Галерея 2923425
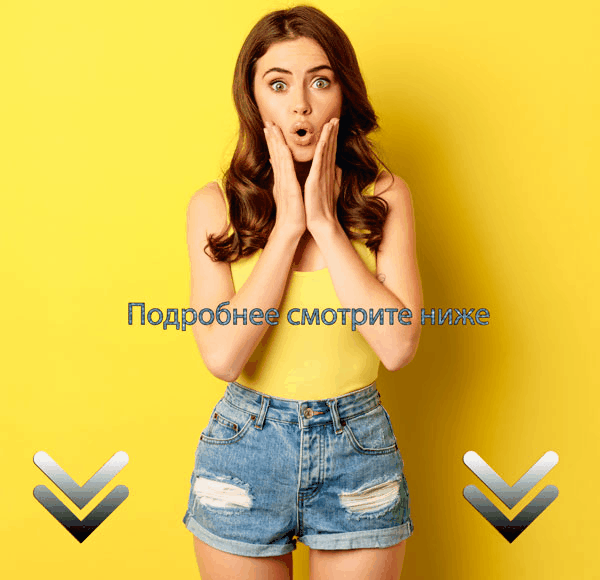
⚡ ПОДРОБНЕЕ ЖМИТЕ ЗДЕСЬ 👈🏻👈🏻👈🏻
Галерея 2923425
All Books Conferences Courses Journals & Magazines Standards Authors Citations
An illustration of a UWSN architecture.
Abstract: In recent research on 3D underwater wireless sensor network (UWSN), magnetic induction communication is a promising candidate, thanks to several unique features, such as ... View more
In recent research on 3D underwater wireless sensor network (UWSN), magnetic induction communication is a promising candidate, thanks to several unique features, such as small transmission delay, constant channel behavior, and adequate long communication range. However, designing a routing protocol that prolongs the network lifetime and reduces the transmission delay has been still a challenge for a 3D UWSN. In this paper, we propose an efficient routing protocol based on reinforcement learning, in particular, the Q-learning that aims to investigate the resource management in the hierarchical networks. Through defining the single hopping bonus metrics of distance and energy, we deduce the updating formula of the routing algorithm and derive the relationship between energy priority and distance priority. In addition, we set up a regulatory factor to adjust the proportion between energy saving and low delay, and thus, it can meet different needs. The simulation results show that the proposed routing approach outperforms the conventional protocol in extending the network lifetime and reducing the transmission delay.
Published in: IEEE Access ( Volume: 7 )
An illustration of a UWSN architecture.
TABLE 1
Comparison of Underwater Communication Technologies
TABLE 2
State-of-the-Art Related Work
TABLE 3
Overview of Mathematical Notations Used in This Paper
M. Stojanovic and J. Preisig, "Underwater acoustic communication channels Propagation models and statistical characterization", IEEE Commun. Mag. , vol. 47, pp. 84-89, Jan. 2009.
S. Ahmed, N. Javaid, F. A. Khan, M. Y. Durrani, A. Ali, A. Shaukat, et al., "Co-UWSN Cooperative energy-efficient protocol for underwater WSNs", Int. J. Distrib. Sensor Netw. , vol. 11, no. 4, pp. 1-16, Sep. 2015.
M. C. Domingo, "Magnetic induction for underwater wireless communication networks", IEEE Trans. Antennas Propag. , vol. 60, no. 6, pp. 2929-2939, Jun. 2012.
H. Guo, Z. Sun and P. Wang, "Multiple frequency band channel modeling and analysis for magnetic induction communication in practical underwater environments", IEEE Trans. Veh. Technol. , vol. 66, no. 8, pp. 6619-6632, Aug. 2017.
I. F. Akyildiz, P. Wang and Z. Sun, "Realizing underwater communication through magnetic induction", IEEE Commun. Mag. , vol. 53, no. 11, pp. 42-48, Nov. 2015.
B. Gulbahar and O. B. Akan, "A communication theoretical modeling and analysis of underwater magneto-inductive wireless channels", IEEE Trans. Wireless Commun. , vol. 11, no. 9, pp. 3326-3334, Sep. 2012.
Z. Sun, I. F. Akyildiz, S. Kisseleff and W. Gerstacker, "Increasing the capacity of magnetic induction communications in RF-challenged environments", IEEE Trans. Commun. , vol. 61, no. 9, pp. 3943-3952, Sep. 2013.
Z. Sun and I. F. Akyildiz, "Optimal deployment for magnetic induction-based wireless networks in challenged environments", IEEE Trans. Wireless Commun. , vol. 12, no. 3, pp. 996-1005, Mar. 2013.
S. Wang, T. L. N. Nguyen and Y. Shin, "Data collection strategy for magnetic induction based monitoring in underwater sensor networks", IEEE Access , vol. 6, pp. 43644-43653, 2018.
J. Jiang, K. Song, G. Wei, R. Lu, Q. Zhang and C. Zhu, "Capacity and bandwidth analysis of symmetric two-coil resonant magnetic communication using frequency divarication", IEEE Antennas Wireless Propag. Lett. , vol. 14, pp. 370-373, 2015.
S. Rani, S. H. Ahmed, J. Malhotra and R. Talwar, "Energy efficient chain based routing protocol for underwater wireless sensor networks", J. Netw. Comput. Appl. , vol. 92, pp. 42-50, Aug. 2017.
C.-F. Cheng and L.-H. Li, "Data gathering problem with the data importance consideration in underwater wireless sensor networks", J. Netw. Comput. Appl. , vol. 78, pp. 300-312, Jan. 2017.
N. Javaid, T. Hafeez, Z. Wadud, N. Alrajeh, M. S. Alabed and N. Guizani, "Establishing a cooperation-based and void node avoiding energy-efficient underwater WSN for a cloud", IEEE Access , vol. 5, pp. 11582-11593, 2017.
F. Kiani, "Reinforcement learning based routing protocol for wireless body sensor networks", Proc. IEEE SC2 , pp. 71-78, Mar. 2017.
F. Farahnakian, M. Ebrahimi, M. Daneshtalab, P. Liljeberg and J. Plosila, "Q-learning based congestion-aware routing algorithm for on-chip network", Proc. IEEE NESEA , pp. 1-7, Dec. 2011.
S. Kim, J. Son, A. Talukder and C. S. Hong, "Congestion prevention mechanism based on Q-leaning for efficient routing in SDN", Proc. ICOIN , pp. 124-128, Jan. 2016.
F. Hajiaghajani and S. Biswas, "Towards scalable and privacy preserving commercial content dissemination in social wireless networks", Proc. IEEE PIMRC , pp. 1-7, Feb. 2017.
S. Wang, T. L. N. Nguyen and Y. Shin, "Energy-efficient clustering algorithm for magnetic induction-based underwater wireless sensor networks", IEEE Access , vol. 7, pp. 5975-5983, 2019.
Z. Sun and I. F. Akyildiz, "Magnetic induction communications for wireless underground sensor networks", IEEE Trans. Antennas Propag. , vol. 58, no. 7, pp. 2426-2435, Jul. 2010.
M. Zorzi, P. Casari, N. Baldo and A. F. Harris, "Energy-efficient routing schemes for underwater acoustic networks", IEEE J. Sel. Areas Commun. , vol. 26, no. 9, pp. 1754-1766, Dec. 2008.
H. van Hasselt, A. Guez and D. Silver, "Deep reinforcement learning with double Q-learning", Proc. AAAI , pp. 2094-2100, Feb. 2016.
C. Wu, Y. Ji, F. Liu, S. Ohzahata and T. Kato, "Toward practical and intelligent routing in vehicular ad hoc networks", IEEE Trans. Veh. Technol. , vol. 64, no. 12, pp. 5503-5519, Dec. 2015.
W.-S. Jung, J. Yim and Y.-B. Ko, "QGeo Q-learning-based geographic ad hoc routing protocol for unmanned robotic networks", IEEE Commun. Lett. , vol. 21, no. 10, pp. 2258-2261, Oct. 2017.
F. Li, X. Song, H. Chen, X. Li and Y. Wang, "Hierarchical routing for vehicular ad hoc networks via reinforcement learning", IEEE Trans. Veh. Technol. , vol. 68, no. 2, pp. 1852-1865, Feb. 2019.
S. Wang and Y. Shin, "3D-deployment of magnetic induction relays in underwater sensor networks", Proc. IEEE ICOIN , pp. 222-226, May 2019.
IEEE Account
Change Username/Password
Update Address
Purchase Details
Payment Options
Order History
View Purchased Documents
Need Help?
US & Canada: +1 800 678 4333
Worldwide: +1 732 981 0060
Contact & Support
Owing to several unique features such as negligible signal propagation delay, predictable and constant channel response, and stealth underwater operations, MI communication technology is attracting more attention in today’s UWSNs [5] . In the last decade, MI communication has made great progress, which was mainly divided into three categories; theories of the MI communication, deployment of MI coils, and physical experiments. The path loss of MI waves, bit error rate and other communication metrics in underwater environments have been addressed in [3] and [6] . Through designing a near-field MI transmission model, [3] investigated the path loss and the bit error rate, and found that the distance of MI communication could be extended to hundreds of meters in freshwater environments. A 3D underwater network covering hundreds of meters depth and several km 2 area was studied in [6] . These results proved that the MI communication could be applied to UWSNs very well. In recent research on the deployment of MI coils, the researchers mainly focused on the 1D and 2D networks. For instance, [5] – [7] analyzed the 1D network where multiple relay coils were located along a polygonal line. This was because the location of the transmitter and the receiver often determined the distribution of MI coils in 1D networks. To solve the relay deployment issue in the MI-based networks, [8] proposed the optimal MI waveguide deployment strategies (i.e., minimum spanning tree (MST) algorithm and Voronoi-Fermat (VF) algorithm) which reduced the cost and enhanced the network robustness.
Recently, [9] presented a novel deployment strategy based on a 3D random distribution with hexagonal characteristics for 3D UWSNs. This method introduced three small random variables that were added to the coordinates of sensor nodes following honeycomb distribution. In this way, the influence of seafloor terrain could be considered. Besides, [4] and [10] verified their proposed MI communication models in the form of experiments for underwater networks. However, one of the biggest challenges of the MI communication in underwater environments is how to design effective strategies of data collection. At present, people mainly use autonomous underwater vehicles (AUVs) and multi-hop routing to gather data. In [9] and [11] – [13] , the authors investigated several routing protocols for UWSNs. Both [9] and [11] divided the underwater network into several clusters and then utilized AUVs and multi-hop transmission to collect data from the cluster head, respectively. Utilization of AUVs led to a huge end-to-end delay if the traveling path was too long. To mitigate the imbalance in the energy consumption and to reduce the delivering delay time, [12] proposed two algorithms, i.e., the data gathering algorithm for sensors (DGS) and the data gathering algorithm for AUV (DGA), based on the combination of AUVs and multi-hop transmission. In addition, the avoiding void node with adaptive hop-by-hop vector based forwarding (AVN-AHH-VBF) and the cooperation-based AVN-AHH-VBF (CoAVN-AHH-VBF) [13] were proposed to improve the utilization rate of resources, but one sink gathering data could lead to an uneven energy distribution. In Table 2 , we report a list of the state-of-the-art related work.
Although above methods have good performance in extending the network or reducing the delivering delay, there are still some issues that need to be improved. We wonder such a routing protocol in which the network lifetime and the transmission delay are considered at the same time. For this issue, the reinforcement learning is an effective method. Especially, Q-learning has been applied to many scenes, i.e., network congestion and maximum reward. In [14] – [16] , the authors designed less congested paths based on the Q-learning to reduce transmission latency and power consumption. To disseminate the maximum commercial content in social wireless networks, [17] proposed a Q-learning based device-to-device multicast routing framework. However, these algorithms did not consider the characteristics of the underwater communication, thus they could not be applied to UWSNs. In this paper, we propose a Q-learning based energy-delay routing (QL-EDR) algorithm that aims to improve the energy efficiency and reduce the delivering delay for 3D UWSNs. The main contributions of this paper are summarized as follows.
Based on the 3D random distribution with hexagonal characteristics [9] , hierarchical underwater communication network is constructed.
Two performance metrics of balancing the remaining energy and reducing the transmission delay are added to the QL-EDR algorithm.
Via setting the regulatory factor, we obtain the relationship between two performance metrics. Thus, we can adjust it to prolong the network lifetime or to reduce the transmission delay according to the actual demand.
The remainder of this paper is organized as follows. In Section II , we describe the MI communication network. The routing protocol based on the Q-learning is presented in Section III . Section IV evaluates the performance of the proposed routing protocol. Finally, conclusions are given in Section V .
There are many factors that affect the network lifetime and the data collection efficiency such as node deployment, data aggregation pattern, and model of data processing. In this section, we introduce a UWSN architecture from four aspects that are network architecture, data processing framework, data packet structure and energy consumption.
As shown in Fig. 1 , a hierarchical approach is used to deploy sensor nodes in a 3D UWSN where each network layer is equipped with the same number of sensor nodes following the 3D random distribution with hexagonal characteristics [9] . However, this does not mean all nodes are distributed in the same plane, but in a certain space. The number of layers in the network is determined by the node’s maximum communication distance and seabed depth. In addition, the initial battery capacity of sensor nodes in different layers may be different. According to the amount of work, these underlying and top-layer nodes are equipped with more initial power. Meanwhile those nodes in the relay-layer do not need much energy, since they only forward the data from underlying layer to top layer. It should be noted that the initial energy of nodes in each layer is the same. Specifically, the battery capacity of the sensor node is set based on its functionality and the amount of work. Of course, if the upper layer networks are just used to transmit the data from the source layer, the entire network can adopt the same sensor nodes including the same initial energy and the same modem. To avoid the short signal overloading, we set the maximum input threshold to choose a suitable wireless transmitter gain. For those network layers that sense or monitor environmental information, the improved high energy node priority clustering (IHENPC) algorithm [18] is utilized to divide the network into several clusters. Because results show the IHENPC algorithm has a good performance in balancing the remaining energy of node and avoiding premature appearance of energy holes. On the other hand, the data is transmitted to the base station through the sinks in a multi-hop manner.
An illustration of a UWSN architecture.
In the UWSN, those sinks serving the base station and lower sensor nodes are equipped with a more powerful function of processing data compared to the sensor node. One of the important tasks is that they need to feedback the routing table to the entire network. In the base station, a reinforcement learning client is to plan an efficient routing of data aggregation. Fig. 2 shows the data processing framework including three parts: data collection, data processing, and decision management. During the data collection process, each network layer chooses several cluster heads to divide itself into different clusters. Simultaneously, the monitoring information will be transmitted from the sensor nodes to their cluster heads, and then converged to the base station via a multi-hop route in inter-layers. After the client extracts the features from the database, we can obtain two important performance metrics: routing timeliness and energy consumption from the data processing. Finally, the reinforcement learning is used to design efficient multi-hop paths from the source nodes to the base station for the next round of data collection according to these two performance metrics.
An overview of the data processing framework in the UWSN.
The way to collect the data within the cluster has a huge impact on the network lifetime. A good data collection method not only prolongs the network lifetime, but also improves the efficiency of data collection. To reduce the energy consumption, sensor nodes should work in a low power sleeping state. Fig. 3 shows the process of collecting the data in which the horizontal axis represents the timeline. Each round includes two phases: a sleeping phase and an operating phase. They adopt different data frames (e.g., frame control, sequence number, source address, destination address) to deal with the data. During the sleeping phase, each node is in a sleeping (standby) state with duration T_{s}
(using TDMA). After waking up, these nodes listen to the channel state to determine whether they need to send or receive data. To improve the communication quality, the time synchronization of sleep and listening should be ensured between adjacent nodes. During the operating phase, the cluster head first broadcasts a scheduling information to keep the time synchronization among nodes and allocates different time slots to different nodes for data aggregation. At every time slot, there is only one sensor node that forwards the data. After finishing receiving the data chunk with duration h
, the cluster head will communicate with the next node. When the cluster head receives all the data in the cluster, it will check whether each data packet is complete. If someone is lost due to network congestion or differences in the sampling period, the cluster head will re-request that node to send the data. Finally, when the cluster head receives the complete data, it will select a multi-hop path based on the routing table.
An overview of the data packet structure in the UWSN.
In the UWSN, the node localization is an important aspect. In particular, AUVs require the accurate coordinate of sensor nodes to collect the data. The global positioning system (GPS) is infeasible in the underwater environment, which brings several great challenges for designing the underwater localization scheme. There are three kinds of underwater methods, i.e., acoustic-based, dead-reckoning and MI-based ones. Due to the limitations of the former two methods, the underwater MI communication usually adopts the received magnetic field strength (RMFS)-based localization method [5] where omnidirectional MI antennas are used to locate the position of nodes based on RMFS. An omnidirectional antenna consists of three coils that are perpendicular to each other, so that it improves the accuracy of the localization scheme.
The matrices, vectors and mathematical notations used in this paper are listed in Table 3 .
In different network layers, data aggregation occurs at the same time. This means that the source nodes need more time to forward the data. Thus, we must use the average end-to-end delay to test the efficiency of data collection.
In this section, we introduce the Q-learning algorithm and the proposed routing algorithm based on reinforcement learning for 3D UWSNs in details.
The Q-learning is a reinforcement learning technique, which can obtain an optimal action-selection policy and the maximum reward from the delay reward without a specific environment model [21] . Based upon the feedback, three main variables are considered in the Q-learning.
s
: Discrete environment state, i.e., the position of the sensor node.
a
: Discrete agent action, i.e., the next hopping node accessible.
R
: Discrete environmental reward. It is calculated by a reward function about the transmission distance.
The details of the QL-EDR algorithm are presented in Algorithm 1 . In actual operation, every node maintains a Q-value and other network information. Before data aggregation, the BS assigns tasks by broadcasting. Simultaneously, all sensor nodes update their Q-value based on the formula (18) from the top-layer to the underlying layer. According to the final updating Q-table, we design those paths with the maximum reward in Algorithm 2 . For all next hopping nodes accessible, the node will choose the next hopping node with the maximum Q-value. If a node has no next hopping nodes, this means that the node lies in a void region in the direction of the base station. Under this circumstance, this node will choose a node with the maximum remaining energy as the next hopping node from all connectable nodes, i.e., nodes within the communication range.
In this section, we investigate the numerical performance of our proposed routing algorithm
Эта пухлая мамочка любит ебаться с молодыми парнями
Сексуальной телке нужна работа
Эми Грин снимает униформу медсестры