17, no. 5, 2019.
Y. Sun, "Iterative RELIEF for feature weighting: Algorithms theories and applications", IEEE Trans. Pattern Anal. Mach. Intell. , vol. 29, no. 6, pp. 1035-1051, Jun. 2007.
L. Chen and D. Chen, "Alignment based feature selection for multi-label learning", Neural Process. Lett. , vol. 50, no. 3, pp. 2323-2344, 2019.
S. P. Patel and S. Upadhyay, "Euclidean distance based feature ranking and subset selection for bearing fault diagnosis", Expert Syst. with Appl. , vol. 154, no. 113400, 2020.
B. C. Kuo, H. H. Ho, C. H. Li and C. C. Hung, "A kernel-based feature selection method for SVM with RBF kernel for hyperspectral image classification", IEEE J. Sel. Topics Appl. Earth Observ. Remote Sens. , vol. 7, no. 1, pp. 317-326, Jan. 2014.
C. L. Huang and C. J. Wang, "A GA-based feature selection and parameters optimizationfor support vector machines", Expert Syst. Appl. , vol. 31, no. 2, pp. 231-240, 2006.
W. Zhang, X. Li and L. Zhao, "A fast hyperspectral feature selection method based on band correlation analysis", IEEE Geosci. Remote Sens. Lett. , vol. 15, no. 11, pp. 1750-1754, Nov. 2018.
IEEE Account
Change Username/Password
Update Address
Purchase Details
Payment Options
Order History
View Purchased Documents
Need Help?
US & Canada: +1 800 678 4333
Worldwide: +1 732 981 0060
Contact & Support
Most of the hyperspectral scenes include dozens or hundreds of spectral bands which may cause the Hughes phenomenon [1] . The high dimension and low sample size problem is a great challenge for hyperspectral images (HSIs) classification [2] – [4] . Some features are useful for pixel classification, while the other features have negative effects on the classification accuracy. Therefore, how to select a feature subset required by the HSI classification through a certain algorithm must be considered. An intuitive interpretation is that the feature selection problem is a combinatorial optimization problem which is used to compute the scores of different feature subsets. Feature selection obtains a most useful feature subset by eliminating irrelevant features. Irrelevant features will lead to decreased accuracies and overfitting problems [5] , [6] . According to the relationship with the learning model, existing feature selection algorithms can be generally categorized as filter methods, wrapper methods and embedded methods [7] , [8] . There are also some algorithms which combine the filter and wrapper methods [9] , [10] . Recently, Ghosh et al. [11] proposed a wrapper-filter combination of ant colony optimization, which introduces the subset evaluation using a filter method instead of using a wrapper method to reduce computational complexity. As for wrapper methods, the feature selection and classification are separated, and conducted iteratively. Typical examples are dynamic classifier [12] , hybrid whale optimization algorithm with simulated annealing [13] , and modified ant lion optimizer [14] . In the embedded methods, the feature selection and classification are unified into one model. Typical examples are sparse rescaled linear square regression [15] and weighted Gini index feature selection [16] . However, the computational cost of wrapper methods and embedded methods are high in application. In contrast to the other methods, using filter methods for feature selection has two advantages: efficiency and robustness. And many filter-based feature selection methods have been proposed, such as clustering-based, sparsity-based, and ranking-based methods. The clustering-based methods construct the feature subsets for the HSIs by grouping the similar features and separating dissimilar features within the clustering framework. The features around the cluster centroids are considered as the most representative features and selected to constitute the final feature subset. Typical examples are spectral clustering [17] , optimal clustering [18] , dual clustering [19] , and kernel-based probabilistic clustering [20] . However, the performance of clustering-based algorithm is usually sensitive to the number of clusters. On the other hand, the best feature subset in the cluster may not be the global best one. In recent years, sparsity-based methods have been applied to solve the feature selection problem, which make full use of sparse coefficients of all features to select features [17] . Some typical algorithms are as follows: Laplacian-regularized low-rank subspace clustering [21] and dissimilarity-weighted sparse self-representation [22] . However, the sparse coefficients are sensitive to the convergence of defined optimization program. The ranking-based methods evaluate the contribution of each feature to HSI classification, and select the top-ranked features. Examples of ranking-based methods are minimum redundancy maximum relevance [23] , mutual information [24] , [25] , and similarity-based ranking method [26] . In this article, we focus on the ranking-based methods, because every selected feature contains more useful information for classification.
It is conceivable that using abundant labeled samples can improve the performance of feature selection. However, there are some HSIs which lack labeled samples, because labeling samples is a costly, time-consuming, and labor-intensive task. On these HSIs with a small number of labeled samples, unsupervised learning and semi-supervised learning have played huge roles [12] , [18] , [27] . As the number of HSIs increases, it can be found that many HSIs are related. For example, cities always have the same land-cover classes, like land, trees, rivers, etc. Therefore, it is useful to utilize the strongly related scene with abundant labeled samples (named source scene) to improve the classification accuracy of the scene which lacks labeled samples (named target scene), that is, transfer learning. But when the labeled samples from the source scene are directly merged with a limited number of labeled samples from the target scene, the classification results are often poor. This is caused by spectral shift which is a common problem between different scenes [28] . The spectral features of the land-cover classes are affected in many ways, e.g., the difference on illumination, atmosphere, humidity, sensor, and even the angle of image acquisition. So how to reduce the impact of the spectral shift on HSI classification and promote the consistency of selected features between two related HSI scenes are huge challenges. Transfer learning is usually applied to classification and feature dimensionality reduction. Domain adaptation is a popular research direction in transfer learning, state-of-the-art classification algorithms include discriminative transfer joint matching [29] , joint correlation alignment-based graph neural network [30] , multiple domain adaptation fusion method, and the multiple base classifier fusion method [31] . And the typical examples of cross-domain feature selection are corss-domain feature selection using clustering (CDFSC) [32] and cross-domain information gain [33] .
The measure of distance between samples is a commonly used evaluation criterion in filter methods. Based on this evaluation criterion, the following conditions need to be considered. The feature distance between samples in the same class should be as small as possible, while the distance between samples in different classes should be larger. Following this principle, Kir
Шикарная латвийская модель Виола
Ебля зрелой с красивой жопой
Гэнг Бэнг у бассейна с азиатской шлюхой