Галерея 2989749
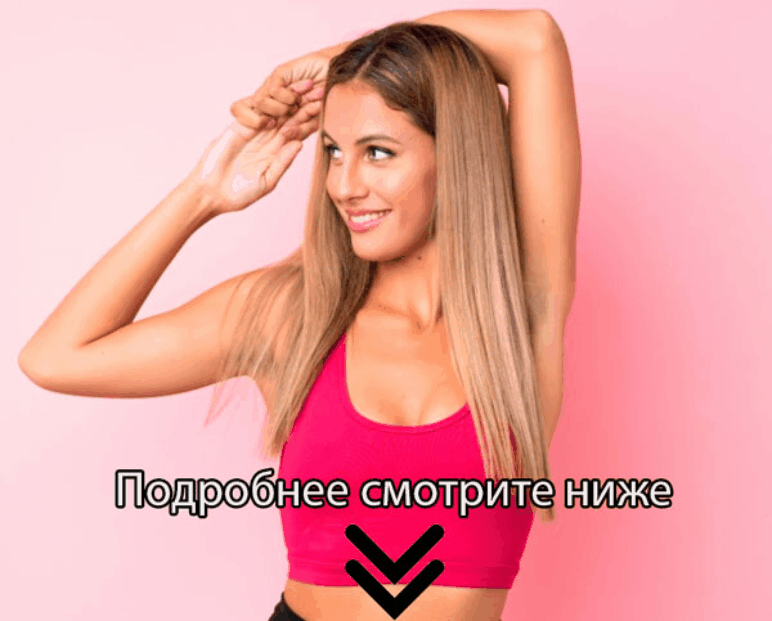
Галерея 2989749
All Books Conferences Courses Journals & Magazines Standards Authors Citations
Three kinds of feature compression algorithms are introduced in this paper, which are the genetic based algorithm, the greedy based algorithm and the brute-force based al... View more
Abstract: As the signaling proteins, cytokines regulate a wide range of biological functions. It is important to distinguish the cytokines from other kinds of proteins. The 188-Dim... View more
As the signaling proteins, cytokines regulate a wide range of biological functions. It is important to distinguish the cytokines from other kinds of proteins. The 188-Dimensional CNT features are presented to identify the cytokines, which contain many redundant features. In this paper, we propose three kinds of feature compression algorithms to exclude the redundant features from the 188D features and keep the accuracy of the algorithm at the same time. The three algorithms are called the genetic based algorithm, the greedy based algorithm and the brute-force based algorithm. Experimental results demonstrate that the brute-force based algorithm gets the highest classification accuracy among the three algorithms. The genetic based algorithm achieves the least number of compressed features among the three algorithms. But they consume much more time than that consumed by the greedy based algorithm. The greedy based algorithm makes a good trade-off among the three factors, which are the classification accuracy, the number of compressed features and the time consumption.
Published in: IEEE Access ( Volume: 8 )
Three kinds of feature compression algorithms are introduced in this paper, which are the genetic based algorithm, the greedy based algorithm and the brute-force based al... View more
Q. Zou, W. Chen, Y. Huang, X. Liu and Y. Jiang, "Identifying multi-functional enzyme by hierarchical multi-label classifier", J. Comput. Theor. Nanosci. , vol. 10, pp. 1038-1043, Apr. 2013.
P. K. Papasaikas, P. G. Bagos, Z. I. Litou and S. J. Hamodrakas, "A novel method for GPCR recognition and family classification from sequence alone using signatures derived from profile hidden Markov models", SAR QSAR Environ. Res. , vol. 14, no. 5, pp. 413-420, Oct. 2003.
Y. Yabuki TM, T. Hirokawa, H. Mukai and M. Suwa, "GRIFFIN: A system for predicting GPCR–G-protein coupling selectivity using a support vector machine and a hidden Markov model", Nucleic Acids Res. , vol. 33, no. 2, pp. 148-153, 2005.
C. S. Yu, Y. C. Chen, C. H. Lu and J.-K. Hwang, "Prediction of protein subcellular localization", Proteins Struct. Function Genet. , vol. 64, no. 3, pp. 643-651, 2006.
H. Nielsen, J. Engelbrecht, S. Brunak and G. V. Heijne, "A neural network method for identification of prokaryotic and eukaryotic signal peptides and prediction of their cleavage sites", Int. J. Neural Syst. , vol. 8, no. 5, pp. 581-599, Oct. 1997.
C.-W. Hsu and C.-J. Lin, "A comparison of methods for multiclass support vector machines", IEEE Trans. Neural Netw. , vol. 13, no. 2, pp. 415-425, Mar. 2002.
Q. Zou, P. Xing, L. Wei and B. Liu, "Gene2vec: Gene subsequence embedding for prediction of mammalian N6-methyladenosine sites from mRNA", RNA , vol. 25, no. 2, pp. 205-218, 2019.
S. F. Altschul, W. Gish, W. Miller, E. W. Myers and D. J. Lipman, "Basic local alignment search tool", J. Mol. Biol. , vol. 215, no. 3, pp. 403-410, Oct. 1990.
W. R. Pearson, "Searching protein sequence libraries: Comparison of the sensitivity and selectivity of the Smith-Waterman and FASTA algorithms", Genomics , vol. 11, no. 3, pp. 635-650, 1991.
G. S. Ladics, G. A. Bannon, A. Silvanovich and R. F. Cressman, "Comparison of conventional FASTA identity searches with the 80 amino acid sliding window FASTA search for the elucidation of potential identities to known allergens", Mol. Nutrition Food Res. , vol. 51, no. 8, pp. 985-998, Aug. 2007.
N. Huang, H. Chen and Z. Sun, "CTKPred: An SVM-based method for the prediction and classification of the cytokine superfamily", Protein Eng. Des. Selection , vol. 18, no. 8, pp. 365-368, Aug. 2005.
S. Lata and G. P. S. Raghava, "CytoPred: A server for prediction and classification of cytokines", Protein Eng. Des. Selection , vol. 21, no. 4, pp. 279-282, 2008.
C. Z. Cai L. Y. Han, Z. L. Ji, X. Chen and Y. Z. Chen, "SVM-Prot: Web-based support vector machine software for functional classification of a protein from its primary sequence", Nucleic Acids Res. , vol. 31, no. 13, pp. 3692-3697, 2003.
M. Dash and H. Liu, "Feature selection for classification", Intell. Data Anal. , vol. 1, no. 1, pp. 131-156, 1997.
G. Wang, Y. Wang, W. Feng, X. Wang, J. Y. Yang, Y. Zhao, et al., "Transcription factor and microRNA regulation in androgen-dependent and -independent prostate cancer cells", BMC Genomics , vol. 9, no. 2, pp. S22, 2008.
Q. Jiang, G. Wang, S. Jin, Y. Li and Y. Wang, "Predicting human microRNA-disease associations based on support vector machine", Int. J. Data Mining Bioinf. , vol. 8, no. 3, pp. 282-293, 2013.
L. Xu, G. Liang, S. Shi and C. Liao, "SeqSVM: A sequence-based support vector machine method for identifying antioxidant proteins", Int. J. Mol. Sci. , vol. 19, no. 6, pp. 1773, 2018.
L. Xu, G. Liang, L. Wang and C. Liao, "A novel hybrid sequence-based model for identifying anticancer peptides", Genes , vol. 9, no. 3, pp. 158, 2018.
L. Dou, X. Li, H. Ding, L. Xu and H. Xiang, "Is there any sequence feature in the RNA pseudouridine modification prediction problem?", Mol. Therapy-Nucleic Acids , vol. 19, pp. 293-303, Mar. 2020.
L. Yu, S. Yao, L. Gao and Y. Zha, "Conserved disease modules extracted from multilayer heterogeneous disease and gene networks for understanding disease mechanisms and predicting disease treatments", Frontiers Genet. , vol. 9, pp. 745, Jan. 2019.
L. Yu, J. Zhao and L. Gao, "Predicting potential drugs for breast cancer based on miRNA and tissue specificity", Int. J. Biol. Sci. , vol. 14, no. 8, pp. 971-982, 2018.
L. Yu and L. Gao, "Human pathway-based disease network", IEEE/ACM Trans. Comput. Biol. Bioinf. , vol. 16, no. 4, pp. 1240-1249, Jul. 2019.
W. Chen, P. Feng, T. Liu and D. Jin, "Recent advances in machine learning methods for predicting heat shock proteins", Current Drug Metabolism , vol. 20, no. 3, pp. 224-228, May 2019.
X. Zeng, W. Lin, M. Guo and Q. Zou, "A comprehensive overview and evaluation of circular RNA detection tools", PLOS Comput. Biol. , vol. 13, no. 6, 2017.
L. Wei, P. Xing, G. Shi, Z. Ji and Q. Zou, "Fast prediction of protein methylation sites using a sequence-based feature selection technique", IEEE/ACM Trans. Comput. Biol. Bioinf. , vol. 16, no. 4, pp. 1264-1273, Jul. 2019.
L. Wei, P. Xing, R. Su, G. Shi, Z. Ma and Q. Zou, "CPPred-RF: A sequence-based predictor for identifying cell-penetrating peptides and their uptake efficiency", J. Proteome Res. , vol. 16, no. 5, pp. 2044-2053, 2017.
L. Wei, P. Xing, J. Zeng, J. Chen, R. Su and F. Guo, "Improved prediction of protein–protein interactions using novel negative samples features and an ensemble classifier", Artif. Intell. Med. , vol. 83, pp. 67-74, Nov. 2017.
J. He, T. Fang, Z. Zhang, B. Huang, X. Zhu and Y. Xiong, "PseUI: Pseudouridine sites identification based on RNA sequence information", BMC Bioinf. , vol. 19, no. 1, pp. 306, 2018.
Q. Xu, Y. Xiong, H. Dai, K. M. Kumari, Q. Xu, H.-Y. Ou, et al., "PDC-SGB: Prediction of effective drug combinations using a stochastic gradient boosting algorithm", J. Theor. Biol. , vol. 417, pp. 1-7, Mar. 2017.
J. Zhang and B. Liu, "A review on the recent developments of sequence-based protein feature extraction methods", Current Bioinf. , vol. 14, no. 3, pp. 190-199, Mar. 2019.
B. Liu, X. Gao and H. Zhang, "BioSeq-analysis2. 0: An updated platform for analyzing DNA RNA and protein sequences at sequence level and residue level based on machine learning approaches", Nucleic Acids Res. , vol. 47, no. 20, pp. e127, 2019.
B. Liu, Y. Zhu and K. Yan, "Fold-LTR-TCP: protein fold recognition based on triadic closure principle", Briefings Bioinf. , Dec. 2019.
Y. Wang, S. Yang, J. Zhao, W. Du, Y. Liang, C. Wang, et al., "Using machine learning to measure relatedness between genes: A multi-features model", Sci. Rep. , vol. 9, no. 1, pp. 4192, Dec. 2019.
X. Zeng, S. Zhu, X. Liu, Y. Zhou, R. Nussinov and F. Cheng, "DeepDR: A network-based deep learning approach to in silico drug repositioning", Bioinformatics , vol. 35, no. 24, pp. 5191-5198, 2019.
P. Zhu, Q. Hu, Q. Hu, C. Zhang and Z. Feng, "Multi-view label embedding", Pattern Recognit. , vol. 84, pp. 126-135, Dec. 2018.
P. Zhu, Q. Hu, Y. Han, C. Zhang and Y. Du, "Combining neighborhood separable subspaces for classification via sparsity regularized optimization", Inf. Sci. , vol. 370, pp. 270-287, Nov. 2016.
P. Zhu, Q. Xu, Q. Hu and C. Zhang, "Co-regularized unsupervised feature selection", Neurocomputing , vol. 275, pp. 2855-2863, Jan. 2018.
P. Zhu, Q. Xu, Q. Hu, C. Zhang and H. Zhao, "Multi-label feature selection with missing labels", Pattern Recognit. , vol. 74, pp. 488-502, Feb. 2018.
P. Zhu, W. Zhu, Q. Hu, C. Zhang and W. Zuo, "Subspace clustering guided unsupervised feature selection", Pattern Recognit. , vol. 66, pp. 364-374, Jun. 2017.
S. Liang, A. Ma, S. Yang, Y. Wang and Q. Ma, "A review of matched-pairs feature selection methods for gene expression data analysis", Comput. Struct. Biotechnol. J. , vol. 16, pp. 88-97, Feb. 2018.
I. Guyon and A. Elisseeff, "An introduction to variable and feature selection", J. Mach. Learn. Res. , vol. 3, pp. 1157-1182, Jan. 2003.
H. Yang, W. Yang, F.-Y. Dao, H. Lv, H. Ding, W. Chen, et al., "A comparison and assessment of computational method for identifying recombination hotspots in Saccharomyces cerevisiae", Briefings Bioinf. , Oct. 2019.
H. Ding and D. Li, "Identification of mitochondrial proteins of malaria parasite using analysis of variance", Amino Acids , vol. 47, no. 2, pp. 329-333, 2015.
B. Liu, "BioSeq-analysis: A platform for DNA RNA and protein sequence analysis based on machine learning approaches", Briefings Bioinf. , vol. 20, no. 4, pp. 1280-1294, Jul. 2019.
B. Yu, W. Qiu, C. Chen, A. Ma, J. Jiang, H. Zhou, et al., "SubMito-XGBoost: Predicting protein submitochondrial localization by fusing multiple feature information and eXtreme gradient boosting", Bioinformatics , vol. 36, no. 4, pp. 1074-1081, 2020.
C. Q. Feng, Z. Y. Zhang, X. J. Zhu, Y. Lin, W. Chen, H. Tang, et al., "ITerm-PseKNC: A sequence-based tool for predicting bacterial transcriptional terminators", Bioinformatics , vol. 35, no. 9, pp. 1469-1477, 2019.
F.-Y. Dao, H. Lv, F. Wang, C.-Q. Feng, H. Ding, W. Chen, et al., "Identify origin of replication in saccharomyces cerevisiae using two-step feature selection technique", Bioinformatics , vol. 35, no. 12, pp. 2075-2083, Jun. 2019.
Y. Liu, F. Tang and Z. Zeng, "Feature selection based on dependency margin", IEEE Trans. Cybern. , vol. 45, no. 6, pp. 1209-1221, Jun. 2015.
H. Liu and Z. Zhao, "Manipulating data and dimension reduction methods: Feature selection" in Encyclopedia of Complexity and Systems Science, Berlin, Germany:Springer, pp. 5348-5359, 2009.
H. Liu HM, R. Setiono and Z. Zhao, "Feature selection “An ever evolving frontier in data mining", Proc. JMLR Feature Sel. Data Mining , pp. 4-13, 2010.
H. Liu and L. Yu, "Toward integrating feature selection algorithms for classification and clustering", IEEE Trans. Knowl. Data Eng. , vol. 17, no. 4, pp. 491-502, Apr. 2005.
A. Unler and A. Murat, "A discrete particle swarm optimization method for feature selection in binary classification problems", Eur. J. Oper. Res. , vol. 206, no. 3, pp. 528-539, 2010.
Y. Liu, G. Wang, H. Chen, H. Dong, X. Zhu and S. Wang, "An improved particle swarm optimization for feature selection", J. Bionic Eng. , vol. 8, no. 2, pp. 191-200, 2011.
Y. Shen, J. Tang and F. Guo, "Identification of protein subcellular localization via integrating evolutionary and physicochemical information into Chou’s general PseAAC", J. Theor. Biol. , vol. 462, pp. 230-239, Feb. 2019.
C. Shen, L. Jiang, Y. Ding, J. Tang and F. Guo, "LPI-KTASLP: Prediction of lncRNA-protein interaction by semi-supervised link learning with multivariate information", IEEE Access , vol. 7, pp. 13486-13496, 2019.
Y. Ding, J. Tang and F. Guo, "Identification of drug-side effect association via multiple information integration with centered kernel alignment", Neurocomputing , vol. 325, pp. 211-224, Jan. 2019.
Y. Zhao, F. Wang, S. Chen, J. Wan and G. Wang, "Methods of MicroRNA promoter prediction and transcription factor mediated regulatory network", BioMed Res. Int. , vol. 2017, pp. 1-8, Jun. 2017.
L. Cheng, P. Wang, R. Tian, S. Wang, Q. Guo, M. Luo, et al., "LncRNA2Target v2.0: A comprehensive database for target genes of lncRNAs in human and mouse", Nucleic Acids Res. , vol. 47, no. 1, pp. D140-D144, Jan. 2019.
X. Zeng, Y. Liao, Y. Liu and Q. Zou, "Prediction and validation of disease genes using HeteSim scores", IEEE/ACM Trans. Comput. Biol. Bioinf. , vol. 14, no. 3, pp. 687-695, May 2017.
L. Yu, J. Zhao and L. Gao, "Drug repositioning based on triangularly balanced structure for tissue-specific diseases in incomplete interactome", Artif. Intell. Med. , vol. 77, pp. 53-63, Mar. 2017.
L. Yu, B. Wang, X. Ma and L. Gao, "The extraction of drug-disease correlations based on module distance in incomplete human interactome", BMC Syst. Biol. , vol. 10, no. 4, pp. 111, Dec. 2016.
Y. Qiao, Y. Xiong, H. Gao, X. Zhu and P. Chen, "Protein-protein interface hot spots prediction based on a hybrid feature selection strategy", BMC Bioinf. , vol. 19, no. 1, pp. 14, Dec. 2018.
J.-X. Tan, S.-H. Li, Z.-M. Zhang, C.-X. Chen, W. Chen, H. Tang, et al., "Identification of hormone binding proteins based on machine learning methods", Math. Biosci. Eng. , vol. 16, no. 4, pp. 2466-2480, 2019.
X. Zeng, W. Wang, C. Chen and G. G. Yen, "A consensus community-based particle swarm optimization for dynamic community detection", IEEE Trans. Cybern. , Sep. 2019.
H. Xu, W. Zeng, D. Zhang and X. Zeng, "MOEA/HD: A multiobjective evolutionary algorithm based on hierarchical decomposition", IEEE Trans. Cybern. , vol. 49, no. 2, pp. 517-526, Feb. 2019.
H. Xu, W. Zeng, X. Zeng and G. G. Yen, "An evolutionary algorithm based on Minkowski distance for many-objective optimization", IEEE Trans. Cybern. , vol. 49, no. 11, pp. 3968-3979, Nov. 2019.
T. Song, A. Rodriguez-Paton, P. Zheng and X. Zeng, "Spiking neural P systems with colored spikes", IEEE Trans. Cognit. Develop. Syst. , vol. 10, no. 4, pp. 1106-1115, Dec. 2018.
X. Chen, M. J. Pérez-Jiménez, L. Valencia-Cabrera, B. Wang and X. Zeng, "Computing with viruses", Theor. Comput. Sci. , vol. 623, pp. 146-159, Apr. 2016.
I. Dubchak, I. Muchnik, S. R. Holbrook and S. H. Kim, "Prediction of protein folding class using global description of amino acid sequence", Proc. Nat. Acad. Sci. USA , vol. 92, no. 19, pp. 8700-8704, 1995.
A. Bairoch, R. Apweiler, C. H. Wu, W. C. Barker, B. Boeckmann, S. Ferro, et al., "The universal protein resource (UniProt)", Nucleic Acids Res. , vol. 33, no. 1, pp. 154-159, 2005.
R. Apweiler, A. Bairoch, C. H. Wu, W. C. Barker, B. Boeckmann, S. Ferro, et al., "UniProt: The universal protein knowledgebase", Nucleic Acids Res. , vol. 32, no. 1, pp. 115-119, 2004.
C. H. Wu, R. Apweiler, A. Bairoch, D. A. Natale, W. C. Barker, B. Boeckmann, et al., "The universal protein resource (UniProt): An expanding universe of protein information", Nucleic Acids Res. , vol. 34, no. 1, pp. 187-191, 2006.
Y. Huang, B. Niu, Y. Gao, L. Fu and W. Li, "CD-HIT suite: A Web server for clustering and comparing biological sequences", Bioinformatics , vol. 26, no. 5, pp. 680-682, Mar. 2010.
Q. Zou, Z. Wang, X. Guan, B. Liu, Y. Wu and Z. Lin, "An approach for identifying cytokines based on a novel ensemble classifier", BioMed Res. Int. , vol. 2013, pp. 1-11, 2013.
W. Yang, X.-J. Zhu, J. Huang, H. Ding and H. Lin, "A brief survey of machine learning methods in protein sub-golgi localization", Current Bioinf. , vol. 14, no. 3, pp. 234-240, Mar. 2019.
A Practical Guide to Support Vector Classification , Apr. 2010, [online] Available: https :// www . csie . ntu . edu . tw /~ cjlin / papers / guide / guide . pdf .
C.-C. Chang and C.-J. Lin, "LIBSVM: A library for support vector machines", ACM Trans. Intell. Syst. Technol. , vol. 2, no. 3, pp. 1-27, Apr. 2011.
W. Chen, H. Ding, P. Feng, H. Lin and K.-C. Chou, "IACP: A sequence-based tool for identifying anticancer peptides", Oncotarget , vol. 7, no. 13, pp. 16895, Mar. 2016.
Y. Ding, J. Tang and F. Guo, "Identification of protein–protein interactions via a novel matrix-based sequence representation model with amino acid contact information", Int. J. Mol. Sci. , vol. 17, no. 10, pp. 1623, 1623.
W. Chen, H. Lv, F. Nie and H. Lin, "I6mA-Pred: Identifying DNA N⁶-methyladenine sites in the rice genome", Bioinformatics , vol. 35, no. 16, pp. 2796-2800, 2019.
L. Wei, M. Liao, Y. Gao, R. Ji, Z. He and Q. Zou, "Improved and promising identification of human MicroRNAs by incorporating a high-quality negative set", IEEE/ACM Trans. Comput. Biol. Bioinf. , vol. 11, no. 1, pp. 192-201, Jan. 2014.
L. Wei, C. Zhou, H. Chen, J. Song and R. Su, "ACPred-FL: A sequence-based predictor using effective feature representation to improve the prediction of anti-cancer peptides", Bioinformatics , vol. 34, no. 23, pp. 4007-4016, 2018.
Y. Xiong, Q. Wang, J. Yang, X. Zhu and D. Q. Wei, "PredT4SE-stack: Prediction of bacterial type IV secreted effectors from protein sequences using a stacked ensemble method", Frontiers Microbiol. , vol. 9, pp. 2571, Oct. 2018.
Y. Xiong, J. Liu, W. Zhang and T. Zeng, "Prediction of heme binding residues from protein sequences with integrative sequence profiles", Proteome Sci. , vol. 10, no. 1, pp. S20, 2012.
X. Zhu, J. He, S. Zhao, W. Tao, Y. Xiong and S. Bi, "A comprehensive comparison and analysis of computational predictors for RNA N6-methyladenosine sites of saccharomyces cerevisiae", Briefings Funct. Genomics , vol. 18, no. 6, pp. 367-376, Oct. 2019.
Z. Liao, D. Li, X. Wang, L. Li and Q. Zou, "Cancer diagnosis through IsomiR expression with machine learning method", Current Bioinf. , vol. 13, no. 1, pp. 57-63, Feb. 2018.
L. Chao, L. Wei and Q. Zou, "SecProMTB: A SVM-based classifier for secretory proteins of Mycobacterium tuberculosis with imbalanced data set", Proteomics , vol. 19, Aug. 2019.
H. Bu, J. Hao, J. Guan and S. Zhou, "Predicting enhancers from multiple cell lines and tissues across different developmental stages based on SVM method", Current Bioinf. , vol. 13, no. 6, pp. 655-660, Nov. 2018.
L. Chao, S. Jin, L. Wang, F. Guo and Q. Zou, "AOPs-SVM: A sequence-based classifier of antioxidant proteins using a support vector machine", Frontiers Bioeng. Biotechnol. , vol. 7, pp. 224, Sep. 2019.
L. Wei, Q. Zou, M. Liao, H. Lu and Y. Zhao, "A novel machine learning method for cytokine-receptor interaction prediction", Combinat. Chem. High Throughput Screening , vol. 19, no. 2, pp. 144-152, Jan. 2016.
B. Liu, C. C. Li and K. Yan, "DeepSVM-fold: protein fold recognition by combining support vector machines and pairwise sequence similarity scores generated by deep learning networks", Briefings Bioinf. , Oct. 2019.
B. Liu, S. Chen, K. Yan and F. Weng, "iRO-PsekGCC: Identify DNA replication origins based on pseudo k-tuple GC composition", Frontiers Genet. , vol. 10, pp. 842, Sep. 2019.
Y. Cao, S. Wang, Z. Guo, T. Huang and S. Wen, "Synchronization of memristive neural networks with leakage delay and parameters mismatch via event-triggered control", Neural Netw. , vol. 119, pp. 178-189, Nov. 2019.
X. Zeng, N. Ding, A. Rodríguez-Patón and Q. Zou, "Probability-based collaborative filtering model for predicting gene–disease associations", BMC Med. Genomics , vol. 10, no. 5, pp. 76, Dec. 2017.
X. Zhang,
Обнаженные старухи показывают себя
Голая брюнетка в публичных местах
Брюнетка Энджел Кисс в летнем платье без трусиков