Галерея 3025638
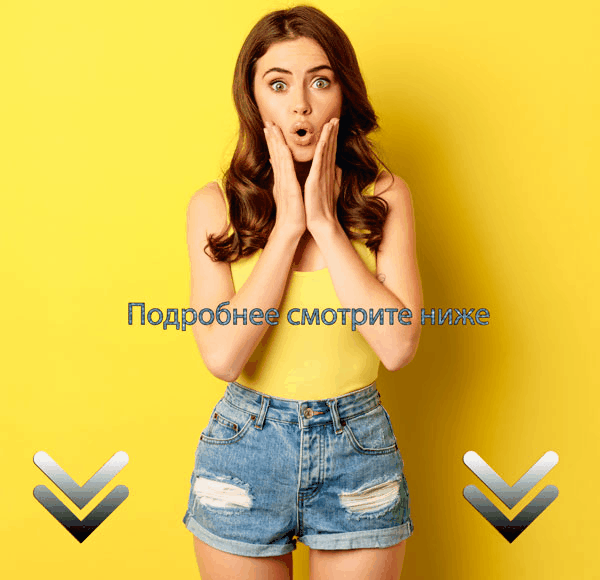
Галерея 3025638
Clipboard, Search History, and several other advanced features are temporarily unavailable.
Dashboard
Publications
Account settings
Log out
Advanced
Clipboard
Format
Abstract
PubMed
PMID
Format:
Summary (text)
PubMed
PMID
Abstract (text)
CSV
Subject:
1 selected item: 3025638 - PubMed
Format:
Summary
Summary (text)
Abstract
Abstract (text)
Create a new collection
Add to an existing collection
Name must be less than 100 characters
Unable to load your collection due to an error
Please try again
Unable to load your delegates due to an error
Please try again
Would you like email updates of new search results?
Saved Search Alert Radio Buttons
Yes
No
Frequency:
Monthly
Weekly
Daily
Which day?
The first Sunday
The first Monday
The first Tuesday
The first Wednesday
The first Thursday
The first Friday
The first Saturday
The first day
The first weekday
Which day?
Sunday
Monday
Tuesday
Wednesday
Thursday
Friday
Saturday
Report format:
Summary
Summary (text)
Abstract
Abstract (text)
PubMed
Send at most:
1 item
5 items
10 items
20 items
50 items
100 items
200 items
Send even when there aren't any new results
Number of items displayed:
5
10
15
20
50
100
Create a new collection Add to an existing collection
Name must be less than 100 characters
Unable to load your collection due to an error Please try again
Page navigation
Title & authors
Abstract
Similar articles
Cited by
References
Publication types
MeSH terms
Substances
Related information
LinkOut - more resources
W H Colledge et al.
Mol Cell Biol .
1986 Nov .
Format
Abstract
PubMed
PMID
Smith AE, Kalderon D, Roberts BL, Colledge WH, Edge M, Gillett P, Markham A, Paucha E, Richardson WD.
Smith AE, et al.
Proc R Soc Lond B Biol Sci. 1985 Oct 22;226(1242):43-58. doi: 10.1098/rspb.1985.0078.
Proc R Soc Lond B Biol Sci. 1985.
PMID: 2866523
Farber JM, Peden KW, Nathans D.
Farber JM, et al.
J Virol. 1987 Feb;61(2):436-45. doi: 10.1128/JVI.61.2.436-445.1987.
J Virol. 1987.
PMID: 3027373
Free PMC article.
Richardson WD, Roberts BL, Smith AE.
Richardson WD, et al.
Cell. 1986 Jan 17;44(1):77-85. doi: 10.1016/0092-8674(86)90486-1.
Cell. 1986.
PMID: 3000623
Manos MM, Gluzman Y.
Manos MM, et al.
Mol Cell Biol. 1984 Jun;4(6):1125-33. doi: 10.1128/mcb.4.6.1125-1133.1984.
Mol Cell Biol. 1984.
PMID: 6330530
Free PMC article.
Das GC, Niyogi SK, Salzman NP.
Das GC, et al.
Prog Nucleic Acid Res Mol Biol. 1985;32:217-36. doi: 10.1016/s0079-6603(08)60349-9.
Prog Nucleic Acid Res Mol Biol. 1985.
PMID: 3003799
Review.
No abstract available.
Nguyen NLT, Panté N.
Nguyen NLT, et al.
Cells. 2022 Sep 22;11(19):2957. doi: 10.3390/cells11192957.
Cells. 2022.
PMID: 36230922
Free PMC article.
Nakada R, Matsuura Y.
Nakada R, et al.
Protein Sci. 2017 Jun;26(6):1231-1235. doi: 10.1002/pro.3173. Epub 2017 Apr 17.
Protein Sci. 2017.
PMID: 28383161
Free PMC article.
Lokareddy RK, Hapsari RA, van Rheenen M, Pumroy RA, Bhardwaj A, Steen A, Veenhoff LM, Cingolani G.
Lokareddy RK, et al.
Structure. 2015 Jul 7;23(7):1305-1316. doi: 10.1016/j.str.2015.04.017. Epub 2015 Jun 4.
Structure. 2015.
PMID: 26051712
Free PMC article.
Lott K, Bhardwaj A, Sims PJ, Cingolani G.
Lott K, et al.
J Biol Chem. 2011 Aug 12;286(32):28160-9. doi: 10.1074/jbc.M111.228007. Epub 2011 Jun 20.
J Biol Chem. 2011.
PMID: 21690087
Free PMC article.
Giesecke A, Stewart M.
Giesecke A, et al.
J Biol Chem. 2010 Jun 4;285(23):17628-35. doi: 10.1074/jbc.M110.102343. Epub 2010 Mar 23.
J Biol Chem. 2010.
PMID: 20335181
Free PMC article.
Format:
AMA
APA
MLA
NLM
Send To
Clipboard
Email
Save
My Bibliography
Collections
Citation Manager
[x]
NLM
NIH
HHS
USA.gov
An official website of the United States government
The .gov means it’s official.
Federal government websites often end in .gov or .mil. Before
sharing sensitive information, make sure you’re on a federal
government site.
The site is secure.
The https:// ensures that you are connecting to the
official website and that any information you provide is encrypted
and transmitted securely.
Site-directed mutagenesis was used to change Lys-128 of the simian virus 40 large-T nuclear location signal to Met, Ile, Arg, Gln, Asn, Leu, or His. Except for the large-T antigen of the Arg mutation, which was present in cytoplasmic and nuclear compartments, the resultant proteins were unable to enter the nucleus. By contrast, mutations at other sites within the signal were generally less severe in their effect. In some cases (Lys-128 to Gln, Asn, and His), the apparently cytoplasmic variants were able to support limited plasmid DNA replication, suggesting that low levels of large-T antigen undetectable by immunofluorescence were present in the nucleus. Such mutants did not support viral DNA replication. We conclude that there is a strong requirement for a basic residue at position 128 in the large-T nuclear location signal, with Lys the preferred residue.
MeSH
PMC
Bookshelf
Disclaimer
The PubMed wordmark and PubMed logo are registered trademarks of the U.S. Department of Health and Human Services (HHS). Unauthorized use of these marks is strictly prohibited.
Help
Accessibility
Careers
All Books Conferences Courses Journals & Magazines Standards Authors Citations
Abstract: Deep learning-based models have greatly advanced the performance of speech enhancement (SE) systems. However, two problems remain unsolved, which are closely related to m... View more
Deep learning-based models have greatly advanced the performance of speech enhancement (SE) systems. However, two problems remain unsolved, which are closely related to model generalizability to noisy conditions: (1) mismatched noisy condition during testing, i.e., the performance is generally sub-optimal when models are tested with unseen noise types that are not involved in the training data; (2) local focus on specific noisy conditions, i.e., models trained using multiple types of noises cannot optimally remove a specific noise type even though the noise type has been involved in the training data. These problems are common in real applications. In this article, we propose a novel denoising autoencoder with a multi-branched encoder (termed DAEME) model to deal with these two problems. In the DAEME model, two stages are involved: training and testing. In the training stage, we build multiple component models to form a multi-branched encoder based on a decision tree (DSDT). The DSDT is built based on prior knowledge of speech and noisy conditions (the speaker, environment, and signal factors are considered in this paper), where each component of the multi-branched encoder performs a particular mapping from noisy to clean speech along the branch in the DSDT. Finally, a decoder is trained on top of the multi-branched encoder. In the testing stage, noisy speech is first processed by each component model. The multiple outputs from these models are then integrated into the decoder to determine the final enhanced speech. Experimental results show that DAEME is superior to several baseline models in terms of objective evaluation metrics, automatic speech recognition results, and quality in subjective human listening tests.
Date of Publication: 05 October 2020
TABLE I PESQ Scores of the DAEME-UAT {_6} System at Four Noise Types and Six SNRs. Avg. Denotes the Average Scores
TABLE II STOI Scores of the DAEME-UAT {_6} System at Four Noise Types and Six SNRs. Avg. Denotes the Average Scores
TABLE III PESQ Scores of the DAEME-USAT _{(SS)(12)} and DAEME-USAT _{(WD)(12)} Systems at Four Noise Types and Six SNRs. Avg. Denotes the Average Scores
TABLE IV STOI scores of the DAEME-USAT _{(SS)(12)} and DAEME-USAT _{(WD)(12)} Systems At Four Noise Types and Six SNRs. Avg. Denotes The Average Scores
TABLE V PESQ Scores of Seen Data of BLSTM and DAEME
TABLE VI STOI Scores of Seen Data of BLSTM and DAEME
S. V. Kuyk, W. B. Kleijn and R. C. Hendriks, "Intelligibility metric based on a simple model of speech communication", Proc. IWAENC , 2016.
J. Li, L. Deng, R. Haeb-Umbach and Y. Gong, "Robust automatic speech recognition: A bridge to practical applications", Elsevier Orlando FL USA: Academic , 2015.
Z.-Q. Wang and D. Wang, "A joint training framework for robust automatic speech recognition", IEEE/ACM Trans. Audio Speech Lang. Process. , vol. 24, no. 4, pp. 796-806, Apr. 2016.
C. Donahue, B. Li and R. Prabhavalkar, "Exploring speech enhancement with generative adversarial networks for robust speech recognition", Proc. ICASSP , pp. 5024-5028, 2018.
T. Ochiai, S. Watanabe, T. Hori and J. R. Hershey, "Multichannel end-to-end speech recognition", Proc. ICML , pp. 2632-2641, 2017.
D. Michelsanti and Z.-H. Tan, "Conditional generative adversarial networks for speech enhancement and noise-robust speaker verification", Proc. INTERSPEECH , 2017.
S. Shon, H. Tang and J. Glass, "Voiceid loss: Speech enhancement for speaker verification", Proc. INTERSPEECH , 2019.
D. Wang, "Deep learning reinvents the hearing aid", IEEE Spectr. , vol. 54, no. 3, pp. 32-37, Mar. 2017.
Y. Zhao, D. Wang, E. M. Johnson and E. W. Healy, "A deep learning based segregation algorithm to increase speech intelligibility for hearing-impaired listeners in reverberant-noisy conditions", The J. Acoust. Soc. Amer. , vol. 144, no. 3, pp. 1627-1637, 2018.
H. Puder, "Hearing aids: An overview of the state-of-the-art challenges and future trends of an interesting audio signal processing application", Proc. ISPA , pp. 1-6, 2009.
P. C. Loizou, "Signal-processing techniques for cochlear implants", IEEE Eng. Med. and Biol. magazine , vol. 18, no. 3, pp. 34-46, May-Jun. 1999.
P. C. Loizou, "Speech processing in vocoder-centric cochlear implants", Cochlear Brainstem Implants , vol. 64, pp. 109-143, 2006.
S.-S. Wang, Y. Tsao, H.-L. S. Wang, Y.-H. Lai and L. P.-H. Li, "A deep learning based noise reduction approach to improve speech intelligibility for cochlear implant recipients in the presence of competing speech noise", Proc. APSIPA , 2017.
S. Boll, "Suppression of acoustic noise in speech using spectral subtraction", IEEE Trans. Acoust. Speech Signal Process. , vol. 27, no. 2, pp. 113-120, Apr. 1979.
P. Scalart and J. Vieira Filho, "Speech enhancement based on a priori signal to noise estimation", Proc. ICASSP , 1996.
Y. Ephraim and D. Malah, "Speech enhancement using a minimum-mean square error short-time spectral amplitude estimator", IEEE Trans. Acoust. Speech and Signal Process. , vol. 32, no. 6, pp. 1109-1121, Dec. 1984.
Y. Ephraim and D. Malah, "Speech enhancement using a minimum mean-square error log-spectral amplitude estimator", IEEE Trans. Acoustics Speech Signal Process. , vol. 33, no. 2, pp. 443-445, Apr. 1985.
Y. Hu and P. C. Loizou, "A subspace approach for enhancing speech corrupted by colored noise", IEEE Trans. Speech Audio Process. , vol. 11, no. 4, 2002.
A. Rezayee and S. Gazor, "An adaptive klt approach for speech enhancement", IEEE Trans. Speech and Audio Process. , vol. 9, no. 2, pp. 87-95, Feb. 2001.
P. S. Huang, S. D. Chen, P. Smaragdis and M. A. Hasegawa-Johnson, "Singing-voice separation from monaural recordings using robust principal component analysis", Proc. ICASSP , vol. 11, 2012.
D. D. Lee and H. S. Seung, "Algorithms for non-negative matrix factorization", Proc. NIPS , 2001.
K. W Wilson, B. Raj, P. Smaragdis and A. Divakaran, "Speech denoising using nonnegative matrix factorization with priors", Proc. ICASSP , 2008.
N. Mohammadiha, P. Smaragdis and A. Leijon, "Supervised and unsupervised speech enhancement using nonnegative matrix factorization", IEEE Trans. Audio Speech Lang. Process. , vol. 21, no. 10, pp. 2140-2151, Oct. 2013.
J.-C. Wang, Y.-S. Lee, C.-H. Lin, S.-F. Wang, C.-H. Shih and C.-H. Wu, "Compressive sensing-based speech enhancement", IEEE/ACM Trans. Audio Speech Lang. Process. , vol. 24, no. 11, pp. 2122-2131, Nov. 2016.
J. Eggert and E. Korner, "Sparse coding and nmf", Proc. IJCNN , 2004.
Y.-H. Chin, J.-C. Wang, C.-L. Huang, K.-Y. Wang and C.-H. Wu, "Speaker identification using discriminative features and sparse representation", IEEE Trans. Inform. Forensics and Secur. , vol. 12, no. 8, pp. 1979-1987, Aug. 2017.
E. J. Candès, X. Li, Y. Ma and J. Wright, "Robust principal component analysis?", J. ACM , vol. 58, no. 3, pp. 11, 2011.
G. Hu and D. Wang, "Speech segregation based on pitch tracking and amplitude modulation", Proc. WASPAA , 2001.
G. Hu and D. Wang, "Complex ratio masking for monaural speech separation", IEEE/ACM Trans. Audio Speech Lang. Process. , vol. 14, no. 3, pp. 774-784, Mar. 2006.
S. Gonzalez and M. Brookes, "Mask-based enhancement for very low quality speech", Proc. ICASSP , 2014.
Y. Wang, A. Narayanan and D. Wang, "On training targets for supervised speech separation", IEEE/ACM Trans. Audio Speech Lang. Process. , vol. 22, no. 12, pp. 1849-1858, Dec. 2014.
H. Erdogan, J. R. Hershey, S. Watanabe and J. Le Roux, "Phase-sensitive and recognition-boosted speech separation using deep recurrent neural networks", Proc. ICASSP , 2015.
Y. Xu, J. Du, L.-R. Dai and C.-H. Lee, "An experimental study on speech enhancement based on deep neural networks", IEEE Signal Process. Lett. , vol. 21, no. 1, pp. 65-68, Jan. 2014.
D. Liu, P. Smaragdis and M. Kim, "Experiments on deep learning for speech denoising", Proc. INTERSPEECH , 2014.
X. Lu, Y. Tsao, S. Matsuda and C. Hori, "Speech enhancement based on deep denoising autoencoder", Proc. INTERSPEECH , 2013.
P. G. Shivakumar and P. G. Georgiou, "Perception optimized deep denoising autoencoders for speech enhancement", Proc. INTERSPEECH , pp. 3743-3747, 2016.
S.-W. Fu, Y. Tsao and X. Lu, "Snr-aware convolutional neural network modeling for speech enhancement", Proc. INTERSPEECH , 2016.
S.-W. Fu, T.-W. Wang, Y. Tsao, X. Lu and H. Kawai, "End-to-end waveform utterance enhancement for direct evaluation metrics optimization by fully convolutional neural networks", IEEE/ACM Trans. Audio Speech Langu. Process. , vol. 26, no. 9, pp. 1570-1584, Sep. 2018.
A. Pandey and D. Wang, "A new framework for CNN-based speech enhancement in the time domain", IEEE/ACM Trans. Audio Speech Lang. Process. , vol. 27, no. 7, pp. 1179-1188, Jul. 2019.
L. Sun, J. Du, L.-R. Dai and C.-H. Lee, "Multiple-target deep learning for LSTM-RNN based speech enhancement", Proc. HSCMA , 2017.
Z. Chen, S. Watanabe, H. Erdogan and J. R. Hershey, "Speech enhancement and recognition using multi-task learning of long short-term memory recurrent neural networks", Proc. INTERSPEECH , 2015.
Y. Bengio, J. Louradour, R. Collobert and J. Weston, "Curriculum learning", Proc. ICML , 2009.
M. P. Kumar, B. Packer and D. Koller, "Self-paced learning for latent variable models", Proc. NIPS , 2010.
H. Chang, E. Learned-Miller and A. McCallum, "Active bias: Training more accurate neural networks by emphasizing high variance samples", Proc. NIPS , 2017.
Y. Tsao and C.-H. Lee, "An ensemble speaker and speaking environment modeling approach to robust speech recognition", IEEE/ACM Trans. Audio Speech Lang. Process. , vol. 17, no. 5, pp. 1025-1037, Jul. 2009.
S. Paisitkriangkrai, C. Shen and A. van den Hengel, "Pedestrian detection with spatially pooled features and structured ensemble learning", IEEE Trans. Pattern Anal. Mach. Intell. , vol. 38, pp. 1243-1257, Jun. 2015.
S. Oh, M. S. Lee and B.-T. Zhang, "Ensemble learning with active example selection for imbalanced biomedical data classification", IEEE/ACM Trans. Comput.
Стриптиз Сарина Валентина
Стеф Брукс обладает очень соблазнительной внешностью
Анальный секс втроем со зрелыми блондинками