3d Different Ages Anal Waldo
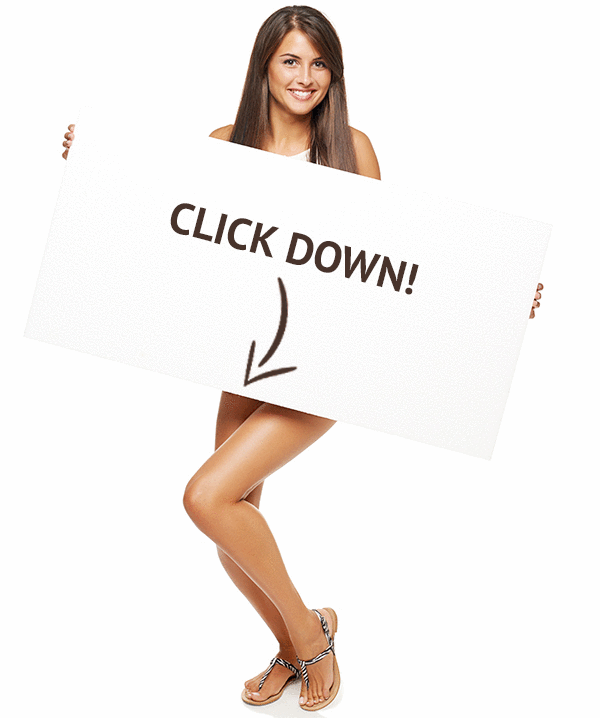
💣 👉🏻👉🏻👉🏻 ALL INFORMATION CLICK HERE 👈🏻👈🏻👈🏻
Your browser does not support the NLM PubReader view.
Go to this page to see a list of supported browsers
or return to the Article in classic view.
A hierarchical 3D-motion learning framework for animal spontaneous behavior mapping
We are experimenting with display styles that make it easier to read articles in PMC. The ePub format uses eBook readers, which have several "ease of reading" features already built in.
The ePub format is best viewed in the iBooks reader. You may notice problems with the display of certain parts of an article in other eReaders.
Generating an ePub file may take a long time, please be patient.
Kang Huang, Yaning Han, [...], and Liping Wang
Animal behavior usually has a hierarchical structure and dynamics. Therefore, to understand how the neural system coordinates with behaviors, neuroscientists need a quantitative description of the hierarchical dynamics of different behaviors. However, the recent end-to-end machine-learning-based methods for behavior analysis mostly focus on recognizing behavioral identities on a static timescale or based on limited observations. These approaches usually lose rich dynamic information on cross-scale behaviors. Here, inspired by the natural structure of animal behaviors, we address this challenge by proposing a parallel and multi-layered framework to learn the hierarchical dynamics and generate an objective metric to map the behavior into the feature space. In addition, we characterize the animal 3D kinematics with our low-cost and efficient multi-view 3D animal motion-capture system. Finally, we demonstrate that this framework can monitor spontaneous behavior and automatically identify the behavioral phenotypes of the transgenic animal disease model. The extensive experiment results suggest that our framework has a wide range of applications, including animal disease model phenotyping and the relationships modeling between the neural circuits and behavior.
Subject terms: Behavioural methods, Computational neuroscience, Animal behaviour
The structure of animal behavior follows a bottom-up hierarchy constructed by time-varying posture dynamics, which has been demonstrated to be classical in ethological theory1,2 and recent animal studies3–6. Such behavioral organization is considered to coordinate with neural activities7,8. Previous studies9–11 using large-scale neuronal recordings have provided preliminary evidence from the neural implementation perspective. As the central goal of modern neuroscience, fully decoding this cross-scale dynamic relationship requires comprehensive quantification of neural activity and behavior. Over the past few decades, scientists have been working on improving the accuracy and throughput of neural dynamics manipulation and capturing. Meanwhile, for behavior quantification, there has been a revolution from simple behavioral parameters extraction to machine-learning (ML)-based behavior sequence recognition12,13. However, most previous methods14,15 often emphasized feature engineering and pattern recognition for mapping raw data to behavioral identities. These black-box approaches lack the interpretability of cross-scale behavioral dynamics. Thus, it is a challenging task, but with a strong demand, to develop a general-purpose framework for the dynamic decomposition of animal spontaneous behavior.
Previous researchers addressed this challenge mainly from two aspects. The first aspect is behavioral feature capturing. Conventional animal behavior experiments usually use a single-camera top-view recording to capture the motion signal of behaving animals, leading to occlusions of the key body parts (e.g., paws), and these are very sensitive to viewpoint differences16. Thus, it is very challenging for the single-camera technology to capture the three-dimensional (3D) motion and then map the spontaneous behavior in a dynamic way. The recent emergence of ML toolboxes17–19 has dramatically facilitated the animal pose estimation with multiple body parts. Thus, it enables us to study the animal kinematics more comprehensively and provides potential applications for capturing 3D animal movements. The second aspect is decomposing continuous time-series data into understandable behavioral modules. Previous studies on lower animals such as flies10,20–22, zebrafishes4,23–25, and Caenorhabditis elegans26–28 utilized ML strategies and multivariate analysis to detect action sequences. However, mammalian behavior is highly complicated. Besides locomotion, animals demonstrate non-locomotor movement (NM) with their limbs (e.g., grooming, rearing, turning), and their organs have high dimensional29–31 and variable spatiotemporal characteristics. Even for similar behaviors, the duration and composition of postural sequences vary. To define the start and end boundaries to segment continuous data into behavioral sequences, many ML-based open-source toolboxes21 and commercial software do excellent work in feature engineering. They usually compute per-frame features that refer to position, velocity, or appearance-based features. The sliding windows technology then converts them into window features to reflect the temporal context14,15. Although these approaches effectively identify specific behaviors, behavior recognition becomes problematic when the dynamics of particular behaviors cannot be represented by the window features.
The present study proposes a hierarchical 3D-motion learning framework to address our contribution to these challenges. First, we acquired the 3D markerless animal skeleton with tens of body parts by the developed flexible and low-cost system. Through the systematic validations, we proved that our system could solve the critical challenges of body occlusion and view disappearance in animal behavior experiments. Second, aiming at the parallel and hierarchical dynamic properties of spontaneous behavior, we proposed a decomposition strategy preserving the behavior’s natural structure. With this strategy, the high-dimensional, time-varying, and continuous behavioral series can be represented as various quantifiable movement parameters and low-dimensional behavior map. Third, we obtained a large sample of the Shank3B−/− mouse disease model data resources with our efficient framework. The results showed that our framework could detect behavioral biomarkers that have been identified previously and discover potential new behavioral biomarkers. Finally, together with the further group analysis of the behavioral monitoring under different experimental apparatus, lighting conditions, ages, and sexes, we demonstrated our framework could contribute to the hierarchical behavior analysis, including postural kinematics characterization, movement phenotyping, and group level behavioral patterns profiling.
In our framework, first we collect the animal postural feature data (Fig. 1a). These data can be continuous body parts trajectories that comprehensively capture the motion of the animal’s limbs and torso, and they inform the natural characteristics of locomotion and NM. Locomotion can be represented by velocity-based parameters. NM is manifested by movement of the limbs or organs without movement of the torso and is controlled by dozens of degrees of freedom32. Hence, we adopted a parallel motion decomposition strategy to extract features from these time-series data independently (Fig. 1b, c). A two-stage dynamic temporal decomposition algorithm was applied to the centralized animal skeleton postural data to obtain the NM space. Finally, together with the additional velocity-based locomotion dimension, unsupervised clustering was used to reveal the structure of the rodent’s behavior.
Hierarchical 3D-motion learning framework for animal behavior analysis.
Our framework has two main advantages. First, it addresses the multi-timescale of animal behavior33. Animal behavior is self-organized into a multi-scale hierarchical structure from the bottom up, including poses, movements, and ethograms34,35. The poses and movements are low- and intermediate-level elements36, while higher-level ethograms are stereotyped patterns composed of movements that adhere to inherent transfer rules in certain semantic environments37. Our two-stage pose and movement decomposition focuses on extracting the NM features of the first two layers. Second, our framework emphasizes the dynamic and temporal variability of behavior. The most critical aspect of unsupervised approaches is to define an appropriate metric for quantifying the relationship between samples. However, the duration and speed of NM segments of the same cluster may differ. To address this, we used a model-free approach called dynamic time alignment kernel (DTAK) as a metric to measure the similarity between the NM segments and thus equip the model to automatically search repeatable NM sequences. We then apply the uniform manifold approximation and projection (UMAP)38 algorithm to visualize high-dimensional NM representations. After combining the locomotion dimension with NM space (Fig. 1c), we adopted hierarchical clustering to re-cluster the components and map the behavior’s spatial structure (Fig. 1d).
To efficiently and comprehensively characterize the kinematics of free-moving animals, we developed a 3D multi-view motion-capture system (Fig. 2a, b) based on recent advances in techniques for pose estimation17 and 3D skeletal reconstruction39. The most critical issues in 3D animal motion capture are efficient camera calibration, body occlusion, and viewpoint disappearance, which have not been optimized or verified12. To address these issues, we developed a multi-view video capture device (Supplementary Fig. 2a). This device integrates the behavioral apparatus, an auto-calibration module (Supplementary Fig. 2b, d), and synchronous acquisition of multi-view video streams (Supplementary Fig. 2c). While the conventional manual method requires half an hour to produce the required checkerboard for calibration, the auto-calibration module can be completed in 1 min.
Collecting animal behavior trajectories via a 3D motion-capture system.
We collected the naturalistic behavioral data of free-moving mice in a featureless circular open field (Supplementary Fig. 2a and Supplementary Movie M1). We analyzed the mouse skeleton as 16 parts (Fig. 2c) to capture the movements of the rodent’s head, torso, paws, and tail. The following motion quantification did not involve the motion features of two parts of the tail. The data obtained from tracking representative mouse poses tracking (Fig. 2c) include the 3D coordinates (x, y, and z) of the body parts, which reveal that the high-dimensional trajectory series exhibits periodic patterns within a specific timescale. We next investigated whether the 3D motion-capture system could reliably track the animal in cases of body-part occlusion and viewpoint disappearance. We checked the DeepLabCut (DLC) tracking likelihood in the collated videos (0.9807 ± 0.1224, Supplementary Fig. 4a) and evaluated the error between the estimated two-dimensional (2D) body parts of every training set frame and the ground truth (0.534 ± 0.005%, Supplementary Fig. 5b). These results indicated that in most cases, four cameras were available for 2D pose tracking. Since 3D reconstruction can be achieved as long as any two cameras obtain the 2D coordinates of the same point in 3D space from different views, the reconstruction failure rate caused by body occlusion and viewpoint disappearances is determined by the number of available cameras. Therefore, we evaluated the average proportion of available cameras in situations of body part occlusion and viewpoint disappearance. The validation results for body-part occlusion show an average reconstruction failure rate of only 0.042% due to body occlusion or inaccurate body-part estimation (Supplementary Fig. 5c). While for viewpoint disappearances, both tests (Supplementary Fig. 6 and Supplementary Movies M4 and M5) proved that our system has a high reconstruction rate for animal body parts. Moreover, the artifact detection and correction features can recover the body parts that failed to be reconstructed. We calculated an overall reconstruction quality (0.9981 ± 0.0010, Fig. 2d) to ensure that the data were qualified for downstream analysis.
Conceptually, behavior adheres to a bottom-up hierarchical architecture (Fig. 3a)34,35, and research has focused on elucidating behavioral component sequences contained in stimuli-related ethograms40. The purpose of the two-stage NM decomposition is to bridge the low-level vision features (postural time-series) to high-level behavioral features (ethograms). The first stage of the decomposition involves extracting postural representations from postural feature data. Since the definition of NM does not involve the animal’s location or orientation, we pre-processed these data through center alignment and rotation transformation (Supplementary Fig. 7). Animal movement is continuous, and due to the high dimensionality of the mammalian skeleton, the behaviorally relevant posture variables are potentially infinite in number12. However, adjacent poses are usually highly correlated and redundant for behavior quantification and analysis1, which is particularly evident in long-term recording. Therefore, for computational efficiency, we adopted a temporal reduction algorithm to merge adjacent, similar poses as postural representations in a local time range.
Dynamic temporal decomposition of multi-scale hierarchical behavior.
In the second stage, NM modules are detected from temporal reduced postural representations. Unlike the static property of poses, mammalian movements have high dimensionality and large temporal variability41: e.g., the contents, phases, and durations of the three pose sequences were not the same (Fig. 3a). Hence, we adopted a model-free approach to dynamically perform temporal aligning and cluster the temporally reduced postural representation data (Fig. 3b)42. This problem is equivalent to providing a d-dimensional time-series of animal postural representations with n frames. Our task decomposes X into m NM segments, each of which belongs to one of the corresponding k behavioral clusters. This method detects the change point by minimizing the error across segments; therefore, dynamic temporal segmentation becomes a problem of energy minimization. An appropriate distance metric is critical for modeling the temporal variability and optimizing the NM segmentation of a continuous postural time-varying series. Although dynamic time warping has commonly been applied in aligning time-series data, it does not satisfy the triangle inequality43. Thus, we used the improved DTAK method to measure the similarity between time sequences and construct an energy equation (objective function) for optimization. The relationship between each pair of segments was calculated with the kernel similarity matrix K (Fig. 3c). DTAK was used to compute the normalized similarity value of K and generate the paired-wise segment kernel matrix T (Fig. 3d).
Because dynamic temporal segmentation is a non-convex optimization problem whose solution is very sensitive to initial conditions, this approach begins with a coarse segmentation process based on the spectral clustering method, which combines the kernel k-means clustering algorithms. To define the timescale of segmentation, the algorithm sets the maximum and minimum lengths to constrain the length of the behavioral component. For the optimization process, a dynamic programming (DP)-based algorithm is employed to perform coordinate descent and minimize energy. For each iteration, the algorithm updates the segmentation boundary and segment kernel matrix until the decomposition reaches the optimal value (Fig. 3e, f). The final segment kernel matrix represents the optimal spatial relationship between these NM segments, which can be further mapped into its feature space in tandem with dimensionality reduction (DR).
We demonstrate the pipeline of this two-stage behavior decomposition (Fig. 3h) in a representative 300-s sample of mouse skeletal data. The raw skeletal traces were segmented into NM slices of an average duration of 0.89 ± 0.29 s. In these segments, a few long-lasting movements occurred continuously, while most others were intermittent (Fig. 3g). The trajectories of these movement slices can reflect the actual kinematics of the behaving animal. For instance, when the animal is immobile, all of its body parts are still; when the animal is walking, its limbs show rapid periodic oscillations. Consistent with our observations, the movements corresponding to the other two opposite NMs, left and right turning, tended to follow opposite trajectories. These preliminary results demonstrated that DTAK could be used for the decomposition and mapping of NMs.
We validated our framework in a single-session experiment with free-moving mouse behavioral data collected with the 3D motion-capture system. First, the two-stage behavioral decomposition strategy decomposed the 15-min experimental data into 936 NM bouts (Supplementary Movie M2). A 936 × 936 segment kernel matrix was then constructed using the DTAK metric. This segment kernel matrix could flexibly represent the relationship and provide insight into the relationships between each behavioral component sequence in their feature space. However, since the 936-D matrix cannot provide an informative visualization of behavioral structure, it is necessary to perform DR on this data. Various DR algorithms have been designed either to preserve the global representation of original data or to focus on local neighborhoods for recognition or clustering44,45. Thus, in animal behavior quantification, we face a trade-off between discretizing behavior to provide a more quantitative analysis and maintaining a global representation of behavior to characterize the potential manifolds of neural-behavioral relationships46. Therefore, we first evaluated the commonly used DR algorithms from the standpoints of preserving either the global or the local structure. The evaluation results show that UMAP can balance both aspects for our data (Supplementary Fig. 8) and provide 2D embeddings of these NM segments. In addition, in our parallel feature fusion framework, the factor of an animal’s interaction with the environment—i.e., velocity—is considered an independent dimension. Together with 2D NM embedding, they construct a spatiotemporal representation of movements (Fig. 4a).
Identify movement phenotypes on single experimental data.
We used an unsupervised clustering algorithm to investigate the behavior’s spatiotemporal representation and identify the movement phenotypes. Most unsupervised clustering require a pre-specified number of clusters, and the number chosen can be data-driven or refer to the context of the practical biological problem47. In the single experimental dat
Mature Hard Anal Porno
Savanna Bond Xxx Kino
Scooby Doo Xxx Parody Part 1
Drunk Sex Porno Hd
Yoga Sex Videos Download
3D Endoanal Ultrasound and Anal Fistula - Full Text View ...
Tridimensional Pelvic Floor Ultrasound and Quality of Life ...
A hierarchical 3D-motion learning framework for animal ...
Dark Horse Comics
Poverty Universe, All Ages for Waldo County, ME ...
Daz 3D
Where's Waldo? : Toys for Ages 11+ : Target
The Little Rascals (film) - Wikipedia
Stock Images, Photos, Vectors, Video, and Music | Shutterstock
Naked Boy photos on Flickr | Flickr
3d Different Ages Anal Waldo


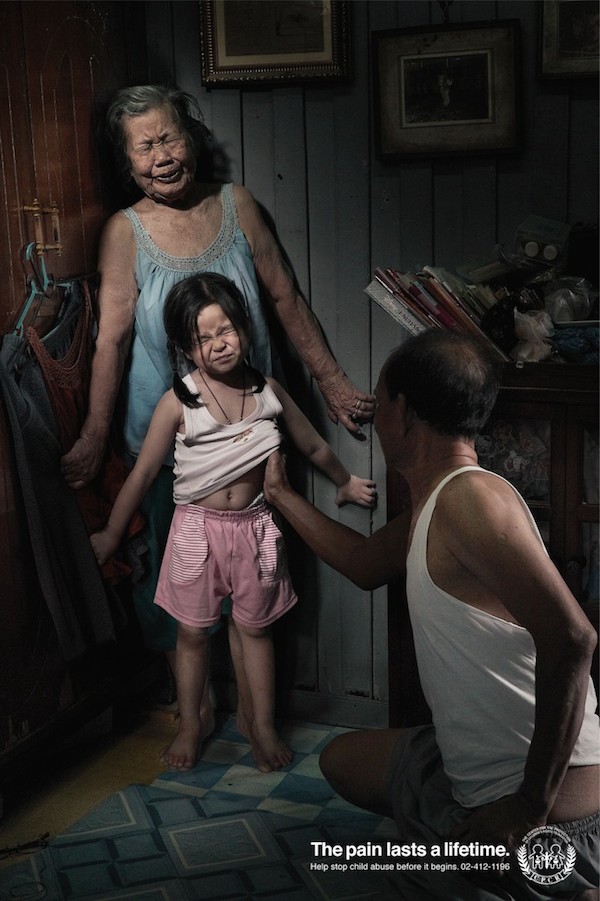
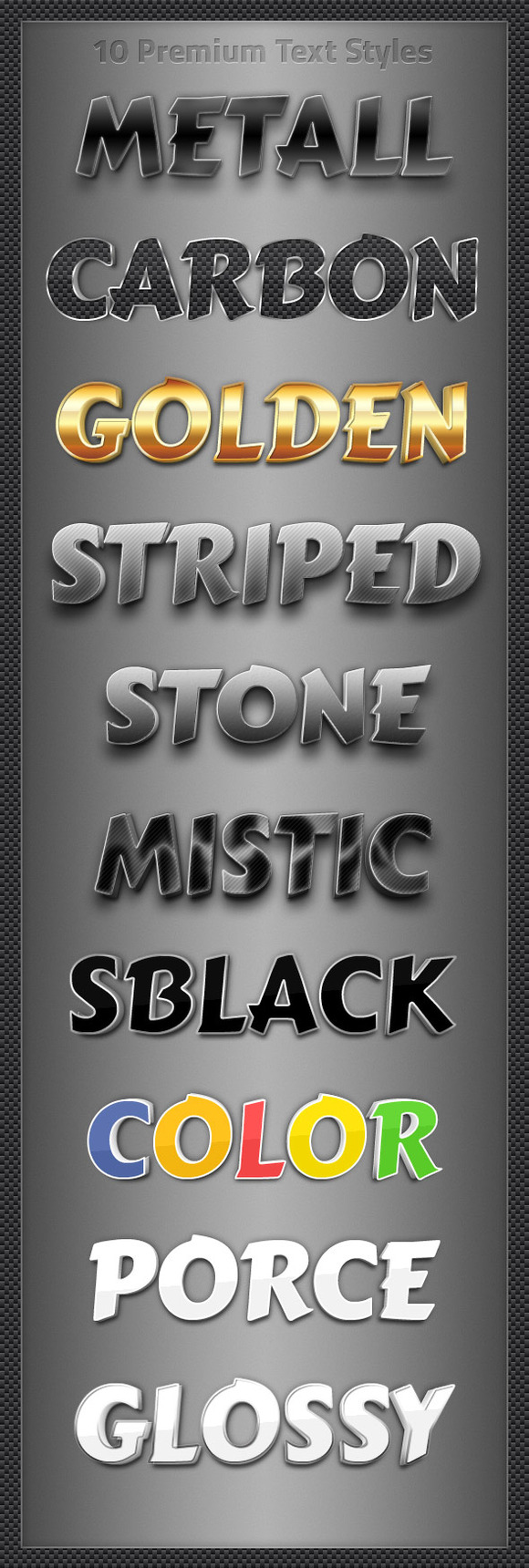
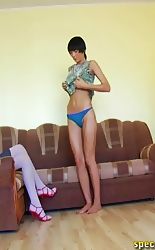

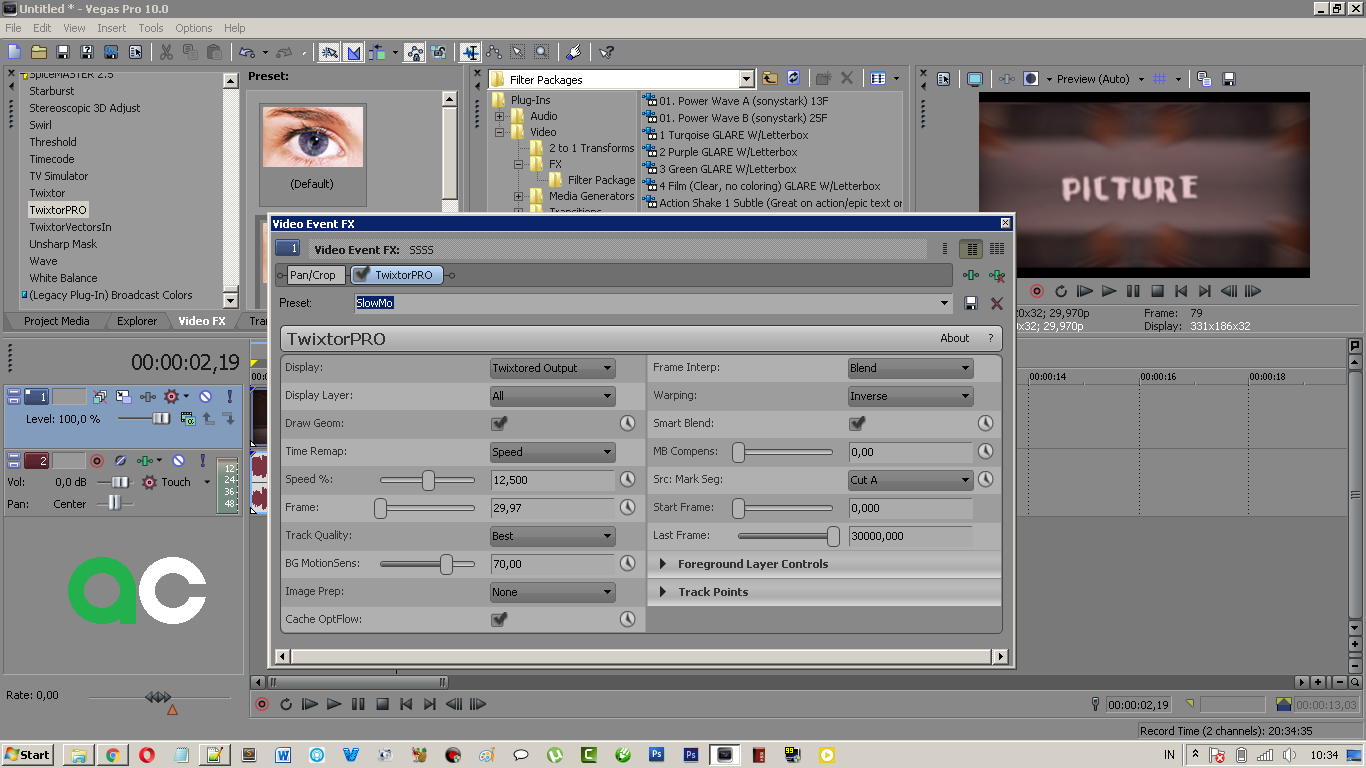



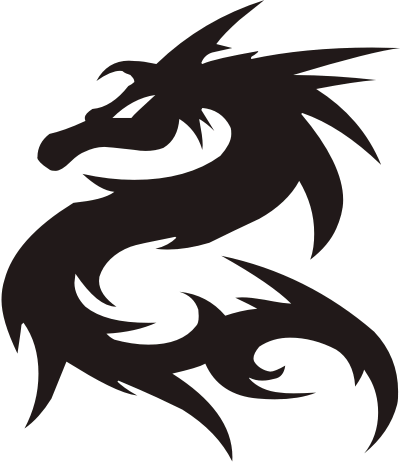


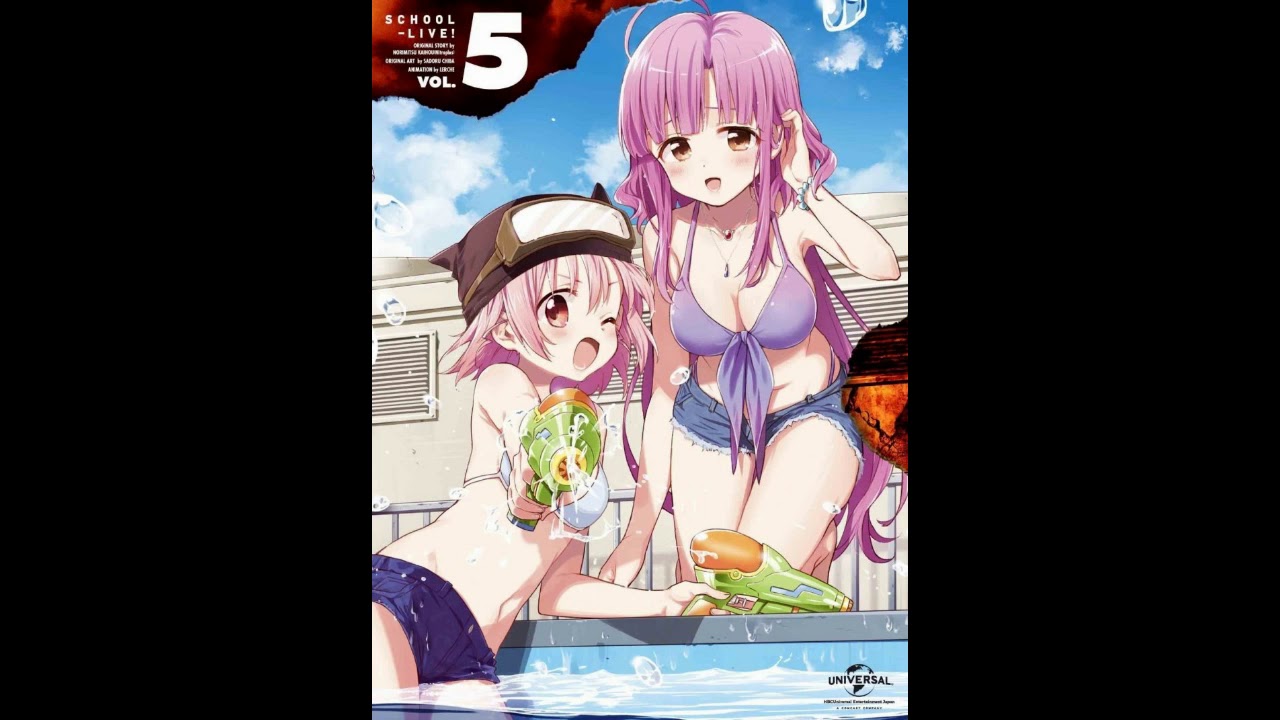
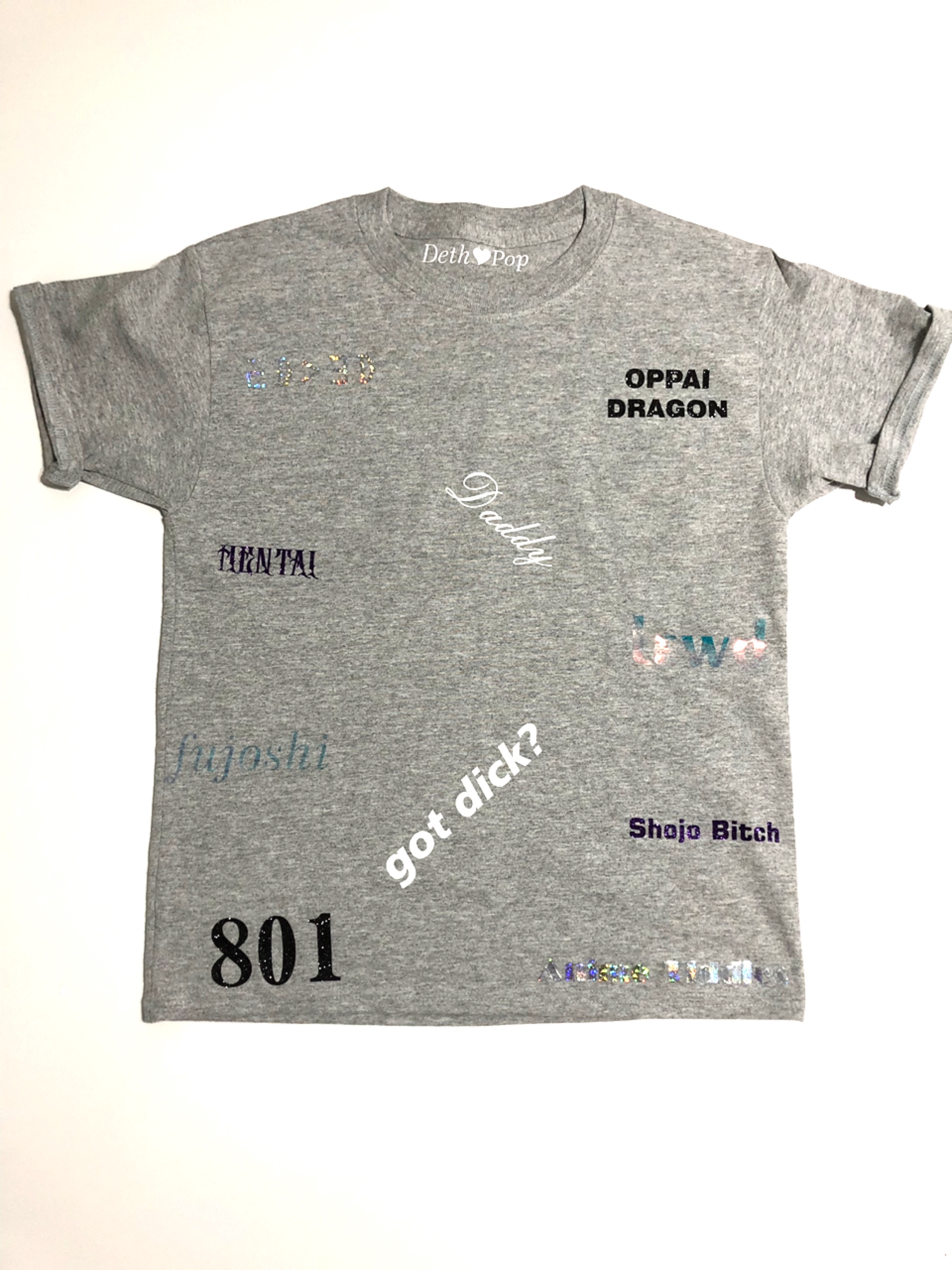
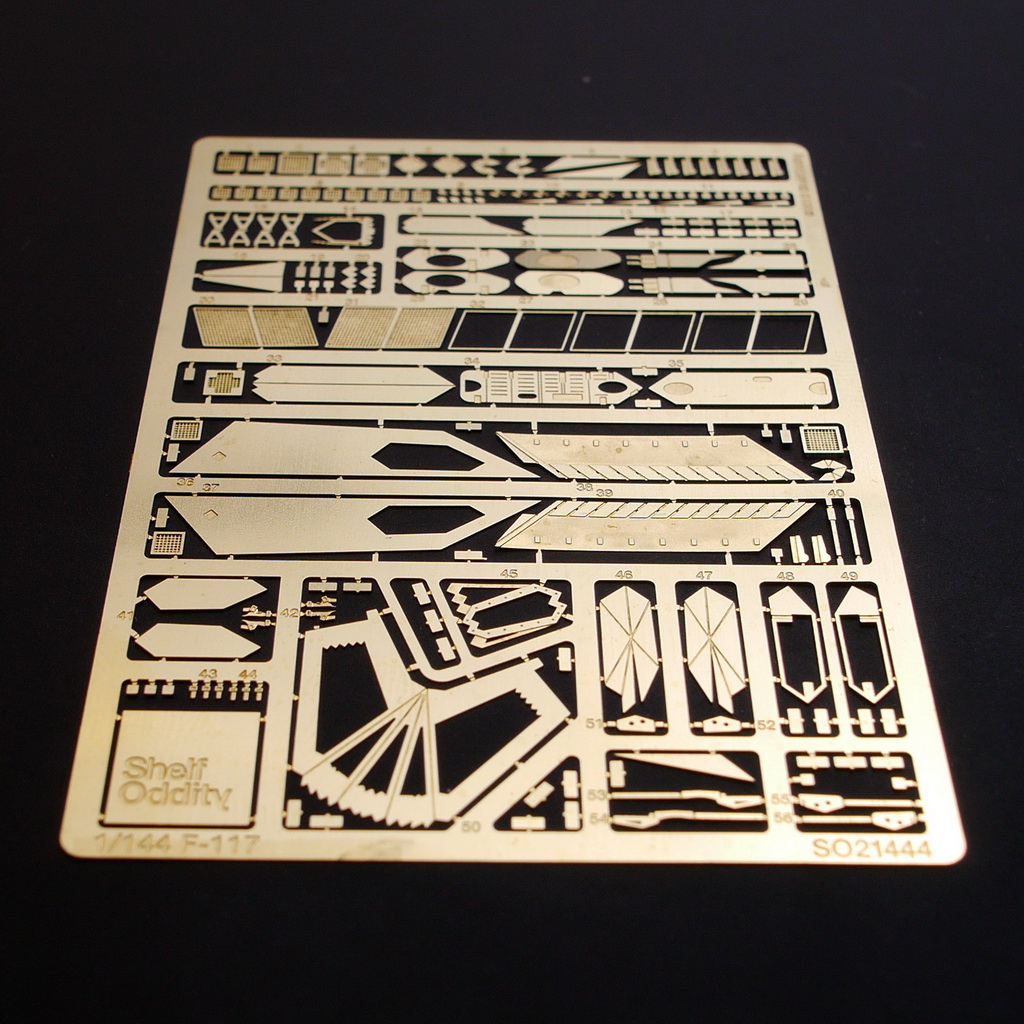
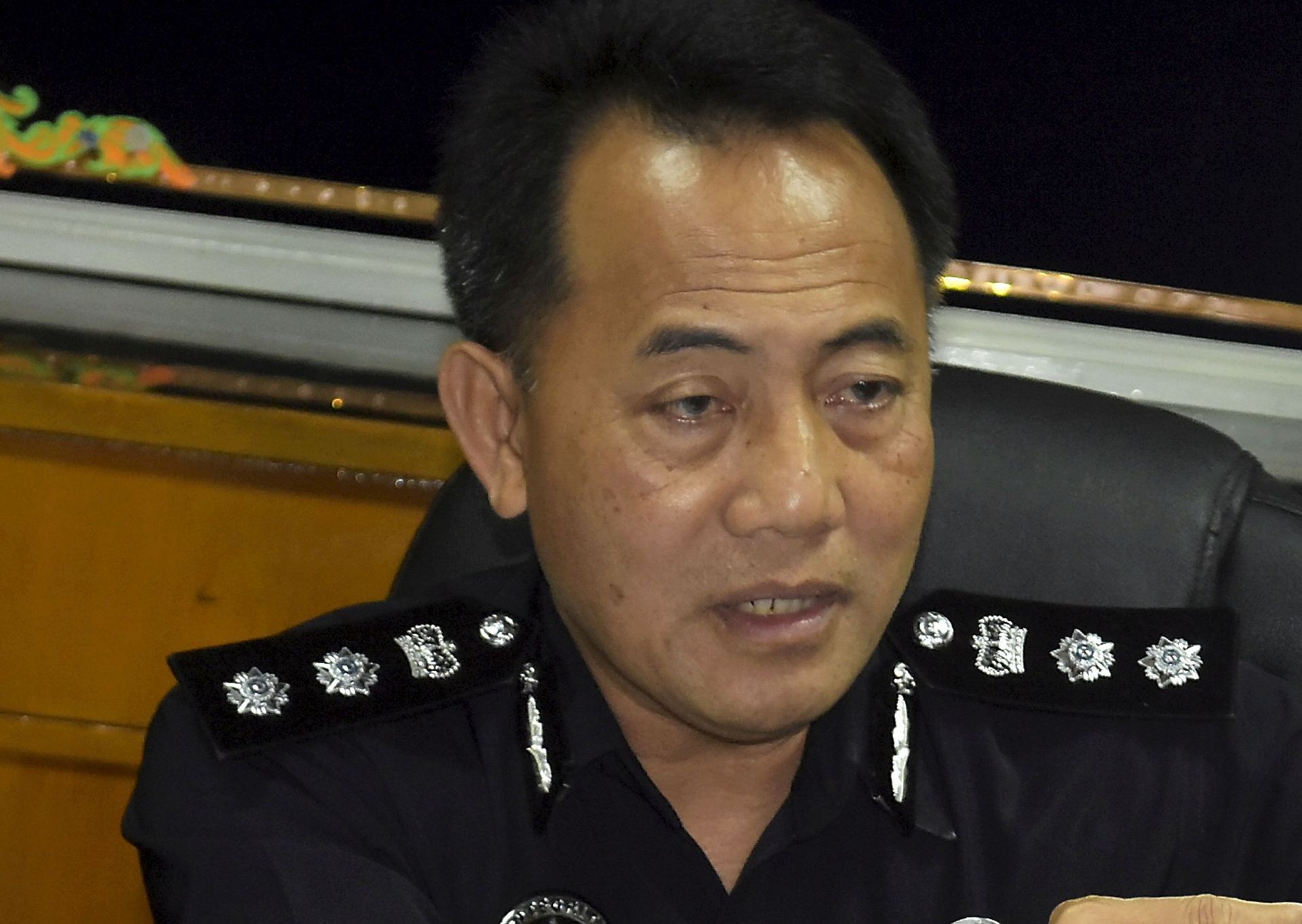
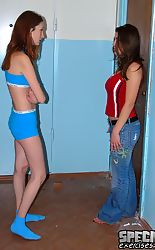





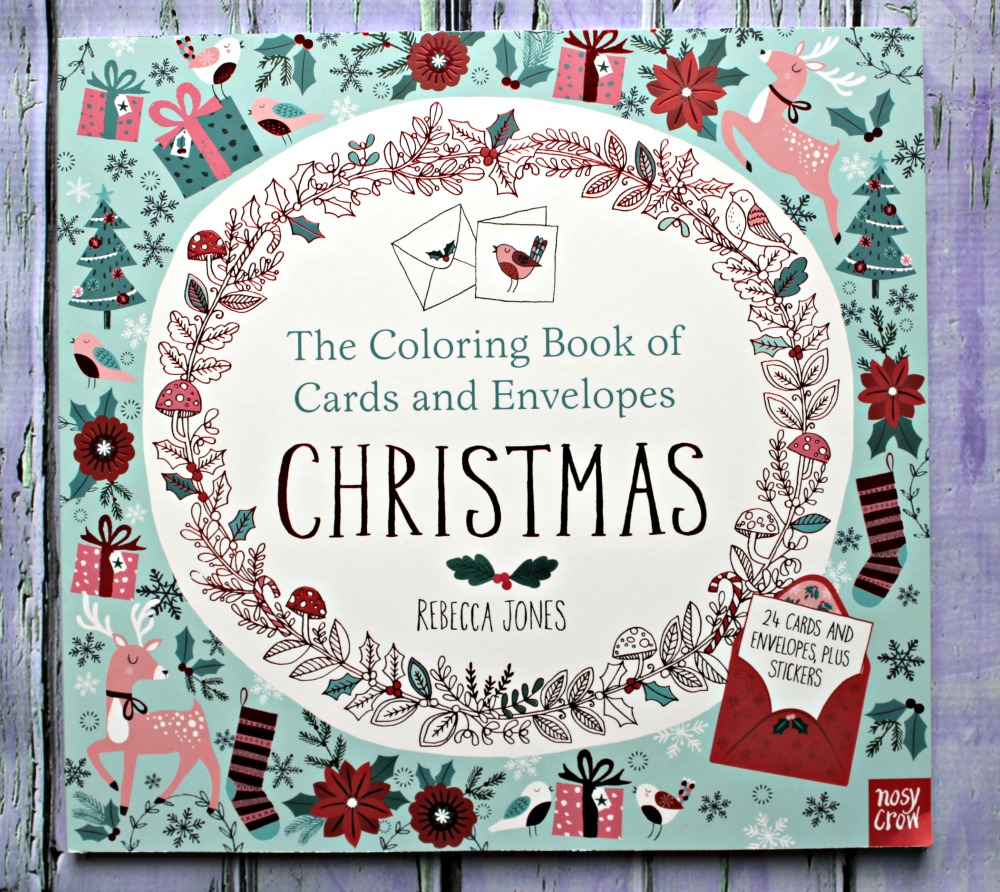
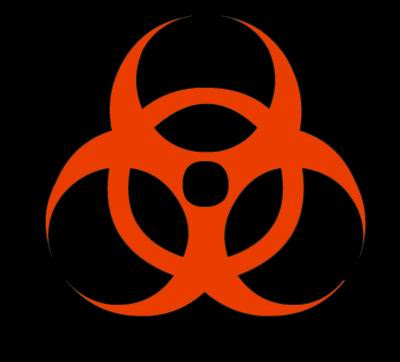
