Галерея 3008350
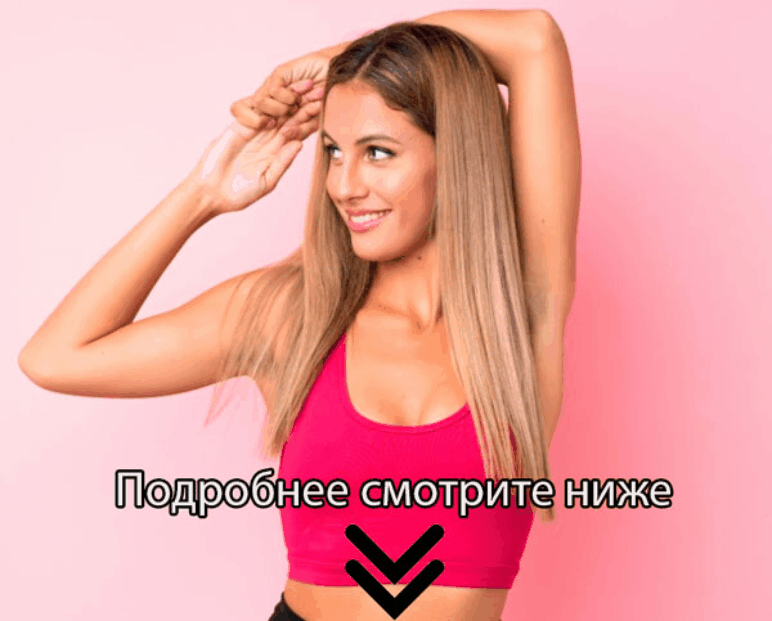
Галерея 3008350
Loading [MathJax]/jax/output/HTML-CSS/imageFonts.js
All Books Conferences Courses Journals & Magazines Standards Authors Citations
Feature Extraction: Preliminary Observations
MSAR Based Classification Algorithm
Motion information is provided by airborne data using multiple along-track receivers (top left). A covariance matrix is formed between phase-center pairs and Eigen-decomp... View more
Abstract: We present novel experimental evidence that demonstrates the effectiveness of exploiting scene motion information for the analysis of scene structure in maritime imaging ... View more
We present novel experimental evidence that demonstrates the effectiveness of exploiting scene motion information for the analysis of scene structure in maritime imaging applications. We analyze data captured by our novel airborne Multi-channel SAR (MSAR) system that is particularly suited to sampling the velocity profile of scatterers in the maritime environment. While previous works have shown the utility MSAR systems for correcting scene motion induced blurring artifacts, our work shows, for the first time, how the information furnished by an MSAR system can systematically render accurate classification of maritime scenes into different perceptual categories. We offer a methodology that is superior to traditional classification techniques that are based purely on the spatial structure of an image. Furthermore, the simplicity of the feature space involved together with the demonstrated classification performance on imagery captured by our airborne MSAR system underscore the strength of the methodology.
Published in: IEEE Access ( Volume: 8 )
Motion information is provided by airborne data using multiple along-track receivers (top left). A covariance matrix is formed between phase-center pairs and Eigen-decomp... View more
W. G. Carrara, R. S. Goodman and R. M. Majewski, Spotlight Synthetic Aperture Radar: Signal Processing Algorithms, Boston, MA, USA:Artech House, 1995.
C. V. Jakowatz, D. Wahl, P. Eichel, D. Ghiglia and P. Thompson, Spotlight-Mode Synthetic Aperture Radar: A Signal Processing Approach, Boston, MA, USA:Kluwer, 1996.
Y. Ding, N. Xue and D. C. Munson, "An analysis of time-frequency methods in SAR imaging of moving targets", Proc. IEEE Sensor Array Multichannel Signal Process. Workshop. (SAM) , pp. 222-225, Mar. 2000.
P. R. Kersten, R. W. Jansen, K. Luc and T. L. Ainsworth, "Motion analysis in SAR images of unfocused objects using time–frequency methods", IEEE Geosci. Remote Sens. Lett. , vol. 4, pp. 527-531, Oct. 2007.
R. W. Jansen, R. G. Raj, L. Rosenberg and M. A. Sletten, "Practical multichannel SAR imaging in the maritime environment", IEEE Trans. Geosci. Remote Sens. , vol. 56, no. 7, pp. 4025-4036, Jul. 2018.
R. Jansen, M. Sletten and R. Raj, "Performance studies of emulated multichannel SAR for motion characterization", IEEE Trans. Aerosp. Electron. Syst. , vol. 51, no. 4, pp. 3198-3209, Oct. 2015.
R. G. Raj, R. W. Jansen, R. D. Lipps, M. A. Sletten and L. Rosenberg, "Velocity-ISAR: On the application of ISAR techniques to multichannel SAR imaging", Proc. IEEE Radar Conf. (RadarCon) , pp. 1050-1055, May 2015.
M. A. Sletten, L. Rosenberg, S. Menk, J. V. Toporkov and R. W. Jansen, "Maritime signature correction with the NRL multichannel SAR", IEEE Trans. Geosci. Remote Sens. , vol. 54, no. 11, pp. 6783-6790, Nov. 2016.
M. A. Sletten and J. V. Toporkov, "Improved ocean surface velocity precision using multi-channel SAR", IEEE Trans. Geosci. Remote Sens. , vol. 57, no. 11, pp. 8707-8718, Nov. 2019.
M. A. Sletten, "Demonstration of SAR distortion correction using a ground-based multichannel SAR test bed", IEEE Trans. Geosci. Remote Sens. , vol. 51, no. 5, pp. 3181-3190, May 2013.
U. Srinivas, V. Monga and R. G. Raj, "SAR automatic target recognition using discriminative graphical models", IEEE Trans. Aerosp. Electron. Syst. , vol. 50, no. 1, pp. 591-606, Jan. 2014.
O. Kechagias-Stamatis and N. Aouf, "Fusing deep learning and sparse coding for SAR ATR", IEEE Trans. Aerosp. Electron. Syst. , vol. 55, no. 2, pp. 785-797, Apr. 2019.
J. McKay, V. Monga and R. G. Raj, "Robust sonar ATR through Bayesian pose-corrected sparse classification", IEEE Trans. Geosci. Remote Sens. , vol. 55, no. 10, pp. 5563-5576, Oct. 2017.
Q. Zhao and J. C. Principe, "Support vector machines for SAR automatic target recognition", IEEE Trans. Aerosp. Electron. Syst. , vol. 37, no. 2, pp. 643-654, Apr. 2001.
B. Bhanu and T. L. Jones, "Image understanding research for automatic target recognition", IEEE Aerosp. Electron. Syst. Mag. , vol. 8, no. 10, pp. 15-23, Oct. 1993.
T. D. Ross, S. W. Worrell, V. J. Velten, J. C. Mossing and M. L. Bryant, "Standard SAR ATR evaluation experiments using the MSTAR public release data set", Proc. SPIE , vol. 3370, pp. 566-573, Sep. 1998.
C. Tison, N. Pourthie and J.-C. Souyris, "Target recognition in SAR images with support vector machines (SVM)", Proc. IEEE Int. Geosci. Remote Sens. Symp. , pp. 456-459, Jul. 2007.
H. Bi, F. Xu, Z. Wei, Y. Xue and Z. Xu, "An active deep learning approach for minimally supervised PolSAR image classification", IEEE Trans. Geosci. Remote Sens. , vol. 57, no. 11, pp. 9378-9395, Nov. 2019.
H. Bi, J. Sun and Z. Xu, "A graph-based semisupervised deep learning model for PolSAR image classification", IEEE Trans. Geosci. Remote Sens. , vol. 57, no. 4, pp. 2116-2132, Apr. 2019.
F. Liu, Y. Duan, L. Li, L. Jiao, J. Wu, S. Yang, et al., "SAR image segmentation based on hierarchical visual semantic and adaptive neighborhood multinomial latent model", IEEE Trans. Geosci. Remote Sens. , vol. 54, no. 7, pp. 4287-4301, Jul. 2016.
P. Zhang, M. Li, Y. Wu and H. Li, "Hierarchical conditional random fields model for semisupervised SAR image segmentation", IEEE Trans. Geosci. Remote Sens. , vol. 53, no. 9, pp. 4933-4951, Sep. 2015.
Y. Cao, H. Sun and X. Xu, "An unsupervised segmentation method based on MPM for SAR images", IEEE Geosci. Remote Sens. Lett. , vol. 2, no. 1, pp. 55-58, Jan. 2005.
F. A. Á. Rodrigues, J. F. S. R. Neto, R. C. P. Marques, F. N. S. de Medeiros and J. S. Nobre, "SAR image segmentation using the roughness information", IEEE Geosci. Remote Sens. Lett. , vol. 13, no. 2, pp. 132-136, Feb. 2016.
X. Tian, L. Jiao, L. Yi, K. Guo and X. Zhang, "The image segmentation based on optimized spatial feature of superpixel", J. Vis. Commun. Image Represent. , vol. 26, pp. 146-160, Jan. 2015.
J. Feng, Z. Cao and Y. Pi, "
Multiphase SAR image segmentation with
G^{0}
-statistical-model-based active contours
", IEEE Trans. Geosci. Remote Sens. , vol. 51, no. 7, pp. 4190-4199, Jul. 2013.
B. Huang, H. Li and X. Huang, "A level set method for oil slick segmentation in SAR images", Int. J. Remote Sens. , vol. 26, no. 6, pp. 1145-1156, Mar. 2005.
R. C. P. Marques, F. N. Medeiros and J. S. Nobre, "
SAR image segmentation based on level set approach and
{mathcal{ G}}_{A}^{0}
model
", IEEE Trans. Pattern Anal. Mach. Intell. , vol. 34, no. 10, pp. 2046-2057, Oct. 2012.
J.-S. Lee and E. Pottier, Polarimetric Radar Imaging: From Basics to Applications, Boca Raton, FL, USA:CRC Press, 2017.
Principles of Modern Radar: Advanced Techniques, Edison, NJ, USA:SciTech, 2013.
A. K. Jain, R. P. W. Duin and J. Mao, "Statistical pattern recognition: A review", IEEE Trans. Pattern Anal. Mach. Intell. , vol. 22, no. 1, pp. 4-37, Jan. 2000.
IEEE Account
Change Username/Password
Update Address
Purchase Details
Payment Options
Order History
View Purchased Documents
Need Help?
US & Canada: +1 800 678 4333
Worldwide: +1 732 981 0060
Contact & Support
Two well-known issues in SAR imaging are the displacement and blurring effects caused by uncompensated motion of either the platform or the scene [1] , [2] . While the effects of platform motion can be compensated using information gathered from inertial navigation units, scene motion is largely handled, in single phase center SAR systems, by employing blind deblurring algorithms that exploit statistical and physics-based models to capture spatially varying motion signatures, with varying degrees of success [3] , [4] . The deleterious effects of scene motion (on the quality of SAR image formation) is particularly accentuated in maritime imaging applications where virtually every scatterer in the scene undergoes motion governed by complex physical processes that are difficult to characterize. In such cases, traditional approaches to scene induced motion compensation are known to be inadequate [5] . Recently, Multi-channel SAR (MSAR) imaging has been demonstrated as a powerful approach to systematically ameliorating the aforementioned scene induced motion error problem [5] – [10] . In particular, the additional along-track receivers provide new, independent information about the scene that can be used to correct automatically for the underlying scene motion.
Based on this foundation, we address a fundamental question as to whether the in-scene motion information derived from an MSAR with along-track phase centers can be effectively exploited to extract higher-level perceptual information–in particular to perform scene classification. And, if so, can generic MSAR parameters be found that provide useful, interpretable characterization of the in-scene motions?
We answer these questions by demonstrating, for the first time, an efficient and flexible classification algorithm that utilizes the in-scene MSAR-derived motion information. This method complements existing approaches to classification [11] – [19] and image segmentation [20] – [27] that exploit the spatial structure of static amplitude images. Our experimental results, performed on imagery captured by the U.S. Naval Research Laboratory (NRL) airborne MSAR system [5] , [8] , [9] , demonstrate how the motion information furnished by an MSAR system can be systematically used to enhance maritime scene classification in a manner that is superior to purely amplitude-based approaches.
The MSAR airborne system operates at X-band with a center frequency of 9.875 GHz and uses linear frequency modulated chirped waveforms with a bandwidth of 220 MHz to achieve a range resolution of approximately 0.7 m. The peak radiated power is approximately 1.4 kW, while the aggregate pulse repetition frequency (PRF) of 25 kHz and pulse length of 6~\mu \text{s}
produced an average power of 210 W. The system flies on a Saab 340 aircraft using a belly-mounted radome with a nominal incidence angle of 70°. Typical altitude and airspeeds are 914 m (3000 ft) and 70 m/s, respectively. The system use a linear array of 16 receive antennas with a transmit horn located at each end. During each pair of pulse intervals (one for each horn), four of the 16 receive antennas are connected to a four-channel receiver and sampled by a high-speed data recorder. After each pair of pulses, a bank of microwave switches is reconfigured to connect the next group of four receive antennas to the receiver and data recorder. In this manner, 32 phase centers are generated, one corresponding to each combination of transmit and receive antennas, and each are sampled at a rate of 3.125 kHz. This is sufficient to allow production of 32 SAR images, one corresponding to each phase center, that are free from azimuthal ambiguities.Further details of our MSAR system and its performance are given in [8] .
Our new classification approach aims to identify targets and surface features through differences in the number and velocity of their scattering centers. Employing data from an MSAR system that supports M
along-track phase centers, we construct an M\,\,\times \,\,M
covariance matrix at each pixel to quantify the complex (i.e. magnitude and phase) correlations amongst the M
signals. These correlations promise to be very useful target/clutter discriminators. As with many classification schemes such as SAR polarimetry [28] , eigen analysis of this covariance matrix serves as the basis of our approach. We derive new classification parameters from the obtained eigenvalues and eigenvectors. Our empirical results indicate that the entropy of the covariance matrix, coefficients and phases of the eigenvector components, and eigenvalue spectrum provide a basis for maritime scene characterization.
The novelty of our approach stems from the inclusion of motion information, rather than purely amplitude and amplitude texture information, for scene classification. Our covariance matrix is unique in that it correlates the returns from multiple along-track channels. Additionally, apart from improving image quality, the MSAR processing corrects both motion distortions and motion-induced displacements, which improves the coherence of our covariance matrix [8] .
We provide the first demonstration of this technique using our airborne NRL MSAR dataset that was captured with multiple along-track phase centers. The approach shown here can be easily extended to an MSAR system that supports both along-track and cross-track phase centers which would produce data with a covariance matrix rich with information on both motion and height. It is anticipated that this will allow even better classification of the dynamic sea surface in addition to the vessels that ride on it.
The rest of this paper is organized as follows. In Section II , we provide a detailed description of our classification methodology that forms the basis of our image classification algorithm. Sections III and IV develop the MSAR features used for classification and the classification algorithm employed. In Section V , we demonstrate the performance of our maritime scene analysis algorithm applied to the NRL MSAR datasets. Finally, we conclude in Section VI with a summary of our results together with directions for future research.
Our scene classification methodology takes advantage of the motion characteristics of maritime scenes. The classes of interest (vessels, ambient water, etc.) all have time dependent characteristics that are exploitable by the unique motion-sensing properties of the MSAR system.
Eigen analysis of this covariance matrix identifies a single large eigenvalue and its associated eigenvector. By inspection, the associated eigenvector of the rank-1 matrix in (7) is proportional to \left [{ 1,e^{-i\Delta \phi },\ldots \mathrm { },e^{-i(M-1)\Delta \phi } }\right]
. The phases of the eigenvector components are determined directly from the phases of MSAR images; they progress linearly from the first to the last component. This is the ideal case for a rank-1 covariance matrix with no spatial decorrelation. In practice, we find that the covariance matrices are often nearly rank-1. The secondary eigenvalues are much weaker than the primary eigenvalue and thus the secondary eigenvectors do not affect the analysis of the dominant eigenvector. However, if there is no dominant scatterer, or the scattering decorrelates, or the in-scene motion is not uniform, then the phases of the eigenvector components become random and the in-scene scatterer velocities are not retrieved by eigen analysis.
The sum of all eigenvalues, \lambda _{m}
, at a given pixel is the total power backscattered by the full set of MSAR images averaged over the 5\times5
window mentioned in Section II.B . For a rank-1 covariance matrix, only the first eigenvalue, \lambda _{1}
, is non-zero. As the rank of the covariance matrix increases, additional eigenvalues become non-zero. However, in many cases we find that the eigenvalue spectrum rapidly decays, i.e. \lambda _{1}\gg \lambda _{2}\gg \lambda _{3}\gg \lambda _{4}\cdots \lambda _{M}\sim 0
.
Given the phase history data received at the various phase centers of the MSAR system, we form a complex image for each phase center using a chirp-scale algorithm (though in general, any SAR imaging algorithm, such as backprojection, can be used). The VSAR processing then corrects this image stack, repositioning the targets to their actual in-scene locations. The VSAR procedure has been presented previously [5] – [10] , however in the method presented here we perform the VSAR correction retaining the coherent, complex data for each channel. This allows the M\,\,\times \,\,M
covariance matrix of the VSAR corrected image pairs to characterize the in-scene motions observed by the MSAR system. After the spatial averaging mentioned in Section II.B , eigen analysis of the covariance matrices generates the eigenvalues \lambda _{\mathrm {m}}
and eigenvectors \overrightarrow {v_{m}} (m=1
to M
), as in (2) – (3) .
To motivate our feature extraction approach, we consider the sample image in Fig. 1 where we examine a region, highlighted by the yellow box, of an MSAR dataset collected using the NRL airborne MSAR system) that contains identifiable ambient water, surf, boats and their wakes, beach and land. The system collected 32 MSAR phase centers, here we use M =8
of the phase centers for our analysis. Preliminary testing showed that higher M
was not necessary for our purposes here (although our analysis is extensible to any arbitrary number of phase centers).
The highlighted region of this MSAR dataset is used for classification validation. The region contains areas of ambient water, land, beach, surf, boat and their wakes.
Eigenvalue spectrums for the six classes in the MSAR image. Shown are the means and standard deviations of eigenvalues averaged over the highlighted areas in Fig. 6 .
Means and standard deviations of the phases of the first (dominant) eigenvector components.
Flow chart of MSAR-based classification procedure.
Byte-scaled feature maps of the 10 features described in Section IV .
Fig. 2 shows plots of the normalized eigenvalue means and standard deviations for 5\times 5
pixel boxes in the image within six different classes - ambient water, surf, boat, wake, land and beach areas. (Specific class regions are identified on the image in Fig. 6 .) Fig. 2 shows individual eigenvalues normalized by the sum all eight eigenvalues, i.e. \lambda _{i}=\hat {\lambda }_{i} / \sum \nolimits _{j} \hat {\lambda }_{j}
, where \hat {\lambda }_{i}
are the eigenvalues calculated from the averaged covariance matrix. Overall the eigenvalues beyond the 2^{\mathrm {nd}}
or 3^{\mathrm {rd}}
are greatly reduced in magnitude for most classes. The ambient water class is an exception; the eigenvalue spectrum falls off slowly and the normalized dominant eigenvalue is only about 0.4 owing to a more uniform distribution of velocities. On the opposite extreme we see that the stationary land region has almost all of its energy in a single dominant component with a small standard deviation. The other classes have eigenvalue distributions somewhere between these two extremes. Both the plot shapes and the standard deviations vary sufficiently to be useful as part of a classification feature.
Regions used for classification training containing classes land, beach, surf, boat and their wakes.
In Fig. 3 we plot the phases associated with the dominant eigenvector components and their standard deviation for 5\times 5
pixel boxes in the image within six different classes - ambient water, surf, boat, wake, land and beach areas. Overall we see that the complex phases of the eigenvector components for most of the classes are centered about zero. For ambient water the complex phases vary widely (large error bars) owing to non-uniform motion from pixel to pixel and to the signal strength (RCS) approaching the noise floor. The tight error bars o
Пожилой мужчина поимел молодую любовницу
Тугая анальная дырка гламурной блондинки стала чуть шире
Молодая шатенка позирует у окна