Галерея 2876860
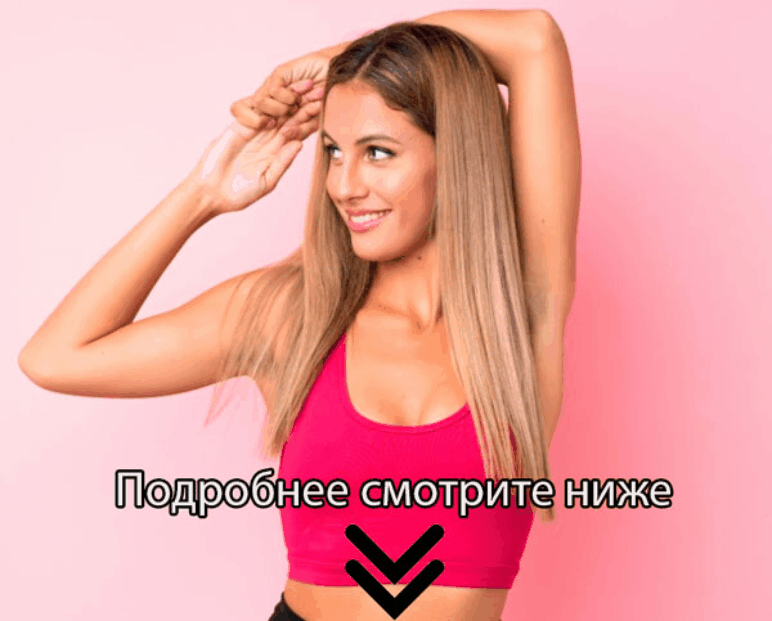
⚡ ПОДРОБНЕЕ ЖМИТЕ ЗДЕСЬ 👈🏻👈🏻👈🏻
Галерея 2876860
All Books Conferences Courses Journals & Magazines Standards Authors Citations
Evolutions of accuracy by GMB on LETTER-HIGH in IAM graph database repository. The evolutions showed that the accuracy increased steadily, result in 25.0 improvements aft... View more
Abstract: This paper presents a novel method for structural data recognition using a large number of graph models. In general, prevalent methods for structural data recognition hav... View more
This paper presents a novel method for structural data recognition using a large number of graph models. In general, prevalent methods for structural data recognition have two shortcomings: 1) only a single model is used to capture structural variation and 2) naive classifiers are used, such as the nearest neighbor method. In this paper, we propose strengthening the recognition performance of these models as well as their ability to capture structural variation. The main contribution of this paper is a novel approach to structural data recognition: graph model boosting. We construct a large number of graph models and train a strong classifier using the models in a boosting framework. Comprehensive structural variation is captured with a large number of graph models. Consequently, we can perform structural data recognition with powerful recognition capability in the face of comprehensive structural variation. The experiments using IAM graph database repository show that the proposed method achieves impressive results and outperforms existing methods.
Published in: IEEE Access ( Volume: 6 )
Date of Publication: 21 October 2018
Evolutions of accuracy by GMB on LETTER-HIGH in IAM graph database repository. The evolutions showed that the accuracy increased steadily, result in 25.0 improvements aft... View more
TABLE 1
Summary of Characteristics of Datasets Used in the Experiments. The Top Row Indicates the Number of Training Data Items, Validation Data, Test Data, Classes, Attribute Types of Vertex and Edge, Average Numbers of Vertices and Edges, Maximum Number of Vertices and Edges, and the Number of Graphs in a Class
TABLE 2
Recognition Rates (%) of Median Graph and PAGGM Using the NN Method On the Test Data
TABLE 3
Average Numbers of Weak Classifiers Voting to Correct and Other Classes. The Maximum Number is 100 Since 100 Rounds are Proceeded
Table 4
Comparison of Recognition Rate
Table 5
The Details of Processing Times (Sec/Round) in GMB
D. White and R. C. Wilson, "Parts based generative models for graphs", Proc. Int. Conf. Pattern Recognit. , pp. 1-4, Dec. 2008.
L. Han, L. Rossi, A. Torsello, R. C. Wilson and E. R. Hancock, "Information theoretic prototype selection for unattributed graphs" in Structural Syntactic and Statistical Pattern Recognition, Berlin, Germany:Springer, vol. 7626, pp. 33-41, 2012.
B. Zhang et al., "Multi-class graph boosting with subgraph sharing for object recognition", Proc. 20th Int. Conf. Pattern Recognit. , pp. 1541-1544, Aug. 2010.
K. Riesen and H. Bunke, "Approximate graph edit distance computation by means of bipartite graph matching", Image Vis. Comput. , vol. 27, pp. 950-959, Jun. 2009.
H. Bunke and K. Riesen, "Towards the unification of structural and statistical pattern recognition", Pattern Recognit. Lett. , vol. 33, no. 7, pp. 811-825, May 2012.
C. F. Moreno-García, F. Serratosa and X. Jiang, "An edit distance between graph correspondences", Proc. Graph-Based Represent. Pattern Recognit. , pp. 232-241, 2017.
T. Miyazaki and S. Omachi, "Graph model boosting for structural data recognition", Proc. 23rd Int. Conf. Pattern Recognit. , pp. 1708-1713, 2016.
J. R. Ullmann, "An algorithm for subgraph isomorphism", J. ACM , vol. 23, no. 1, pp. 31-42, Jan. 1976.
D. E. Ghahraman, A. K. C. Wong and T. Au, "Graph optimal monomorphism algorithms", IEEE Trans. Syst. Man Cybern. , vol. 10, no. 4, pp. 181-188, Apr. 1980.
M. A. Eshera and K.-S. Fu, "A graph distance measure for image analysis", IEEE Trans. Syst. Man Cybern. , vol. SMC-14, no. 3, pp. 398-408, May/Jun. 1984.
A. Sanfeliu and K.-S. Fu, "A distance measure between attributed relational graphs for pattern recognition", IEEE Trans. Syst. Man Cybern. , vol. SMC-13, no. 3, pp. 353-362, May/Jun. 1983.
M. Neuhaus, K. Riesen and H. Bunke, "Fast suboptimal algorithms for the computation of graph edit distance", Proc. Int. Conf. Struct. Syntactic Statist. Pattern Recognit. , pp. 163-172, 2006.
P. E. Hart, N. J. Nilsson and B. Raphael, "A formal basis for the heuristic determination of minimum cost paths", IEEE Trans. Syst. Sci. Cybern. , vol. 4, no. 2, pp. 100-107, Jul. 1968.
J. Munkres, "Algorithms for the assignment and transportation problems", J. Soc. Ind. Appl. Math. , vol. 5, no. 1, pp. 32-38, 1957.
S. Bougleux, B. Gaüzère and L. Brun, "A Hungarian algorithm for error-correcting graph matching", Proc. Graph-Based Represent. Pattern Recognit. , pp. 118-127, 2017.
K. Riesen, A. Fischer and H. Bunke, "Improved graph edit distance approximation with simulated annealing", Proc. Graph-Based Represent. Pattern Recognit. , pp. 222-231, 2017.
D. Conte, P. Foggia, C. Sansone and M. Vento, "Thirty years of graph matching in pattern recognition", Int. J. Pattern Recognit. Artif. Intell. , vol. 18, no. 3, pp. 265-298, 2004.
P. Bille, "A survey on tree edit distance and related problems", Theor. Comput. Sci. , vol. 337, no. 1, pp. 217-239, Jun. 2005.
X. Jiang, A. Münger and H. Bunke, "On median graphs: Properties algorithms and applications", IEEE Trans. Pattern Anal. Mach. Intell. , vol. 23, no. 10, pp. 1144-1151, 2001.
A. K. C. Wong and M. You, "Entropy and distance of random graphs with application to structural pattern recognition", IEEE Trans. Pattern Anal. Mach. Intell. , vol. PAMI-7, no. 5, pp. 599-609, Sep. 1985.
A. D. Bagdanov and M. Worring, "First order Gaussian graphs for efficient structure classification", Pattern Recognit. , vol. 36, no. 6, pp. 1311-1324, Jun. 2003.
F. Serratosa, R. Alquézar and A. Sanfeliu, "Function-described graphs for modelling objects represented by sets of attributed graphs", Pattern Recognit. , vol. 36, no. 3, pp. 781-798, Mar. 2003.
A. Sanfeliu, F. Serratosa and R. Alquézar, "Second-order random graphs for modeling sets of attributed graphs and their application to object learning and recognition", Int. J. Pattern Recognit. Artif. Intell. , vol. 18, no. 3, pp. 375-396, 2004.
A. Torsello and E. R. Hancock, "Learning shape-classes using a mixture of tree-unions", IEEE Trans. Pattern Anal. Mach. Intell. , vol. 28, no. 6, pp. 954-967, Jun. 2006.
J. Rissanen, "Modeling by shortest data description", Automatica , vol. 14, no. 5, pp. 465-471, 1978.
A. Torsello, "An importance sampling approach to learning structural representations of shape", Proc. IEEE Conf. Comput. Vis. Pattern Recognit. , pp. 1-7, Jun. 2008.
J. M. Hammersley and D. C. Handscomb, Monte Carlo Methods, Hoboken, NJ, USA:Wiley, 1964.
L. Han, R. C. Wilson and E. R. Hancock, "A supergraph-based generative model", Proc. Int. Conf. Pattern Recognit. , pp. 1566-1569, Aug. 2010.
H. He and A. K. Singh, "Closure-tree: An index structure for graph queries", Proc. Int. Conf. Data Eng. , pp. 38, Apr. 2006.
B. J. Jain and F. Wysotzki, "Central clustering of attributed graphs", Mach. Learn. , vol. 56, no. 1, pp. 169-207, 2004.
V. N. Vapnik, Statistical Learning Theory, Hoboken, NJ, USA:Wiley, 1998.
T. Kudo, E. Maeda and Y. Matsumoto, "An application of boosting to graph classification", Proc. 17th Adv. Neural Inf. Process. Syst. , pp. 729-736, 2005.
S. Nowozin, K. Tsuda, T. Uno, T. Kudo and G. Bakir, "Weighted substructure mining for image analysis", Proc. IEEE Conf. Comput. Vis. Pattern Recognit. , pp. 1-8, Jun. 2007.
T. G. Dietterich and G. Bakiri, "Solving multiclass learning problems via error-correcting output codes", J. Artif. Intell. Res. , vol. 2, no. 1, pp. 263-286, Jan. 1995.
M. Cho, J. Lee and K. Lee, "Reweighted random walks for graph matching" in Computer Vision—ECCV, Berlin, Germany:Springer, vol. 6315, pp. 492-505, 2010.
M. Cho, K. Alahari and J. Ponce, "Learning graphs to match", Proc. IEEE Int. Conf. Comput. Vis. , pp. 25-32, Dec. 2013.
T. Cour, P. Srinivasan and J. Shi, "Balanced graph matching", Proc. 19th Adv. Neural Inf. Process. Syst. , pp. 313-320, 2007.
S. Gold and A. Rangarajan, "A graduated assignment algorithm for graph matching", IEEE Trans. Pattern Anal. Mach. Intell. , vol. 18, no. 4, pp. 377-388, Apr. 1996.
L. Han, R. C. Wilson and E. R. Hancock, "Generative graph prototypes from information theory", IEEE Trans. Pattern Anal. Mach. Intell. , vol. 37, no. 10, pp. 2013-2027, Oct. 2015.
H. Bunke, P. Foggia, C. Guidobaldi and M. Vento, "Graph clustering using the weighted minimum common supergraph" in Graph Based Representations in Pattern Recognition, Berlin, Germany:Springer, vol. 2726, pp. 235-246, 2003.
Y. Freund and R. E. Schapire, "A decision-theoretic generalization of on-line learning and an application to boosting", J. Comput. Syst. Sci. , vol. 55, no. 1, pp. 119-139, Aug. 1997.
J. Zhu, H. Zou, S. Rosset and T. Hastie, "Multi-class AdaBoost", Statist. Interface , vol. 2, no. 3, pp. 349-360, 2009.
E. L. Allwein, R. E. Schapire and Y. Singer, "Reducing multiclass to binary: A unifying approach for margin classifiers", J. Mach. Learn. Res. , vol. 1, pp. 113-141, Sep. 2001.
L. Breiman, Classification and Regression Trees, London, U.K.:Chapman & Hall, 1984.
K. Riesen and H. Bunke, "IAM graph database repository for graph based pattern recognition and machine learning", Proc. Int. Workshop Struct. Syntactic Statist. Pattern Recognit. , pp. 287-297, 2008.
A. Solé-Ribalta, X. Cortś and F. Serratosa, "A comparison between structural and embedding methods for graph classification" in Structural Syntactic and Statistical Pattern Recognition, Berlin, Germany:Springer, vol. 7626, pp. 234-242, 2012.
S. A. Nene, S. K. Nayar and H. Murase, "Columbia object image library: Coil-100", 1996.
D. Comaniciu and P. Meer, "Robust analysis of feature spaces: Color image segmentation", Proc. IEEE Conf. Comput. Vis. Pattern Recognit. , pp. 750-755, Jun. 1997.
J. Kazius, R. McGuire and R. Bursi, "Derivation and validation of toxicophores for mutagenicity prediction", J. Med. Chem. , vol. 48, no. 1, pp. 312-320, 2005.
H. M. Berman et al., "The protein data bank", Nucl. Acids Res. , vol. 28, no. 1, pp. 235-242, 2000.
F. B. Silva, R. de O. Werneck, S. Goldenstein, S. Tabbone and R. S. da Torres, "Graph-based bag-of-words for classification", Pattern Recognit. , vol. 74, pp. 266-285, Feb. 2018.
K. Riesen and H. Bunke, "Reducing the dimensionality of dissimilarity space embedding graph kernels", Eng. Appl. Artif. Intell. , vol. 22, no. 1, pp. 48-56, 2009.
K. Riesen and H. Bunke, "Graph classification by means of Lipschitz embedding", IEEE Trans. Syst. Man Cybern. B Cybern. , vol. 39, no. 6, pp. 1472-1483, Dec. 2009.
L. Livi, A. Rizzi and A. Sadeghian, "Optimized dissimilarity space embedding for labeled graphs", Inf. Sci. , vol. 266, pp. 47-64, May 2014.
IEEE Account
Change Username/Password
Update Address
Purchase Details
Payment Options
Order History
View Purchased Documents
Need Help?
US & Canada: +1 800 678 4333
Worldwide: +1 732 981 0060
Contact & Support
Structural data represented by graphs are a general and powerful representation of objects and concepts. A molecule of water can be represented as a graph with three vertices and two edges, where the vertices represent hydrogen and oxygen, and the relation is intuitively described by the edges. Structural data recognition is used in a wide range of applications; for example, in handwritten characters, symbols from architectural and electronic drawings, images, bioinformatics, and chemical compounds need to be recognized.
Graph recognition is not straightforward. Even measuring the distance between graphs requires various techniques. The problem of graph recognition has recently been actively studied [1] – [6] . Past research has led to two notable progress in two aspects. First, graph models have been developed to capture structural variations. Second, the embedding of graphs into Euclidean space has been used to apply sophisticated classifiers in the vector domain. However, both these aspects have drawbacks. The drawback of the former is that only naive classifiers are applicable, such as the nearest neighbor (NN) or the k-nearest neighbor (k-NN) methods. The drawback of the second aspect above is the loss of structural variation in the classifiers through embedding process. 1
Our aim in this paper is to overcome the drawbacks of the previous methods. The challenge is how to integrate structural variation into a sophisticated classifier. Inspired by boosting algorithms, we construct a strong classifier by aggregating naive classifiers which use graph models. Hence, the strong classifier can be equipped with comprehensive structural variations because it includes a large number of graph models. Specifically, we construct graph models with weighted training graphs and then train a naive classifier using the models. We update the weight so that we can focus on various graphs. Finally, we construct a strong classifier by aggregating the naive classifiers. We call this novel approach graph model boosting (GMB).
The main contribution of this paper is a novel approach to simultaneously strengthen capability of classification and capturing structural variation. In order to capture structural variation comprehensively, we construct a large number of models in a boosting framework so that the models can contain different structural variations and compensate one another. The capability of classification is strengthened by aggregating naive classifiers constructed with graph models. Consequently, we can equip the classifier with comprehensive structural variation and a powerful recognition capability.
In experiments, we demonstrated structural data recognition using GMB on eight graph datasets that were publicly available. We confirmed that accuracy of GMB notably increased as the boosting process continued. Consequently, the accuracy was comparable with the state-of-the-art methods. The experimental results thus show the effectiveness of the GMB.
A preliminary version of the work reported here was first presented in a conference paper [7] . We consolidate and expand our previous description and results. Firstly, we provide additional technical details concerning the graph model and GMB. Our contributions are highlighted clearly in the Abstract and Introduction. Secondly, we carried out a wider survey about related work to clarify the significance of the proposed method. Lastly, additional experimental results are presented: time complexity to evaluate practicality, the impact of the parameters for robustness assessment to various datasets, and using other graph model to show the capability of GMB. The number of datasets used in the experiments is expanded from five to eight by adding datasets in bioinformatics.
Existing methods for graph recognition can be broadly categorized into three approaches: the one-vs-one approach, the model-based approach, and the embedding approach.
Methods in the one-vs-one approach attempt to classify graphs according to a criterion that can be measured for two graphs, such as graph isomorphism, subgraph isomorphism, and graph edit distance. Two graphs are graph isomorphic if the mapping of their vertices and edges is bijective. Subgraph isomorphism is the case where subgraphs of two graphs satisfy graph isomorphism. Isomorphism is determined by tree search [8] or backtracking [9] . However, it is difficult to determine subgraph isomorphism in case of noisy vertices and edges. Therefore, methods using graph edit distance have been developed. The graph edit distance is defined as the minimum sum of the costs of edit operations that transform the source graph into the target graph by substitution, deletion, and insertion [10] , [11] . Since it is expensive to search every combination of edit operations, approximate edit operations are searched. The method developed in [12] applies the A-star algorithm [13] , the one in [4] exploits Munkres’s algorithm [14] , the one in [15] is based on a Hungarian algorithm, and the one in [16] uses simulated annealing. The literature [17] , [18] will help readers find more details related to graph matching and edit distance. The advantage of this approach is that the calculation is completed in polynomial time. However, the methods in this approach only adopt naive classifiers, such as the NN method and the k-NN method. The performance of NN and k-NN depend on a metric between graphs, hence we need to define it carefully. However, this approach focuses on two graphs. Consequently, the methods measure a metric without structural variation; only two graphs are considered, whereas other graphs are ignored.
Methods in the model-based approach attempt to capture structural variation and classify graphs using a model. The median graph [19] is a model that captures global information concerning graphs. The median graph minimizes the total distance between training graphs. A random graph [20] is a model that specializes in capturing attribute variations at each vertex and edge. The random graph contains variables ranging from 0 to 1, which are associated with attribute values. The variables represent the probabilities of the attribute that the vertices take. However, numerous variables are required when attributes are continuous values. Improved models [21] – [23] based on random graph have been developed as well. There are three such models: First-order Gaussian graph, or FOGG [21] , function-described graph, or FDG [22] , and second-order random graphs, or SORGs [23] . The FOGG is a model designed to avoid increasing number of variables by replacing those of a random graph with parameters of a Gaussian distribution. FDG and SORG incorporate joint probabilities among the vertices and the edges to describe structural information. The difference between FDG and SORG is the numbers of vertices and edges at the calculation of joint probability. FDG uses pairs of vertices or edges, whereas multiple vertices and edges are used in SORG. Recently, models exploiting unsupervised learning methods have been developed [24] . Torsello and Hancock presented a framework to integrate tree graphs into one model by minimizing the minimum description length [25] . Furthermore, Torsello [26] expanded tree graphs to graphs and adopted a sampling strategy [27] to improve calculation efficiency. The EM algorithm has been applied to construct a model [28] . The methods in [24] , [26] , and [28] concentrate on capturing variations in vertex and edge composition. For computational efficiency, a closure tree [29] has been developed. Each vertex of the tree contains information concerning its descendants, so that effective pruning can be carried out. The model-based approach can measure distance based on structural variation. The drawback of this approach is to use NN and k-NN classifiers using only a single model. A metric is important for this approach. However, the methods use only a single model to measure a metric. We stress that minor structural information is lost in a single model. Consequently, a metric is measured by considering only major variation, whereas minor variation is ignored.
Methods in the embedding approach attempt to apply sophisticated classifiers that are widely used in the vector domain. The main obstacle to embedding graphs is the lack of a straightforward and unique transformation from a graph to a vector. For example, a graph with N
vertices can be transformed into N!
Пожилой фотограф кончил в рот молодой блондинке
Губастая шлюха дала сразу двоим
Жирная латина и любовник который лижет у нее жопу